SAVSR: Arbitrary-Scale Video Super-Resolution via a Learned Scale-Adaptive Network
AAAI 2024(2024)
摘要
Deep learning-based video super-resolution (VSR) networks have gained significant performance improvements in recent years. However, existing VSR networks can only support a fixed integer scale super-resolution task, and when we want to perform VSR at multiple scales, we need to train several models. This implementation certainly increases the consumption of computational and storage resources, which limits the application scenarios of VSR techniques. In this paper, we propose a novel Scale-adaptive Arbitrary-scale Video Super-Resolution network (SAVSR), which is the first work focusing on spatial VSR at arbitrary scales including both non-integer and asymmetric scales. We also present an omni-dimensional scale-attention convolution, which dynamically adapts according to the scale of the input to extract inter-frame features with stronger representational power. Moreover, the proposed spatio-temporal adaptive arbitrary-scale upsampling performs VSR tasks using both temporal features and scale information. And we design an iterative bi-directional architecture for implicit feature alignment. Experiments at various scales on the benchmark datasets show that the proposed SAVSR outperforms state-of-the-art (SOTA) methods at non-integer and asymmetric scales. The source code is available at https://github.com/Weepingchestnut/SAVSR.
更多查看译文
关键词
CV: Low Level & Physics-based Vision,CV: Applications,CV: Computational Photography, Image & Video Synthesis
AI 理解论文
溯源树
样例
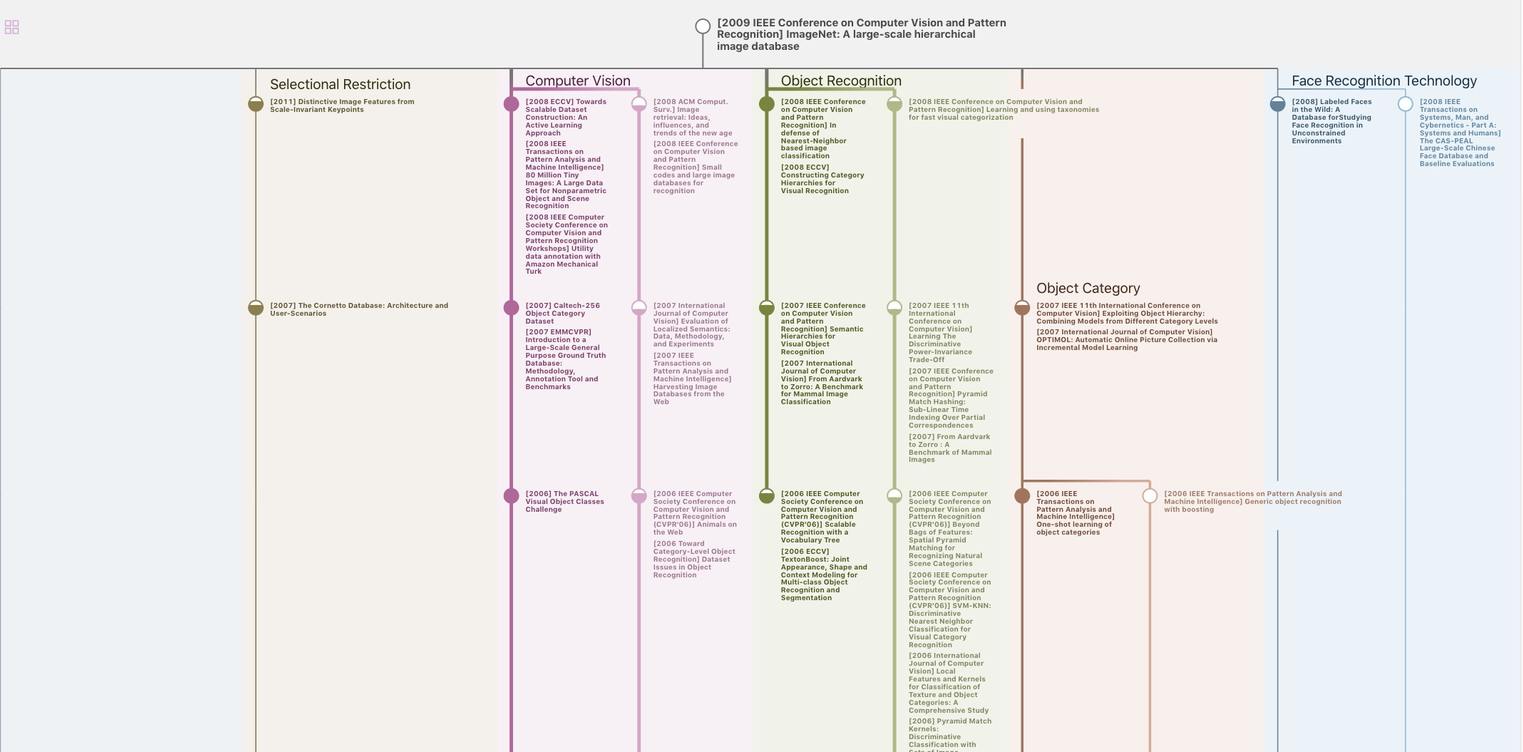
生成溯源树,研究论文发展脉络
Chat Paper
正在生成论文摘要