Reliable Data Generation and Selection for Low-Resource Relation Extraction
AAAI 2024(2024)
摘要
Automated construction of annotated data holds significant importance in Relation Extraction (RE) tasks due to the hardness and cost of human annotation. In this work, we propose Self-RDGS, a method for Self-supervised Reliable Data Generation and Selection in low-resource RE tasks. At first, we fully utilize the knowledge of triplets as prompts to generate sentences by employing the Large Language Models (LLMs). Since the auto-generated data contains noise, we then propose a ranking-based data selection method to select reliable sentences. Finally, we integrate the data selection and RE model training within a self-supervised iterative framework. Through experimentation on three datasets with low-resource settings, we demonstrate the effectiveness of our proposed approach in constructing annotated data and achieving noteworthy improvements in comparison to multiple baselines. Code, data and models are available at https://github.com/jjyunlp/GenerationRE.
更多查看译文
关键词
NLP: Information Extraction,NLP: Generation
AI 理解论文
溯源树
样例
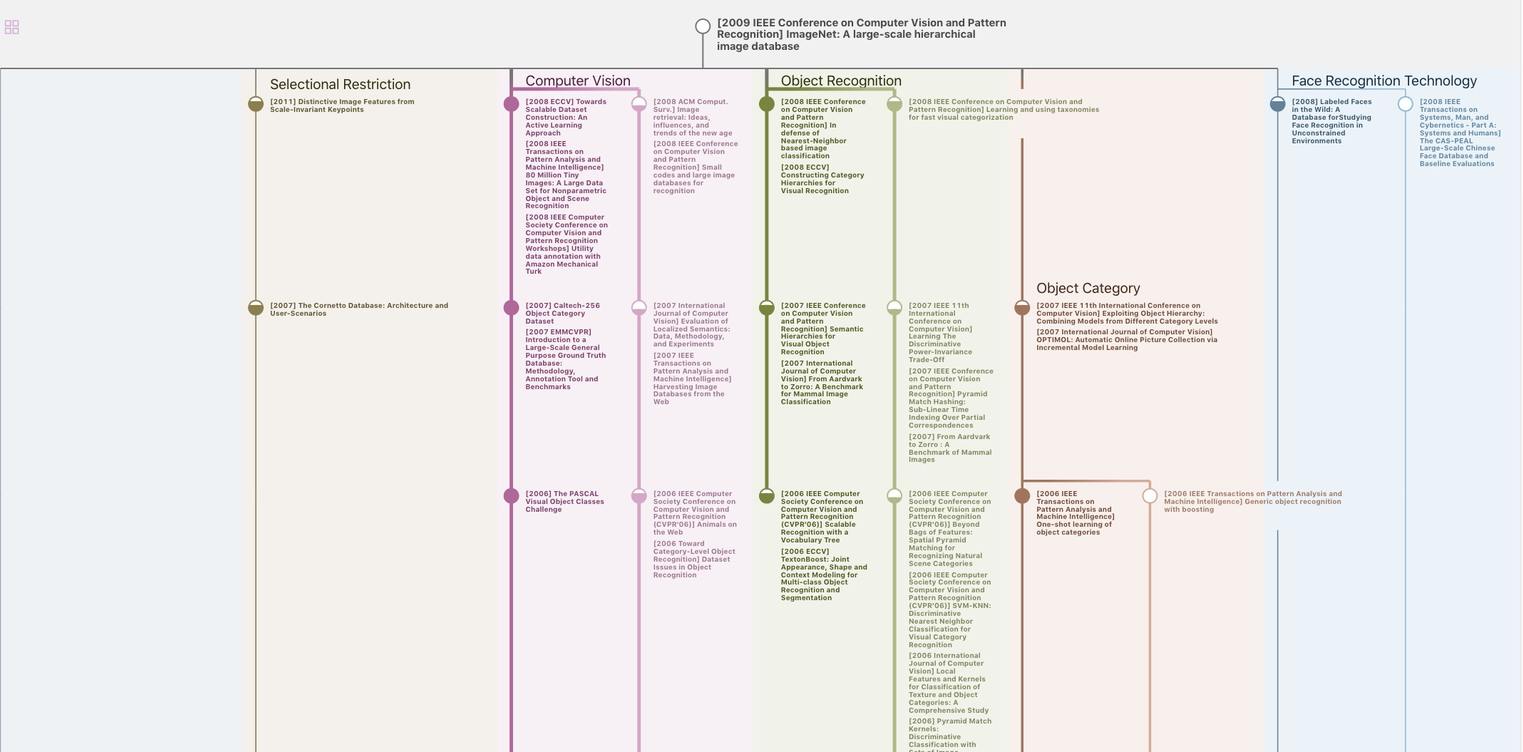
生成溯源树,研究论文发展脉络
Chat Paper
正在生成论文摘要