A Locality-Sensitive Hashing based Collaborative Recommendation Method for Responsible AI Driven Recommender Systems
IEEE Transactions on Artificial Intelligence(2024)
摘要
As one of the most representative recommendation solutions, traditional collaborative filtering models typically have limitations in dealing with large-scale, sparse data to capture complex relationships between users and items. The rise of Artificial Intelligence (AI) provides powerful tools such as deep neural networks to overcome the data sparsity issue with typical collaborative filtering models. Existing works on AI driven recommender systems are focusing on improving recommendation accuracy by extracting rich user/item features from multi-source data with deep learning tools to build more accurate user preference model. How to ensure the responsibility of AI driven recommender systems is still a big challenge. On one hand, AI driven recommender systems need to access raw data such as user profiles to train recommendation model, which face the risk of leaking user privacy information. On the other hand, the ever increasing volume of user/item interaction records raise the efficiency challenge to provide recommendation results in a real time manner. In view of those observations, we propose a collaborative recommendation method by adopting the Locality-Sensitive-Hashing technique (i.e.,
DisRecLSH
), which aims to achieve the goal of privacy protection and calculation efficiency for AI driven recommender systems. More specifically, we split
DisRecLSH
into three phases: (1) Offline feature extraction: deep neural networks are applied to extract user/item features from multi-source user/item data on each local data set. (2) Offline user index building: Locality Sensitive Hashing technique is adopted to map user features to hash codes to quickly recall correlated similar users for a given target user. (3) Online Top-N items calculation: with similar users selected by phase 2, Top-N items are calculated based on the ranking of predicted user-item rating score. Finally, extensive experiments are conducted on three public datasets to evaluate the efficiency of our proposal.
更多查看译文
关键词
collaborative filtering,locality-sensitive-hashing,AI-driven recommender systems,privacy protection
AI 理解论文
溯源树
样例
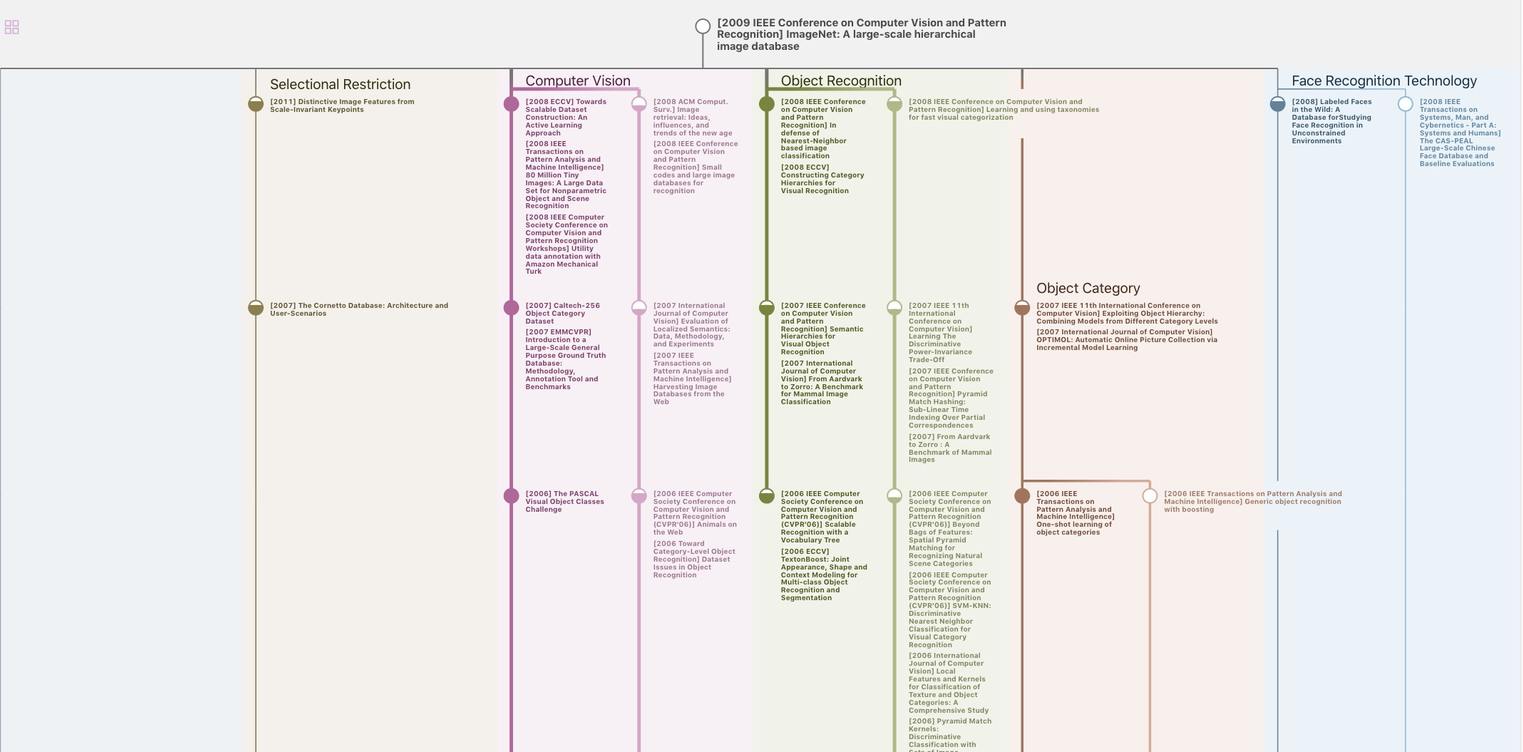
生成溯源树,研究论文发展脉络
Chat Paper
正在生成论文摘要