Towards the Robustness of Differentially Private Federated Learning
AAAI 2024(2024)
摘要
Robustness and privacy protection are two important factors of trustworthy federated learning (FL). Existing FL works usually secure data privacy by perturbing local model gradients via the differential privacy (DP) technique, or defend against poisoning attacks by filtering the local gradients in the outlier of the gradient distribution before aggregation. However, these two issues are often addressed independently in existing works, and how to secure federated learning in both privacy and robustness still needs further exploration. In this paper, we unveil that although DP noisy perturbation can improve the learning robustness, DP-FL frameworks are not inherently robust and are vulnerable to a carefully-designed attack method. Furthermore, we reveal that it is challenging for existing robust FL methods to defend against attacks on DP-FL. This can be attributed to the fact that the local gradients of DP-FL are perturbed by random noise, and the selected central gradients inevitably incorporate a higher proportion of poisoned gradients compared to conventional FL. To address this problem, we further propose a new defense method for DP-FL (named Robust-DPFL), which can effectively distinguish poisoned and clean local gradients in DP-FL and robustly update the global model. Experiments on three benchmark datasets demonstrate that baseline methods cannot ensure task accuracy, data privacy, and robustness simultaneously, while Robust-DPFL can effectively enhance the privacy protection and robustness of federated learning meanwhile maintain the task performance.
更多查看译文
关键词
PEAI: Privacy & Security,ML: Privacy,ML: Adversarial Learning & Robustness
AI 理解论文
溯源树
样例
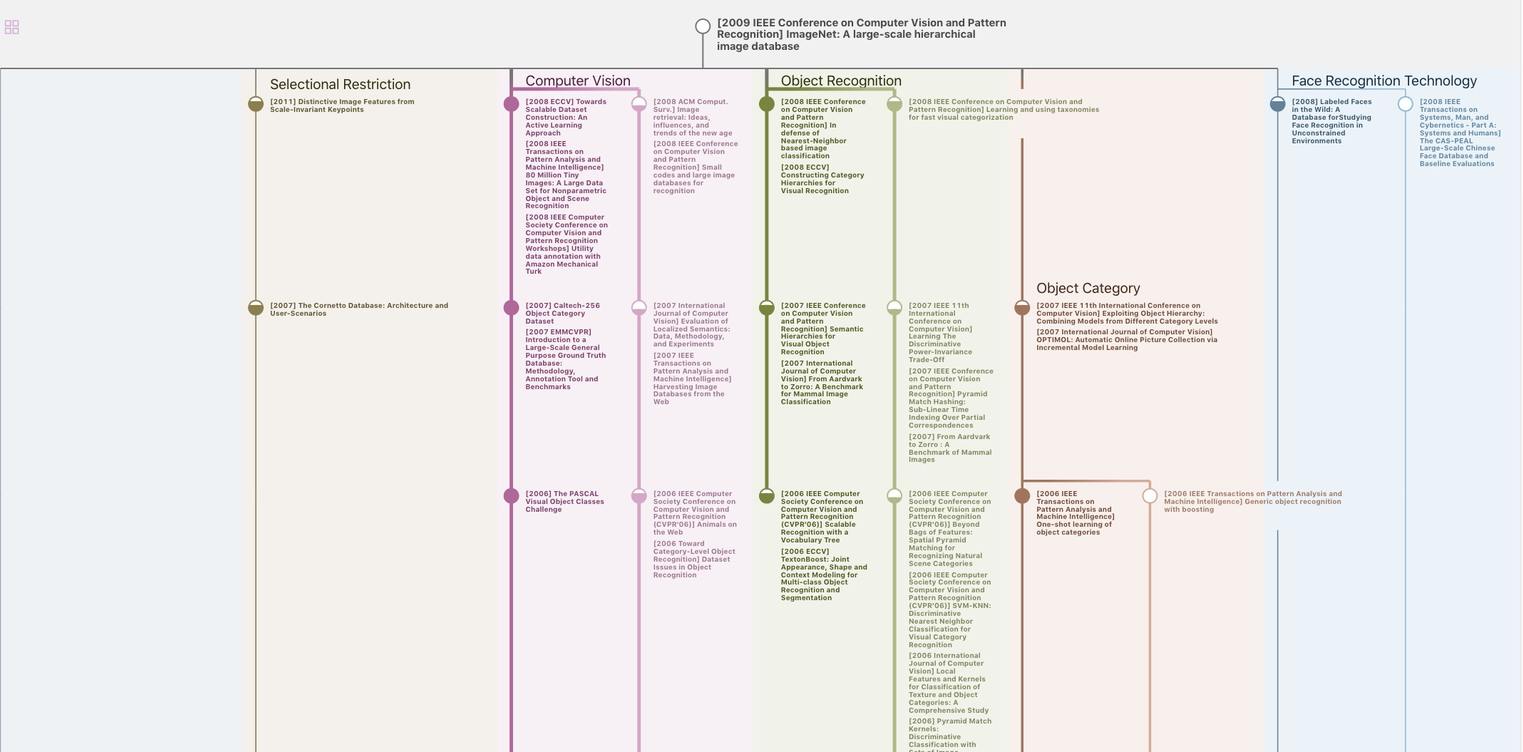
生成溯源树,研究论文发展脉络
Chat Paper
正在生成论文摘要