Learning Task-Aware Language-Image Representation for Class-Incremental Object Detection
AAAI 2024(2024)
摘要
Class-incremental object detection (CIOD) is a real-world desired capability, requiring an object detector to continuously adapt to new tasks without forgetting learned ones, with the main challenge being catastrophic forgetting. Many methods based on distillation and replay have been proposed to alleviate this problem. However, they typically learn on a pure visual backbone, neglecting the powerful representation capabilities of textual cues, which to some extent limits their performance. In this paper, we propose task-aware language-image representation to mitigate catastrophic forgetting, introducing a new paradigm for language-image-based CIOD. First of all, we demonstrate the significant advantage of language-image detectors in mitigating catastrophic forgetting. Secondly, we propose a learning task-aware language-image representation method that overcomes the existing drawback of directly utilizing the language-image detector for CIOD. More specifically, we learn the language-image representation of different tasks through an insulating approach in the training stage, while using the alignment scores produced by task-specific language-image representation in the inference stage. Through our proposed method, language-image detectors can be more practical for CIOD. We conduct extensive experiments on COCO 2017 and Pascal VOC 2007 and demonstrate that the proposed method achieves state-of-the-art results under the various CIOD settings.
更多查看译文
关键词
CV: Object Detection & Categorization,ML: Life-Long and Continual Learning
AI 理解论文
溯源树
样例
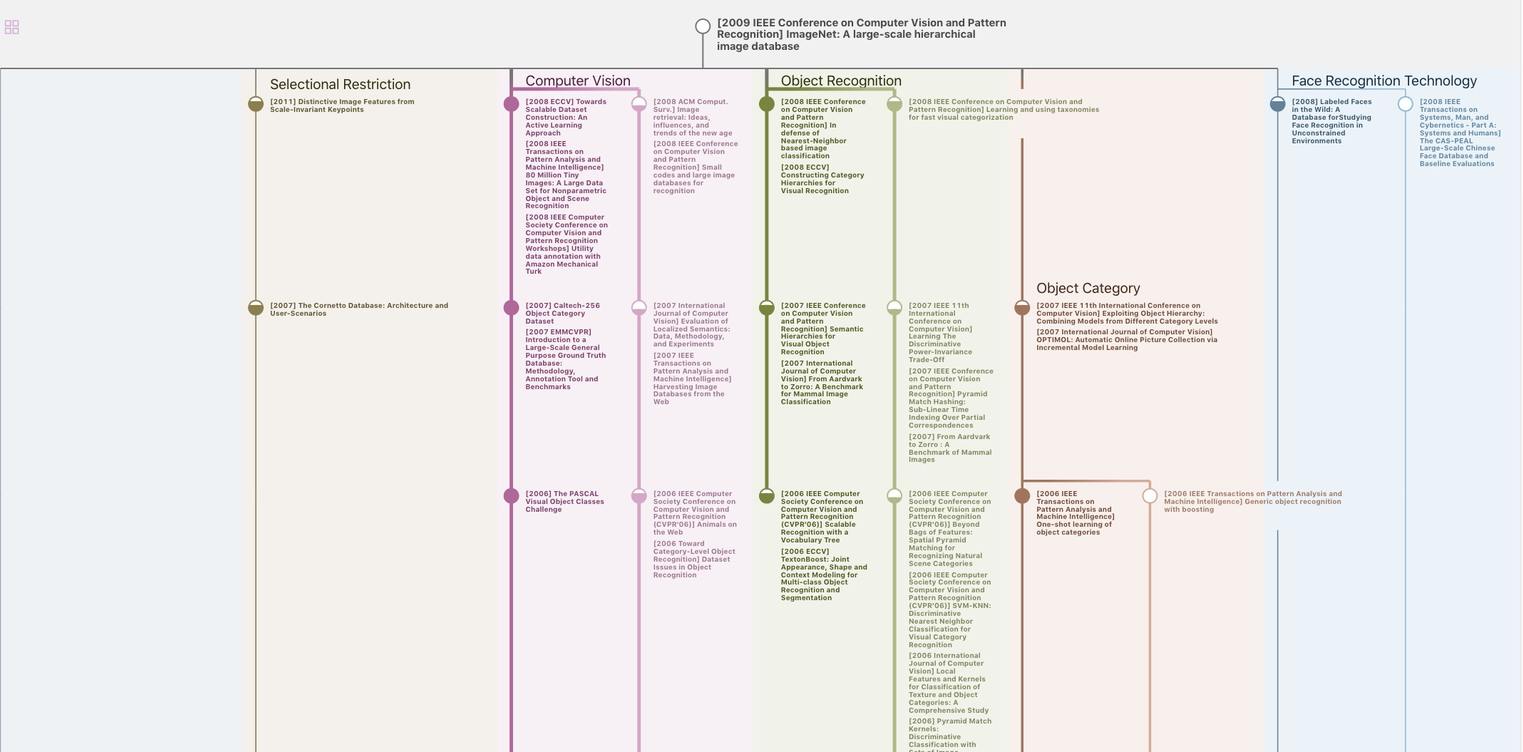
生成溯源树,研究论文发展脉络
Chat Paper
正在生成论文摘要