Propagation Tree Is Not Deep: Adaptive Graph Contrastive Learning Approach for Rumor Detection
AAAI 2024(2024)
摘要
Rumor detection on social media has become increasingly important. Most existing graph-based models presume rumor propagation trees (RPTs) have deep structures and learn sequential stance features along branches. However, through statistical analysis on real-world datasets, we find RPTs exhibit wide structures, with most nodes being shallow 1-level replies. To focus learning on intensive substructures, we propose Rumor Adaptive Graph Contrastive Learning (RAGCL) method with adaptive view augmentation guided by node centralities. We summarize three principles for RPT augmentation: 1) exempt root nodes, 2) retain deep reply nodes, 3) preserve lower-level nodes in deep sections. We employ node dropping, attribute masking and edge dropping with probabilities from centrality-based importance scores to generate views. A graph contrastive objective then learns robust rumor representations. Extensive experiments on four benchmark datasets demonstrate RAGCL outperforms state-of-the-art methods. Our work reveals the wide-structure nature of RPTs and contributes an effective graph contrastive learning approach tailored for rumor detection through principled adaptive augmentation. The proposed principles and augmentation techniques can potentially benefit other applications involving tree-structured graphs.
更多查看译文
关键词
APP: Misinformation & Fake News,NLP: Applications,NLP: Text Classification
AI 理解论文
溯源树
样例
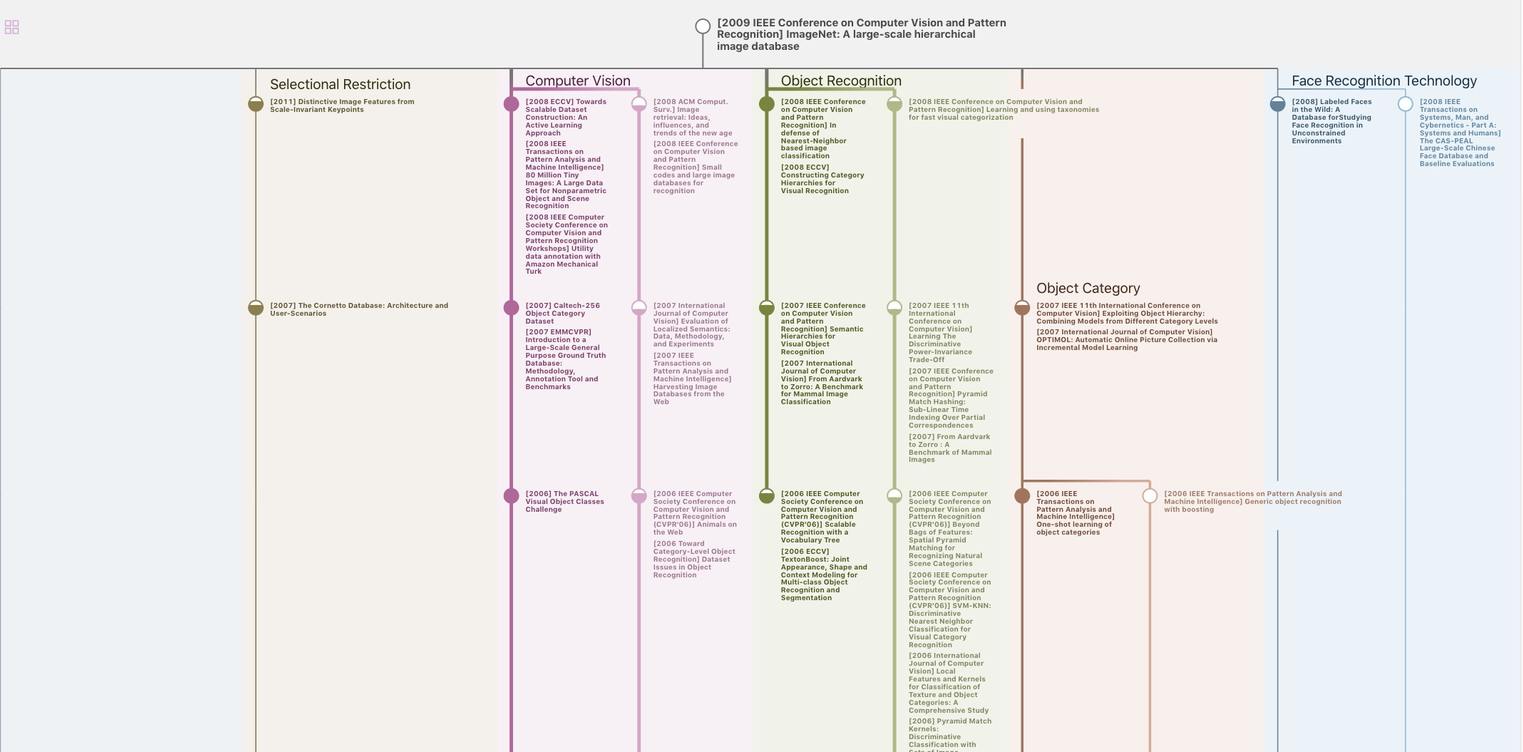
生成溯源树,研究论文发展脉络
Chat Paper
正在生成论文摘要