Quad Bayer Joint Demosaicing and Denoising Based on Dual Encoder Network with Joint Residual Learning
AAAI 2024(2024)
摘要
The recent imaging technology Quad Bayer CFA brings better imaging PSNR and higher visual quality compared to traditional Bayer CFA, but also serious challenges for demosaicing and denoising during the ISP pipeline. In this paper, we propose a novel dual encoder network, namely DRNet, to achieve joint demosaicing and denoising for Quad Bayer CFA. The dual encoders are carefully designed in that one is mainly constructed by a joint residual block to jointly estimate the residuals for demosaicing and denoising separately. In contrast, the other one is started with a pixel modulation block which is specially designed to match the characteristics of Quad Bayer pattern for better feature extraction. We demonstrate the effectiveness of each proposed component through detailed ablation investigations. The comparison results on public benchmarks illustrate that our DRNet achieves an apparent performance gain~(0.38dB to the 2nd best) from the state-of-the-art method and balances performance and efficiency well. The experiments on real-world images show that the proposed method could enhance the reconstruction quality from the native ISP algorithm.
更多查看译文
关键词
CV: Computational Photography, Image & Video Synthesis,CV: Applications,CV: Low Level & Physics-based Vision
AI 理解论文
溯源树
样例
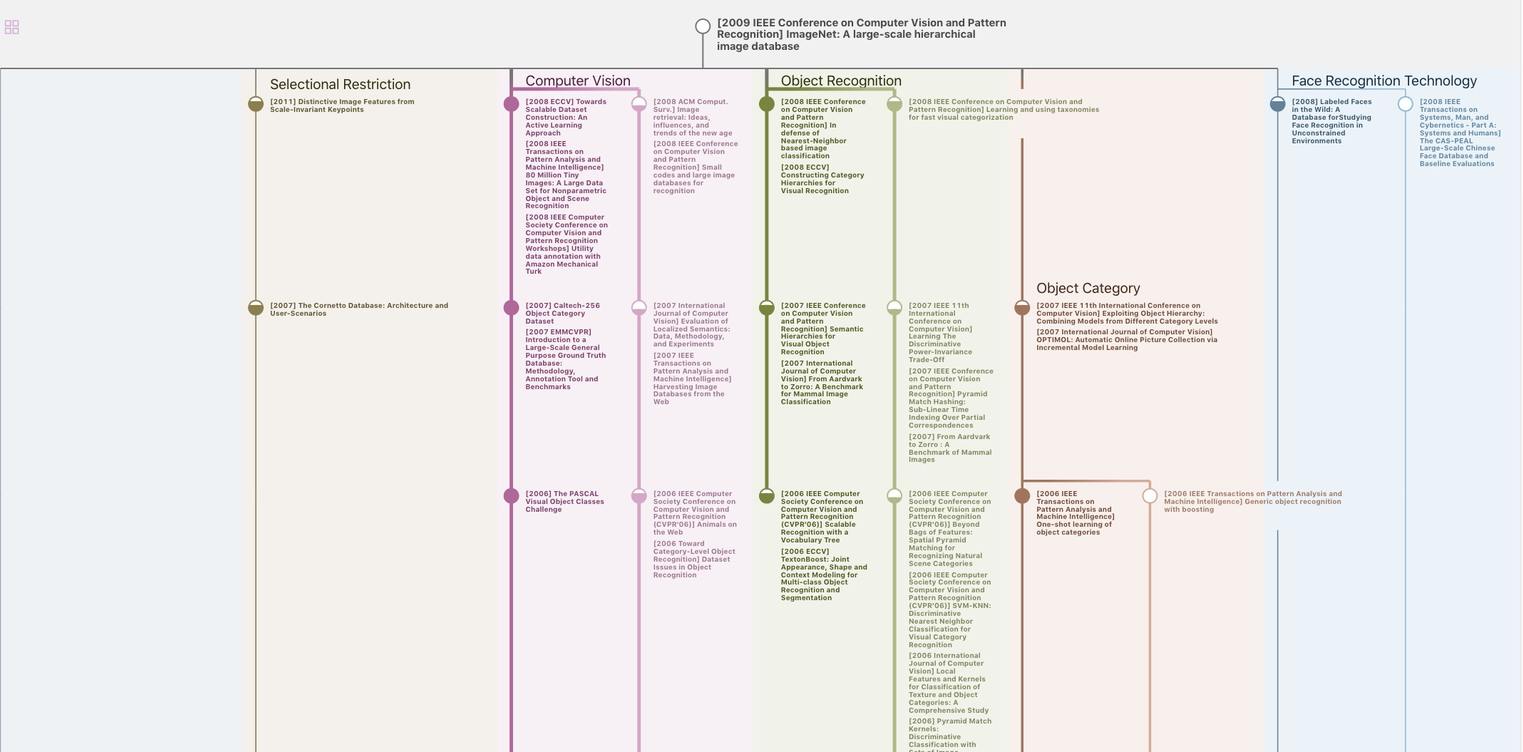
生成溯源树,研究论文发展脉络
Chat Paper
正在生成论文摘要