MFFnet: A Seismic Phase Picking Network Based on Multiple Feature Fusion
IEEE GEOSCIENCE AND REMOTE SENSING LETTERS(2024)
摘要
With the recent improvement of deep learning (DL) techniques and computer hardware capabilities, neural networks are widely used to monitor massive sensor data and detect earthquakes in them. This makes designing fast, accurate, and generalized DL models necessary for an active field of research for automatic seismic phase picking. A seismic phase picking network called MFFnet is proposed to fuse power spectral density (PSD), expert knowledge, spectrograms, recurrence plots (RPs), and Gramian angle fields. The network uses fast Fourier convolution (FFC) on 2-D representations to extract more interpretable features. Considering the high proportion of noisy signals in field applications, MFFnet uses focal loss (FL) as the loss function to improve network accuracy. Experimental results show that MFFnet achieves precision, recall, and accuracy with 0.96, 0.98, and 0.98, respectively, in seismic phase detection tasks. Shapley value is used to evaluate the relationship between features and network predictions. Compared with other DL networks, the feature extraction approach used in this letter is more explanatory and provides greater confidence in the results.
更多查看译文
关键词
Feature extraction,Convolution,Spectrogram,Training,Long short term memory,Earthquakes,Time series analysis,Deep learning (DL),feature fusion,seismic phase picking,Shapley value,fast Fourier convolution (FFC)
AI 理解论文
溯源树
样例
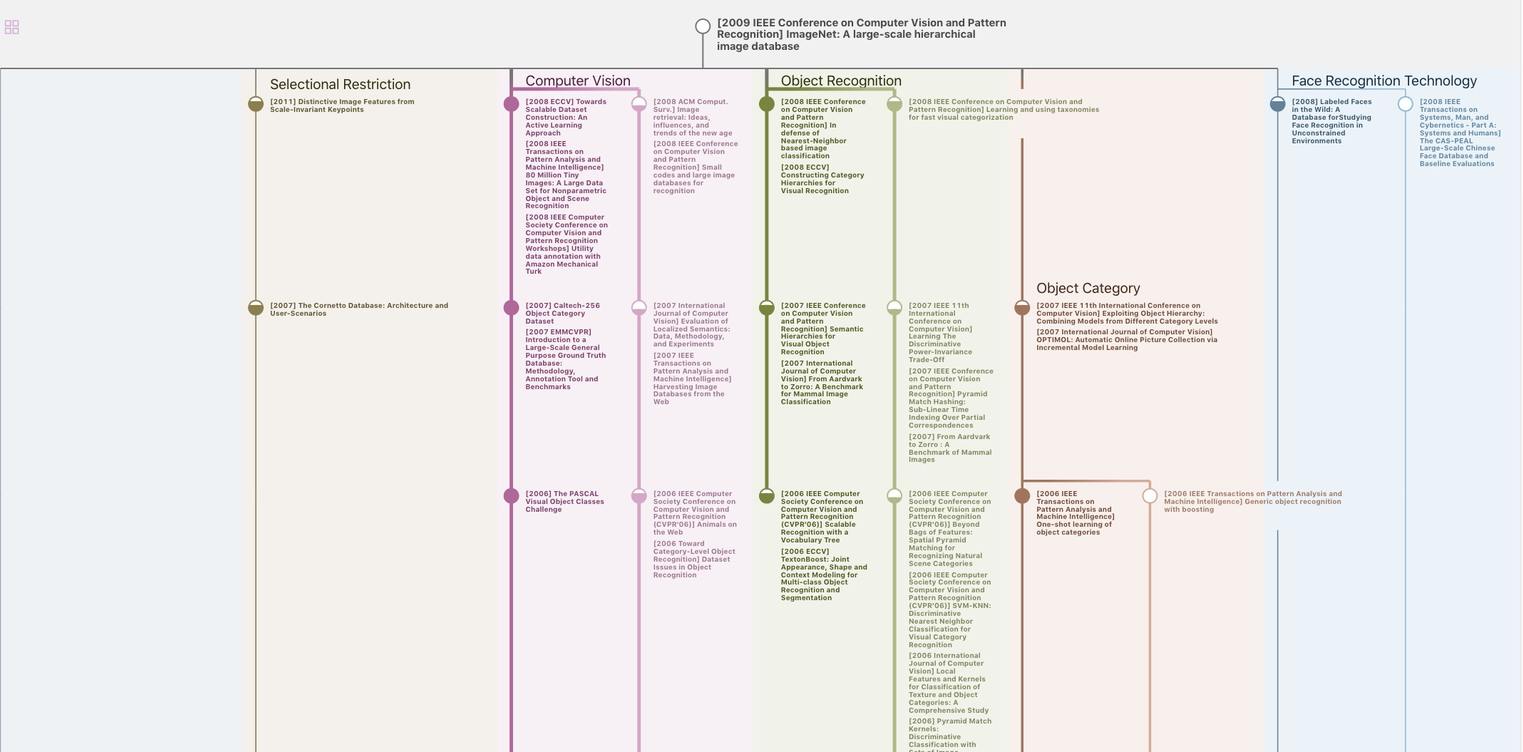
生成溯源树,研究论文发展脉络
Chat Paper
正在生成论文摘要