High Significant Fault Detection in Azure Core Workload Insights
AAAI 2024(2024)
摘要
Azure Core workload insights have time-series data with different metric units. Faults or Anomalies are observed in these time-series data owing to faults observed with respect to metric name, resources region, dimensions, and its dimension value associated with the data. For Azure Core, an important task is to highlight faults or anomalies to the user on a dashboard that they can perceive easily. The number of anomalies reported should be highly significant and in a limited number, e.g., 5-20 anomalies reported per hour. The reported anomalies will have significant user perception and high reconstruction error in any time-series forecasting model. Hence, our task is to automatically identify 'high significant anomalies' and their associated information for user perception.
更多查看译文
关键词
Distributed AI,Machine Learning,Real-Time Systems,Temporal and Geo/Spatial Reasoning,Track: Deployed Applications
AI 理解论文
溯源树
样例
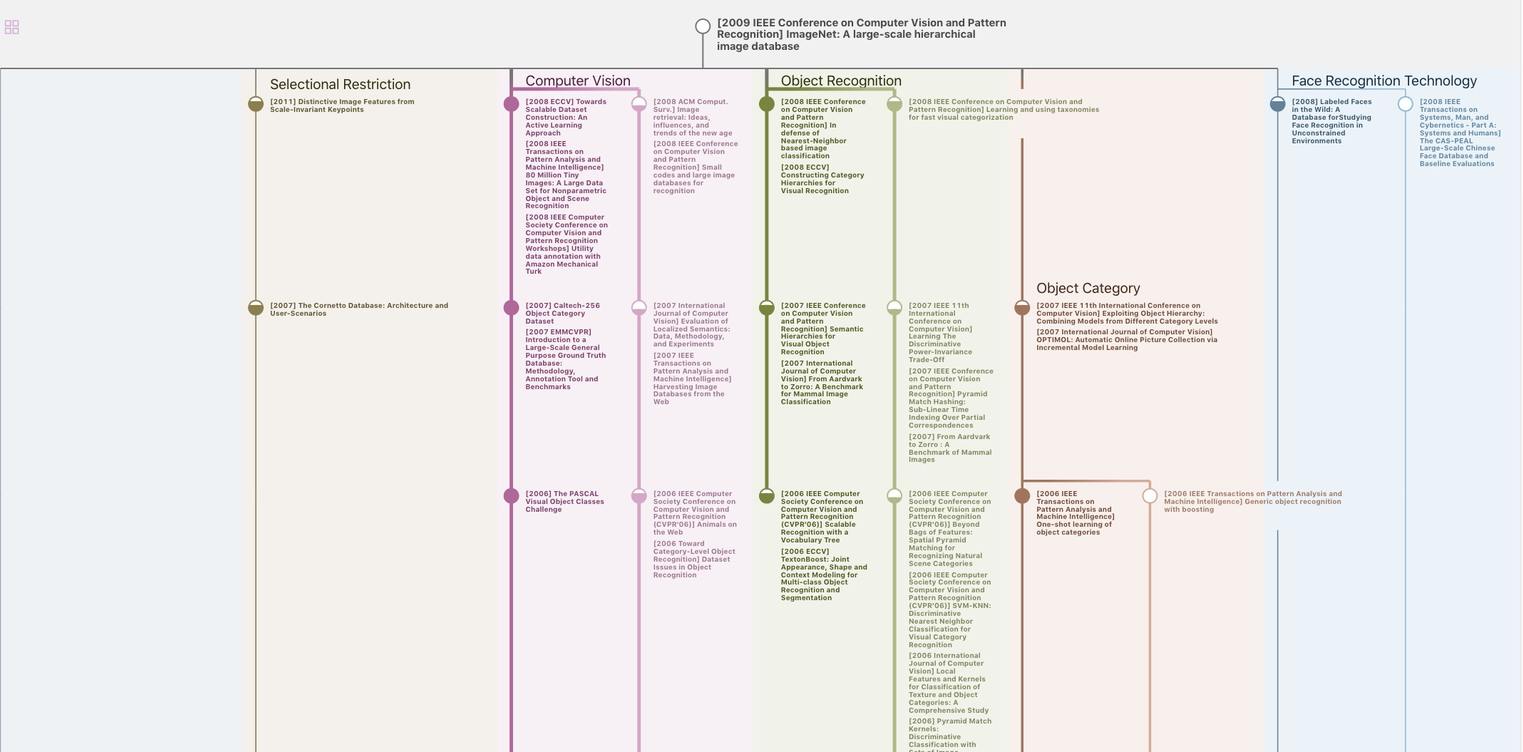
生成溯源树,研究论文发展脉络
Chat Paper
正在生成论文摘要