The Evidence Contraction Issue in Deep Evidential Regression: Discussion and Solution
AAAI 2024(2024)
摘要
Deep Evidential Regression (DER) places a prior on the original Gaussian likelihood and treats learning as an evidence acquisition process to quantify uncertainty. For the validity of the evidence theory, DER requires specialized activation functions to ensure that the prior parameters remain non-negative. However, such constraints will trigger evidence contraction, causing sub-optimal performance. In this paper, we analyse DER theoretically, revealing the intrinsic limitations for sub-optimal performance: the non-negativity constraints on the Normal Inverse-Gamma (NIG) prior parameter trigger the evidence contraction under the specialized activation function, which hinders the optimization of DER performance. On this basis, we design a Non-saturating Uncertainty Regularization term, which effectively ensures that the performance is further optimized in the right direction. Experiments on real-world datasets show that our proposed approach improves the performance of DER while maintaining the ability to quantify uncertainty.
更多查看译文
关键词
General
AI 理解论文
溯源树
样例
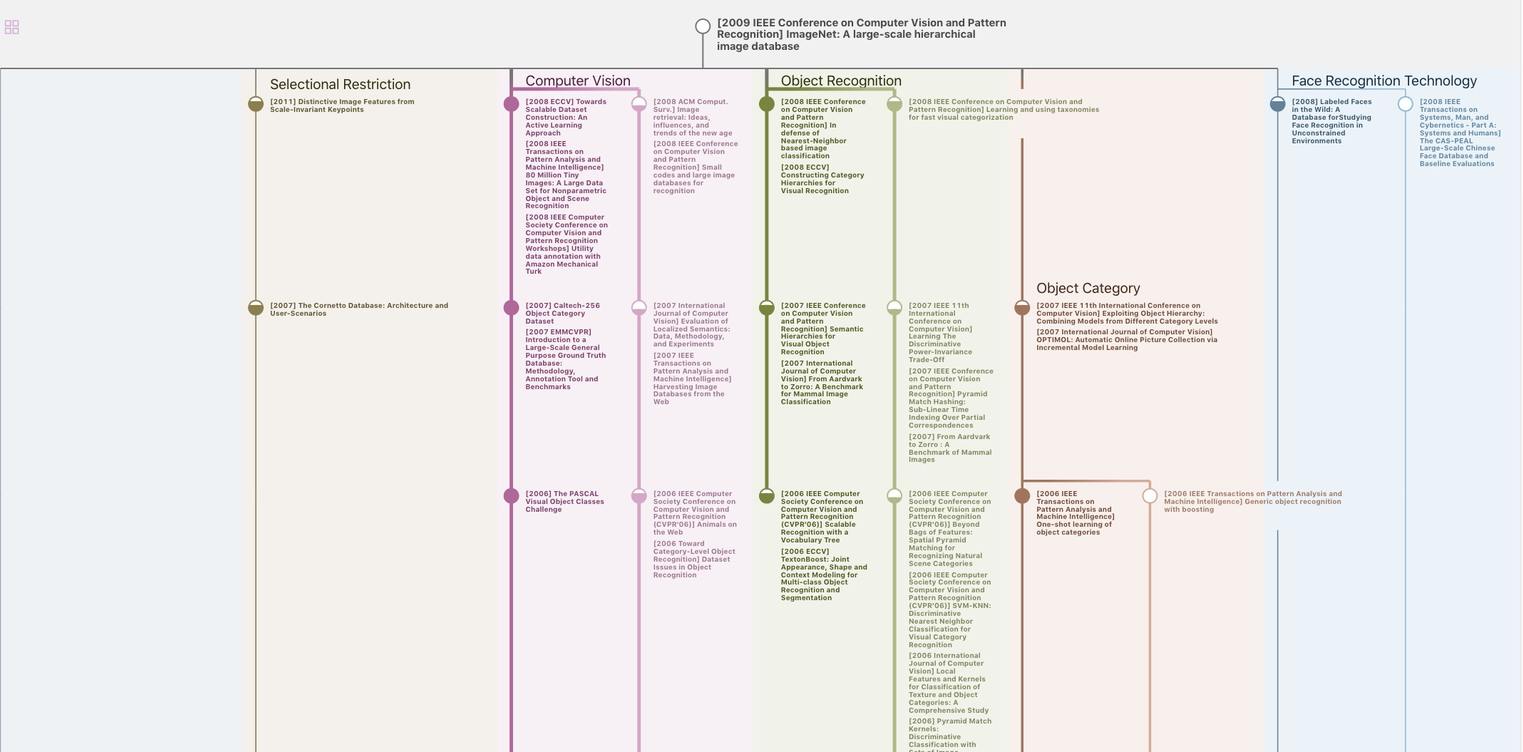
生成溯源树,研究论文发展脉络
Chat Paper
正在生成论文摘要