F³-Pruning: A Training-Free and Generalized Pruning Strategy towards Faster and Finer Text-to-Video Synthesis
AAAI 2024(2024)
摘要
Recently Text-to-Video (T2V) synthesis has undergone a breakthrough by training transformers or diffusion models on large-scale datasets. Nevertheless, inferring such large models incurs huge costs. Previous inference acceleration works either require costly retraining or are model-specific. To address this issue, instead of retraining we explore the inference process of two mainstream T2V models using transformers and diffusion models. The exploration reveals the redundancy in temporal attention modules of both models, which are commonly utilized to establish temporal relations among frames. Consequently, we propose a training-free and generalized pruning strategy called F3-Pruning to prune redundant temporal attention weights. Specifically, when aggregate temporal attention values are ranked below a certain ratio, corresponding weights will be pruned. Extensive experiments on three datasets using a classic transformer-based model CogVideo and a typical diffusion-based model Tune-A-Video verify the effectiveness of F3-Pruning in inference acceleration, quality assurance and broad applicability.
更多查看译文
关键词
CV: Computational Photography, Image & Video Synthesis,ML: Learning on the Edge & Model Compression
AI 理解论文
溯源树
样例
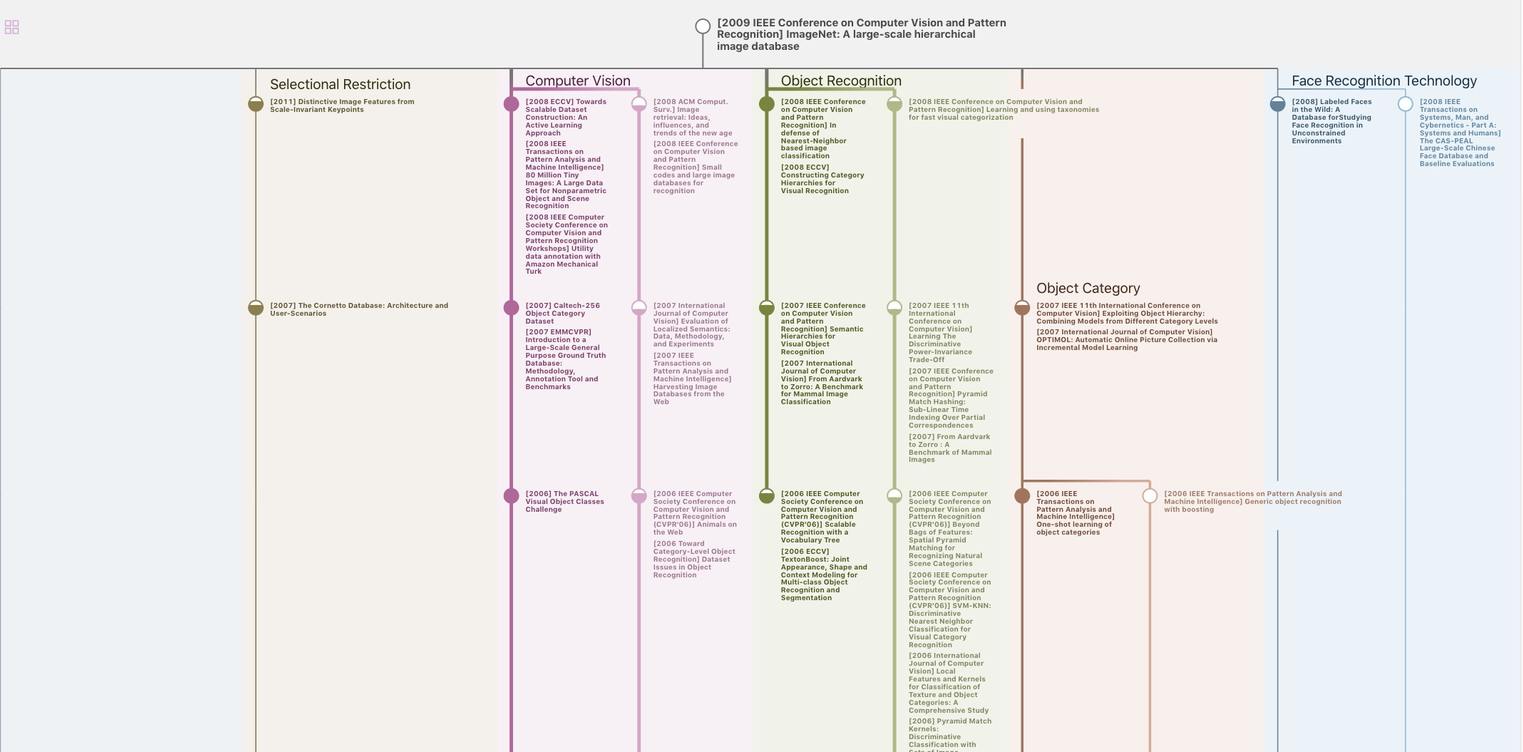
生成溯源树,研究论文发展脉络
Chat Paper
正在生成论文摘要