Deep-Learning-Based Force Sensing Method for a Flexible Endovascular Surgery Robot
IEEE TRANSACTIONS ON INSTRUMENTATION AND MEASUREMENT(2024)
摘要
Endovascular surgical robots (ESRs) are extensively researched because of their potential to minimize surgeons' radiation exposure. However, during the surgical operation, it is difficult for ESR to achieve the same flexibility in manipulating the soft catheter and the slender guidewire as human fingers. The flexibility of ESR can be enhanced by incorporating a soft gripper, but the nonlinear deformation of soft material presents challenges in sensing and controlling the force. To address these issues, this study proposes a deep-learning-based force sensing method that enables the flexible ESR to measure the surgical force and torque. The proposed deep-learning model consists of multiple-layer long short-term memory (LSTM) modules and is trained using the robot's motion and soft gripper deformation datasets. The well-trained LSTM model can predict the operating force and torque in real-time. The contrast experiments with other models demonstrate that our robot exhibits higher force measurement accuracy. Furthermore, a force control strategy is proposed for the application of LSTM-based ESR. In the force control strategy, the robot is encouraged to mimic the dexterity of the surgeon's fingers and to maintain the force within a safe range. Finally, the proposed strategy is compared with other strategies in vascular phantom experiments and is proven to effectively enhance the safety and efficiency of surgical operations.
更多查看译文
关键词
Deep learning,flexible endovascular surgery robot,force control,force sensing,long short-term memory (LSTM)
AI 理解论文
溯源树
样例
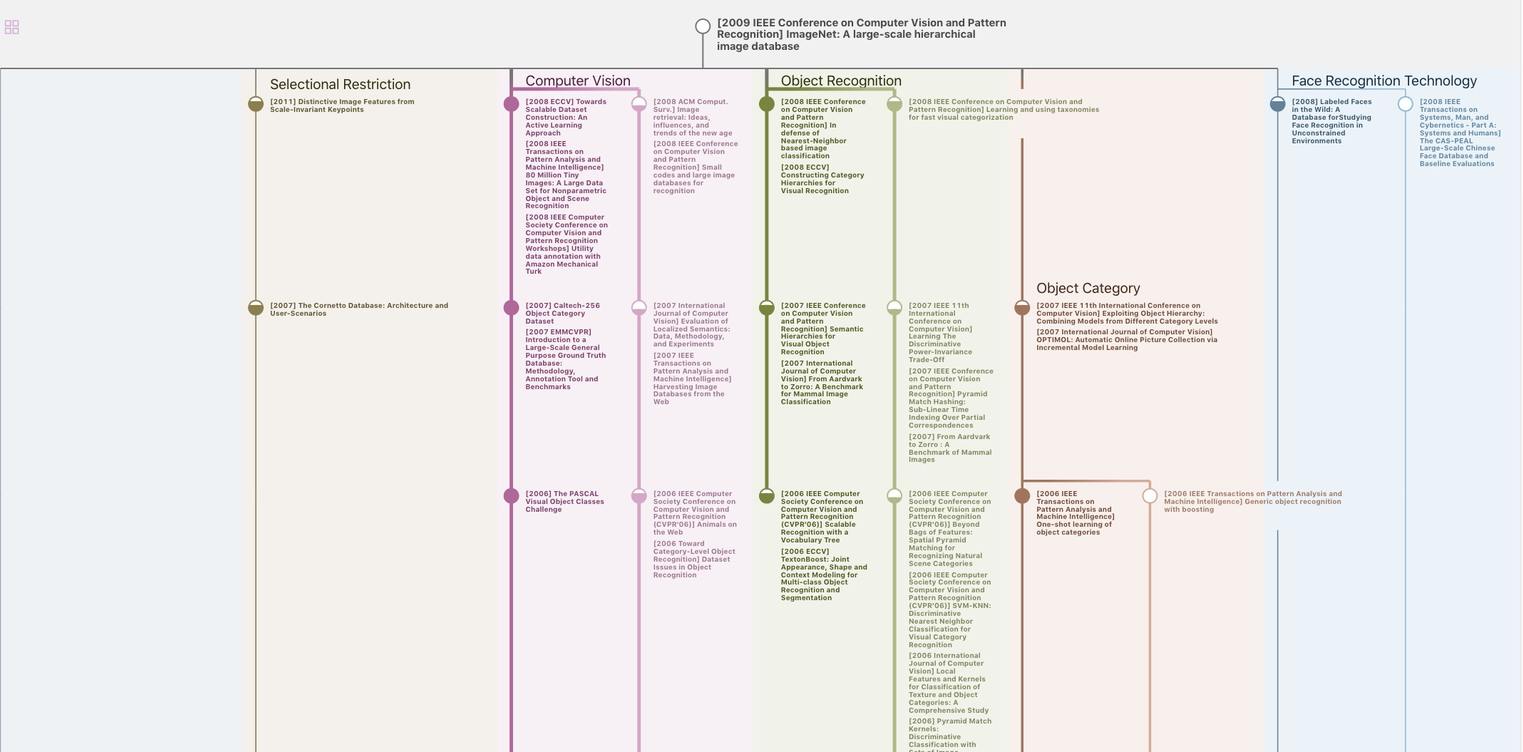
生成溯源树,研究论文发展脉络
Chat Paper
正在生成论文摘要