Photovoltaic Power Prediction Considering VMD-CNN-LSTM and Migration Learning Frameworks for Poor Data Areas
2024 IEEE 4th International Conference on Power, Electronics and Computer Applications (ICPECA)(2024)
摘要
PV power prediction based on historical data and deep learning has been widely used in power systems to improve prediction accuracy. However, it is difficult to establish an accurate PV power prediction model for newly constructed PV power plants due to the lack of historical data. Therefore, based on the migration learning framework, this paper proposes a long and short-term memory network (CNN-LSTM) with the fusion of VMD-CNN-LSTM based on the variable mode decomposition (VMD). Therefore, based on the transfer learning framework, this paper proposes a VMD-CNN-LSTM method for PV power prediction based on the convergence of variational mode decomposition (VMD) and convolutional neural network short term memory (CNN-LSTM). Firstly, VMD is used to decompose the preprocessed historical PV power and meteorological data to reduce its complexity and random volatility; secondly, the decomposed sequences are predicted using a combined CNN-LSTM model, and the prediction results of each component are integrated; finally, Transfer Learning (TL) is introduced to fine-tune the constructed CNN-LSTM model. Through the way of model parameter sharing, the pre-trained model is extrapolated to the data-scarce target PV power plant to realize the migration learning of data features under the condition of few samples. The method is used to conduct experiments on PV power plants in data-poor areas, and the results show that the algorithm proposed in this paper can achieve higher accuracy compared with other prediction models.
更多查看译文
关键词
Transfer Learning,Photovoltaic power generation,Power forecasting,Small Samples
AI 理解论文
溯源树
样例
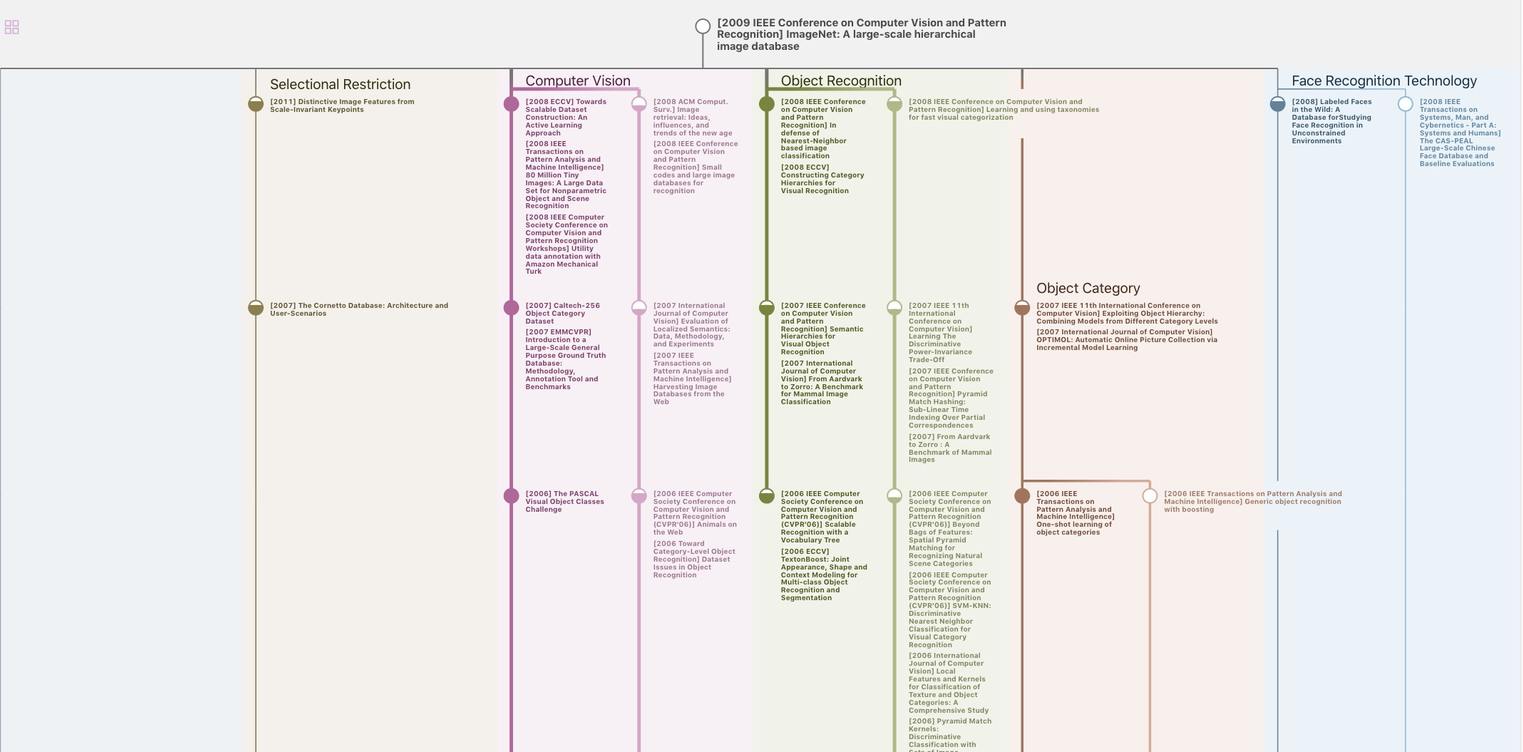
生成溯源树,研究论文发展脉络
Chat Paper
正在生成论文摘要