A Comparative Study of Clustering Methods for Nanoindentation Mapping Data
Integrating Materials and Manufacturing Innovation(2024)
摘要
Nanoindentation testing and instrumented indentation remain regularly utilized techniques for the assessment of multi-scale mechanical characteristics from load–displacement data analysis, which is central to twenty first century material characterization. The advent of high-resolution nanoindentation-based property mapping has, however, presented challenges in data interpretation, especially when applying proper clustering methodologies to quantify and interpret data as well as draw appropriate conclusions. In this research, we utilized the scikit-learn library in Python to assess the performance of various clustering algorithms, with a focus on nanoindentation-based hardness and elastic modulus measurements, and their synergistic effects. Clustering parameters were meticulously optimized, and in conjunction with domain expert recommendations, the total number of clusters was set to three. The evaluation was grounded in established clustering performance metrics such as the Davies–Bouldin Index, Calinski–Harabasz Index, and the Silhouette score, aiming to ascertain the optimal clustering approach. Among the eight evaluated clustering algorithms, K-means, Agglomerative and FCM emerged as the most effective, while the OPTICS algorithm consistently underperformed for the considered datasets. Augmenting this study, we introduce an intuitive interface, negating the necessity for prior coding or machine learning familiarity, and offering effortless model fine-tuning, visualization, and comparison. This innovation empowers material science and engineering experts, technical staff, and instrumentalists and facilitates the selection of ideal models across varied datasets. The insights and tools presented herein not only enrich material science and engineering research but also lay a robust foundation for sophisticated and dependable analyses in subsequent studies.
更多查看译文
关键词
Nanoindentation,Clustering methods,Evaluation scores,Ranking aggregation method,Voting system
AI 理解论文
溯源树
样例
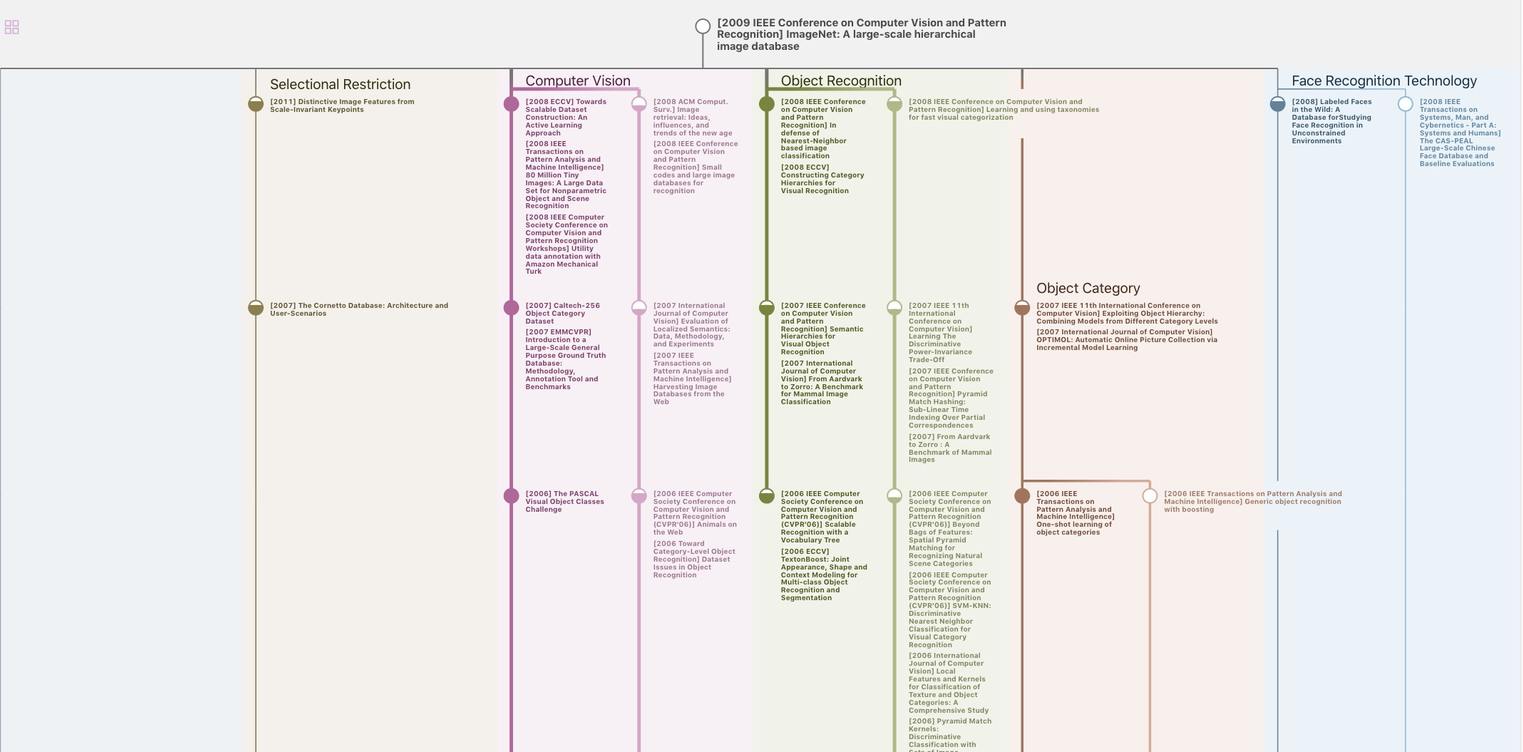
生成溯源树,研究论文发展脉络
Chat Paper
正在生成论文摘要