Learning Image Demoiréing from Unpaired Real Data
AAAI 2024(2024)
摘要
This paper focuses on addressing the issue of image demoiréing. Unlike the large volume of existing studies that rely on learning from paired real data, we attempt to learn a demoiréing model from unpaired real data, i.e., moiré images associated with irrelevant clean images. The proposed method, referred to as Unpaired Demoiréing(UnDeM), synthesizes pseudo moiré images from unpaired datasets, generating pairs with clean images for training demoiréing models. To achieve this, we divide real moiré images into patches and group them in compliance with their moiré complexity. We introduce a novel moiré generation framework to synthesize moiré images with diverse moiré features, resembling real moiré patches, and details akin to real moiré-free images. Additionally, we introduce an adaptive denoise method to eliminate the low-quality pseudo moiré images that adversely impact the learning of demoiréing models. We conduct extensive experiments on the commonly-used FHDMi and UHDM datasets. Results manifest that our UnDeM performs better than existing methods when using existing demoiréing models such as MBCNN and ESDNet-L. Code: https://github.com/zysxmu/UnDeM.
更多查看译文
关键词
CV: Applications
AI 理解论文
溯源树
样例
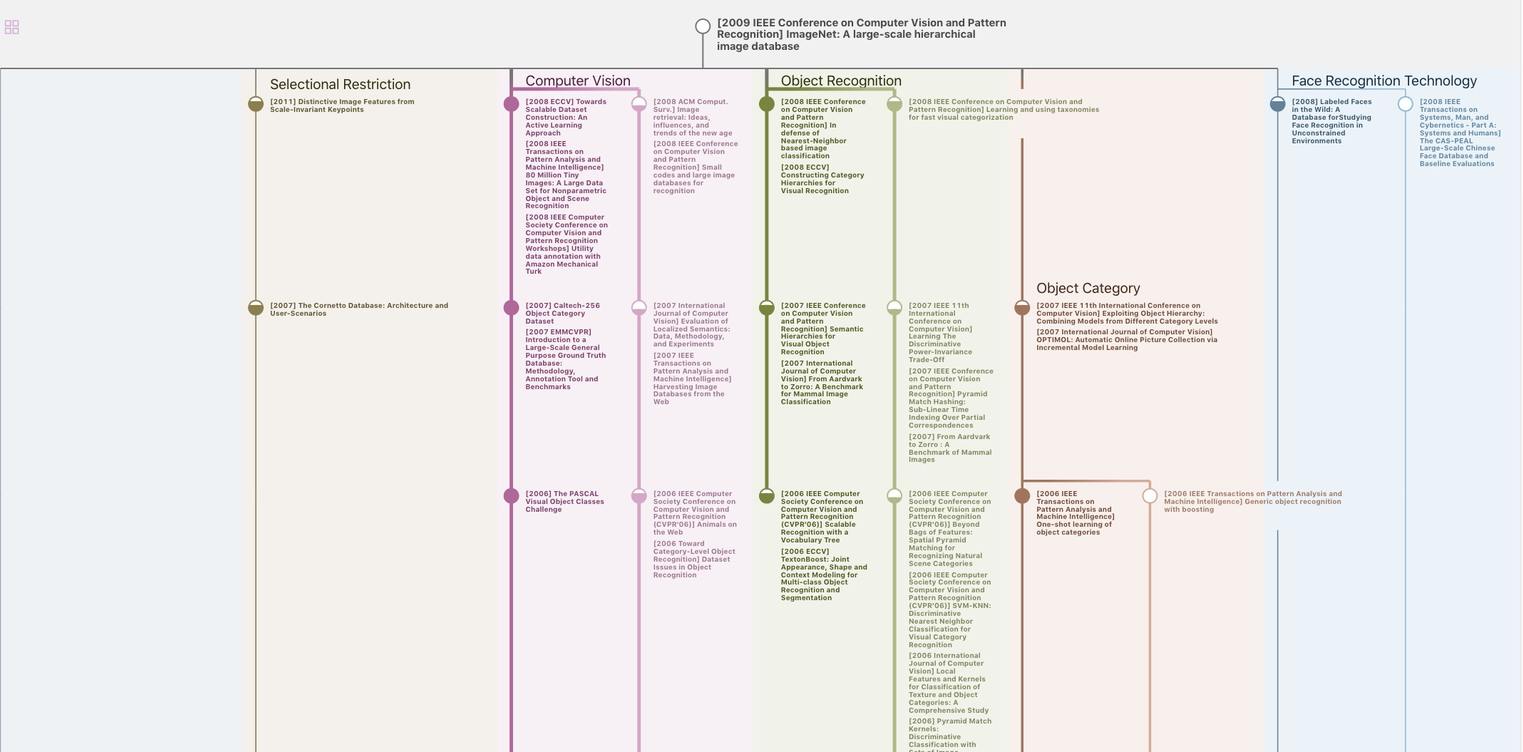
生成溯源树,研究论文发展脉络
Chat Paper
正在生成论文摘要