Unsupervised Gene-Cell Collective Representation Learning with Optimal Transport
AAAI 2024(2024)
摘要
Cell type identification plays a vital role in single-cell RNA sequencing (scRNA-seq) data analysis. Although many deep embedded methods to cluster scRNA-seq data have been proposed, they still fail in elucidating the intrinsic properties of cells and genes. Here, we present a novel end-to-end deep graph clustering model for single-cell transcriptomics data based on unsupervised Gene-Cell Collective representation learning and Optimal Transport (scGCOT) which integrates both cell and gene correlations. Specifically, scGCOT learns the latent embedding of cells and genes simultaneously and reconstructs the cell graph, the gene graph, and the gene expression count matrix. A zero-inflated negative binomial (ZINB) model is estimated via the reconstructed count matrix to capture the essential properties of scRNA-seq data. By leveraging the optimal transport-based joint representation alignment, scGCOT learns the clustering process and the latent representations through a mutually supervised self optimization strategy. Extensive experiments with 14 competing methods on 15 real scRNA-seq datasets demonstrate the competitive edges of scGCOT.
更多查看译文
关键词
APP: Other Applications
AI 理解论文
溯源树
样例
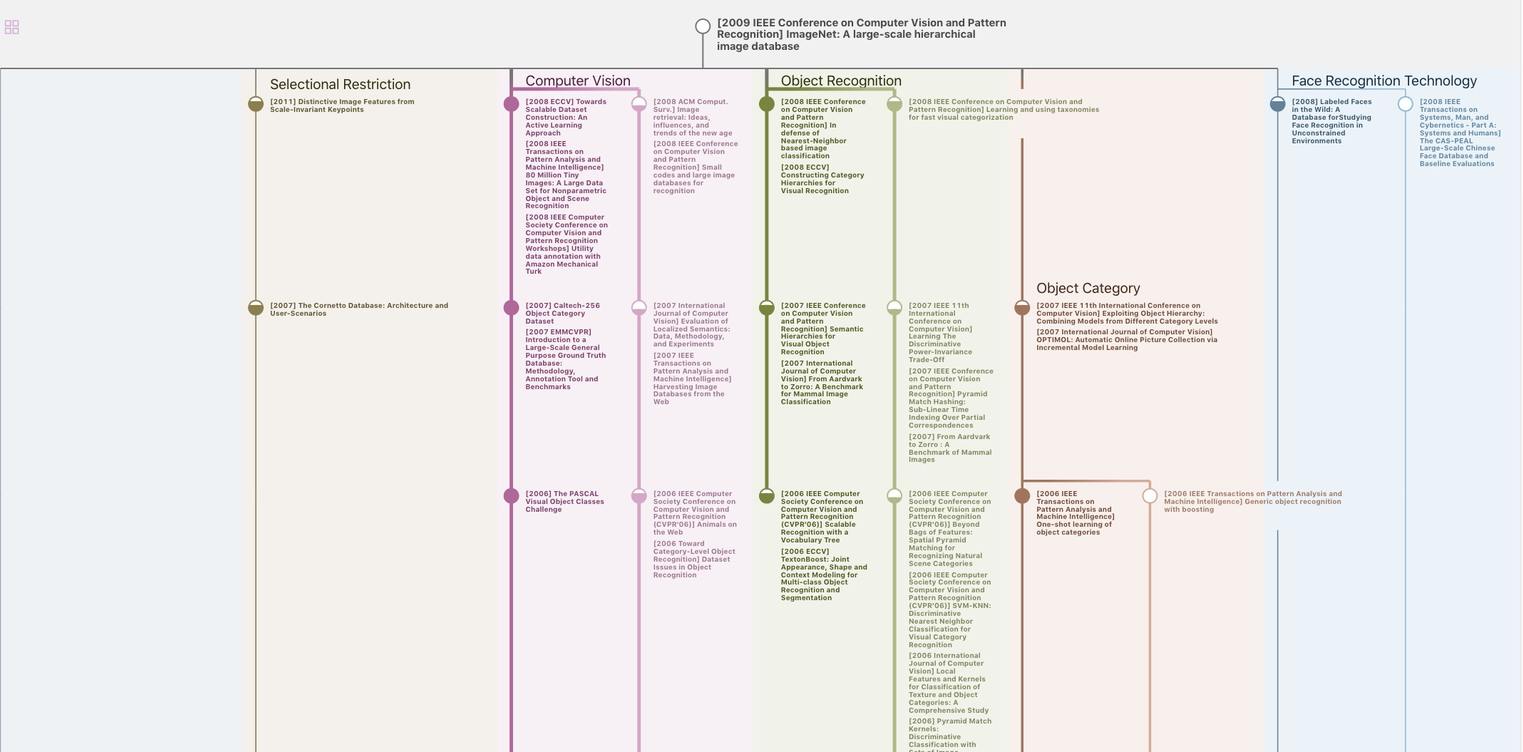
生成溯源树,研究论文发展脉络
Chat Paper
正在生成论文摘要