Multiagent Gumbel MuZero: Efficient Planning in Combinatorial Action Spaces
AAAI 2024(2024)
摘要
AlphaZero and MuZero have achieved state-of-the-art (SOTA) performance in a wide range of domains, including board games and robotics, with discrete and continuous action spaces. However, to obtain an improved policy, they often require an excessively large number of simulations, especially for domains with large action spaces. As the simulation budget decreases, their performance drops significantly. In addition, many important real-world applications have combinatorial (or exponential) action spaces, making it infeasible to search directly over all possible actions. In this paper, we extend AlphaZero and MuZero to learn and plan in more complex multiagent (MA) Markov decision processes, where the action spaces increase exponentially with the number of agents. Our new algorithms, MA Gumbel AlphaZero and MA Gumbel MuZero, respectively without and with model learning, achieve superior performance on cooperative multiagent control problems, while reducing the number of environmental interactions by up to an order of magnitude compared to model-free approaches. In particular, we significantly improve prior performance when planning with much fewer simulation budgets. The code and appendix are available at https://github.com/tjuHaoXiaotian/MA-MuZero.
更多查看译文
关键词
ML: Reinforcement Learning,MAS: Coordination and Collaboration,SO: Sampling/Simulation-based Search
AI 理解论文
溯源树
样例
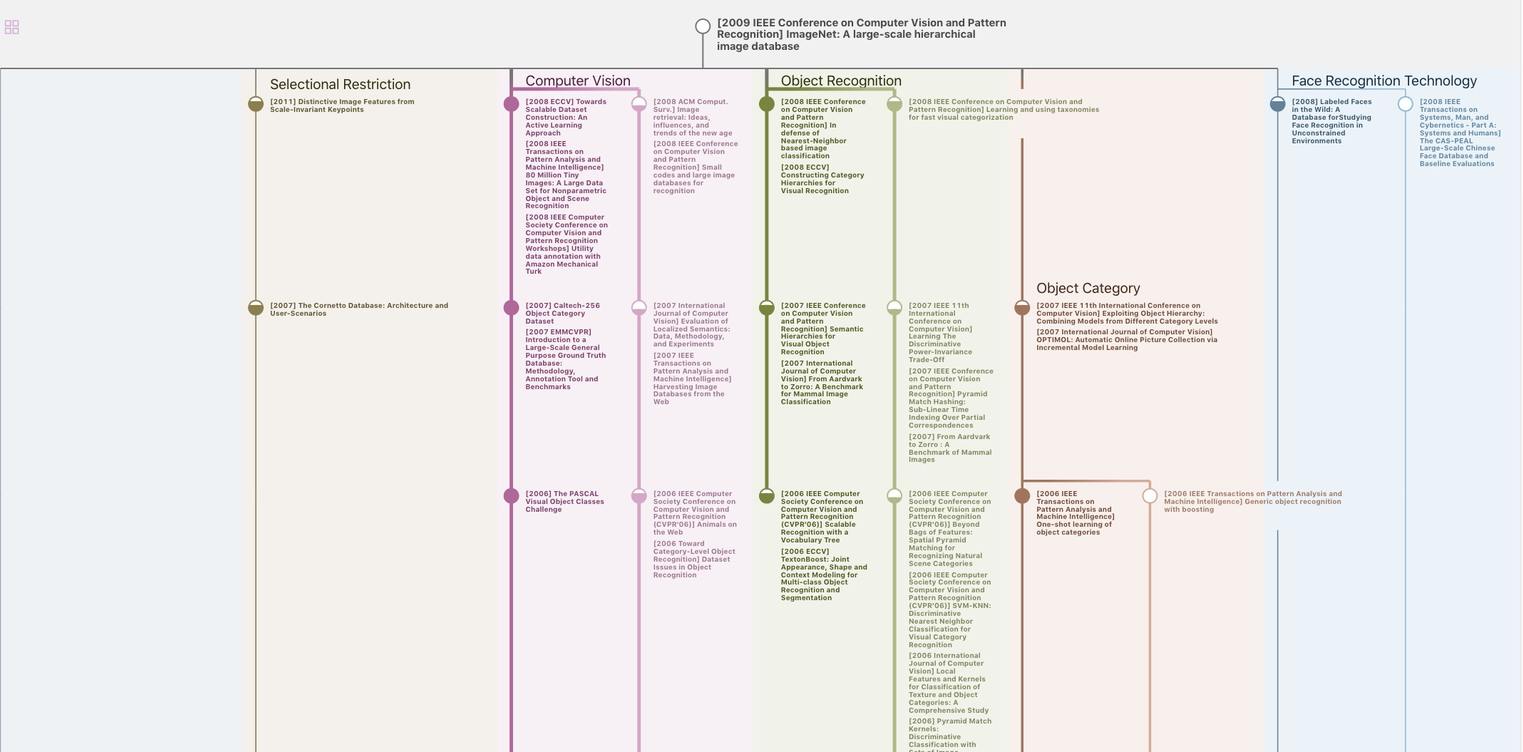
生成溯源树,研究论文发展脉络
Chat Paper
正在生成论文摘要