Visual Redundancy Removal for Composite Images: A Benchmark Dataset and a Multi-Visual-Effects Driven Incremental Method
AAAI 2024(2024)
摘要
Composite images (CIs) typically combine various elements from different scenes, views, and styles, which are a very important information carrier in the era of mixed media such as virtual reality, mixed reality, metaverse, etc. However, the complexity of CI content presents a significant challenge for subsequent visual perception modeling and compression. In addition, the lack of benchmark CI databases also hinders the use of recent advanced data-driven methods. To address these challenges, we first establish one of the earliest visual redundancy prediction (VRP) databases for CIs. Moreover, we propose a multi-visual effect (MVE)-driven incremental learning method that combines the strengths of hand-crafted and data-driven approaches to achieve more accurate VRP modeling. Specifically, we design special incremental rules to learn the visual knowledge flow of MVE. To effectively capture the associated features of MVE, we further develop a three-stage incremental learning approach for VRP based on an encoder-decoder network. Extensive experimental results validate the superiority of the proposed method in terms of subjective, objective, and compression experiments.
更多查看译文
关键词
HAI: Applications,HAI: Learning Human Values and Preferences,HAI: Other Foundations of Human Computation & AI
AI 理解论文
溯源树
样例
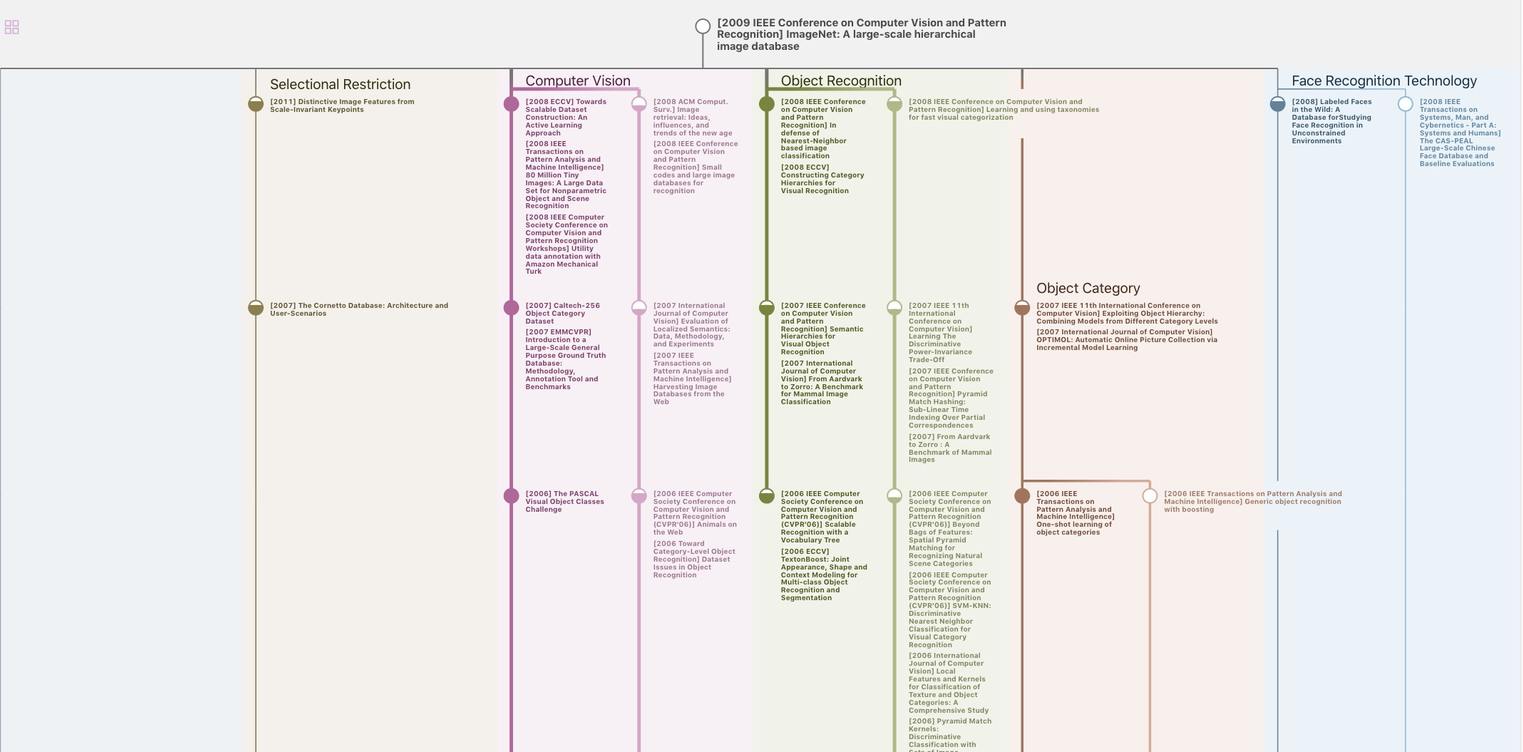
生成溯源树,研究论文发展脉络
Chat Paper
正在生成论文摘要