Understanding Surprising Generalization Phenomena in Deep Learning
AAAI 2024(2024)
摘要
Deep learning has exhibited a number of surprising generalization phenomena that are not captured by classical statistical learning theory. This talk will survey some of my work on the theoretical characterizations of several such intriguing phenomena: (1) Implicit regularization: A major mystery in deep learning is that deep neural networks can often generalize well despite their excessive expressive capacity. Towards explaining this mystery, it has been suggested that commonly used gradient-based optimization algorithms enforce certain implicit regularization which effectively constrains the model capacity. (2) Benign overfitting: In certain scenarios, a model can perfectly fit noisily labeled training data, but still archives near-optimal test error at the same time, which is very different from the classical notion of overfitting. (3) Grokking: In certain scenarios, a model initially achieves perfect training accuracy but no generalization (i.e. no better than a random predictor), and upon further training, transitions to almost perfect generalization. Theoretically establishing these properties often involves making appropriate high-dimensional assumptions on the problem as well as a careful analysis of the training dynamics.
更多查看译文
关键词
Deep Learning,Theory,Generalization,Over-parameterization
AI 理解论文
溯源树
样例
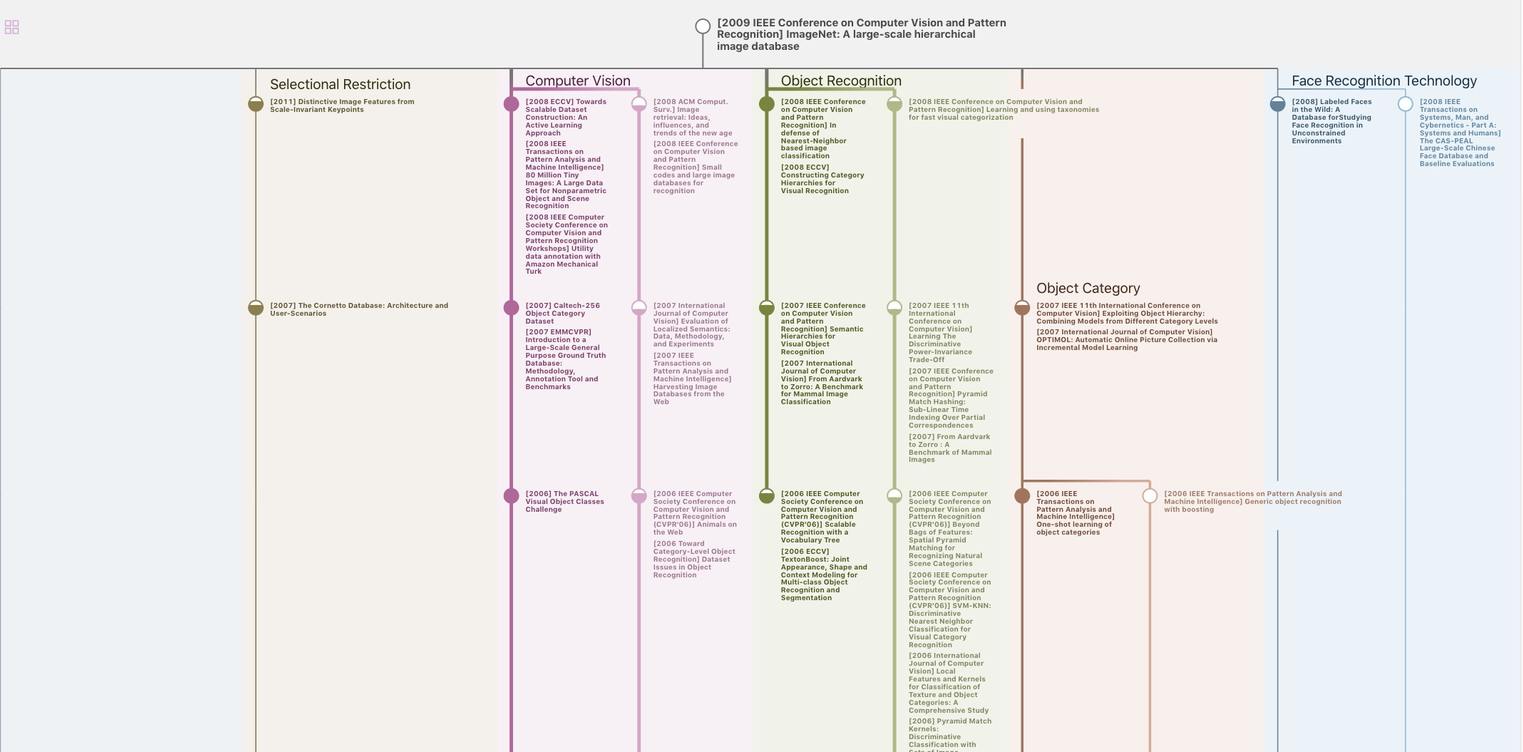
生成溯源树,研究论文发展脉络
Chat Paper
正在生成论文摘要