Towards Human-like Learning from Relational Structured Data
AAAI 2024(2024)
摘要
Relational structured data is a way of representing knowledge using nodes and edges, while also capturing the meaning of that knowledge in a structured form that can be used for machine learning. Compared with vision and natural language data, relational structured data represents and manipulates structured knowledge, which can be beneficial for tasks that involve reasoning or inference. On the other hand, vision and NLP deal more with unstructured data (like images and text), and they often require different types of models and algorithms to extract useful information or features from the data. Human-like Learning develops methods that can harness relational structures and learning-to-learn to rapidly acquire and generalize knowledge to new tasks and situations. With Human-like Learning, the learning algorithm is efficient and can adapt to new or unseen situations, which is crucial in real-world applications where environments may change unpredictably. Moreover, the models are easier for humans to understand and interpret, which is important for transparency and trust in AI systems. In this talk, we present our recent attempts towards human-like learning from relational structured data.
更多查看译文
关键词
Graph Neural Networks,Automated Machine Learning,Neural Architecture Search,Few-shot Learning,Knowledge Graph,Biomedical Network
AI 理解论文
溯源树
样例
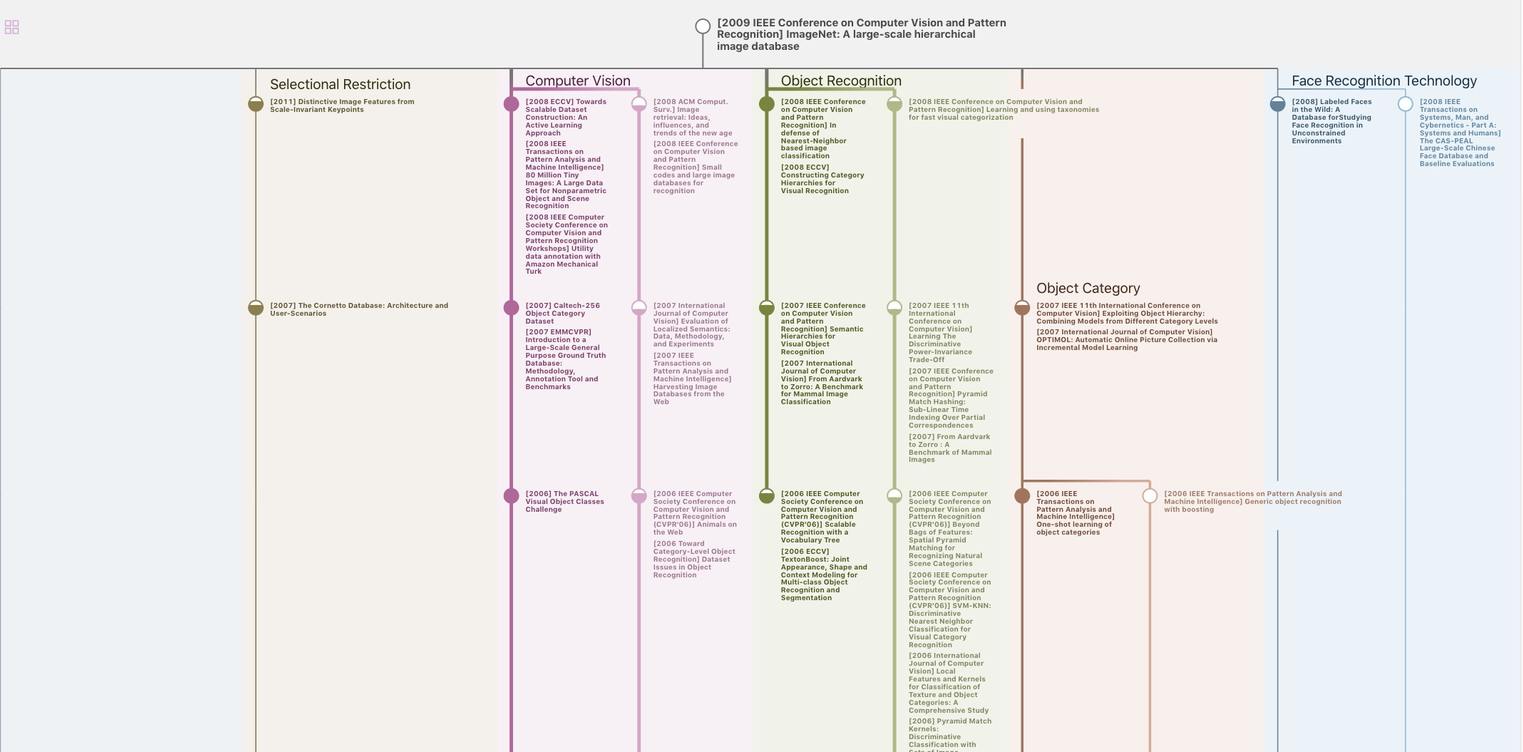
生成溯源树,研究论文发展脉络
Chat Paper
正在生成论文摘要