Structural Information Enhanced Graph Representation for Link Prediction
AAAI 2024(2024)
摘要
Link prediction is a fundamental task of graph machine learning, and Graph Neural Network (GNN) based methods have become the mainstream approach due to their good performance. However, the typical practice learns node representations through neighborhood aggregation, lacking awareness of the structural relationships between target nodes. Recently, some methods have attempted to address this issue by node labeling tricks. However, they still rely on the node-centric neighborhood message passing of GNNs, which we believe involves two limitations in terms of information perception and transmission for link prediction. First, it cannot perceive long-range structural information due to the restricted receptive fields. Second, there may be information loss of node-centric model on link-centric task. In addition, we empirically find that the neighbor node features could introduce noise for link prediction. To address these issues, we propose a structural information enhanced link prediction framework, which involves removing the neighbor node features while fitting neighborhood graph structures more focused through GNN. Furthermore, we introduce Binary Structural Transformer (BST) to encode the structural relationships between target nodes, complementing the deficiency of GNN. Our approach achieves remarkable results on multiple popular benchmarks, including ranking first on ogbl-ppa, ogbl-citation2 and Pubmed.
更多查看译文
关键词
ML: Graph-based Machine Learning,DMKM: Graph Mining, Social Network Analysis & Community,DMKM: Representing, Reasoning, and Using Provenance, Trust
AI 理解论文
溯源树
样例
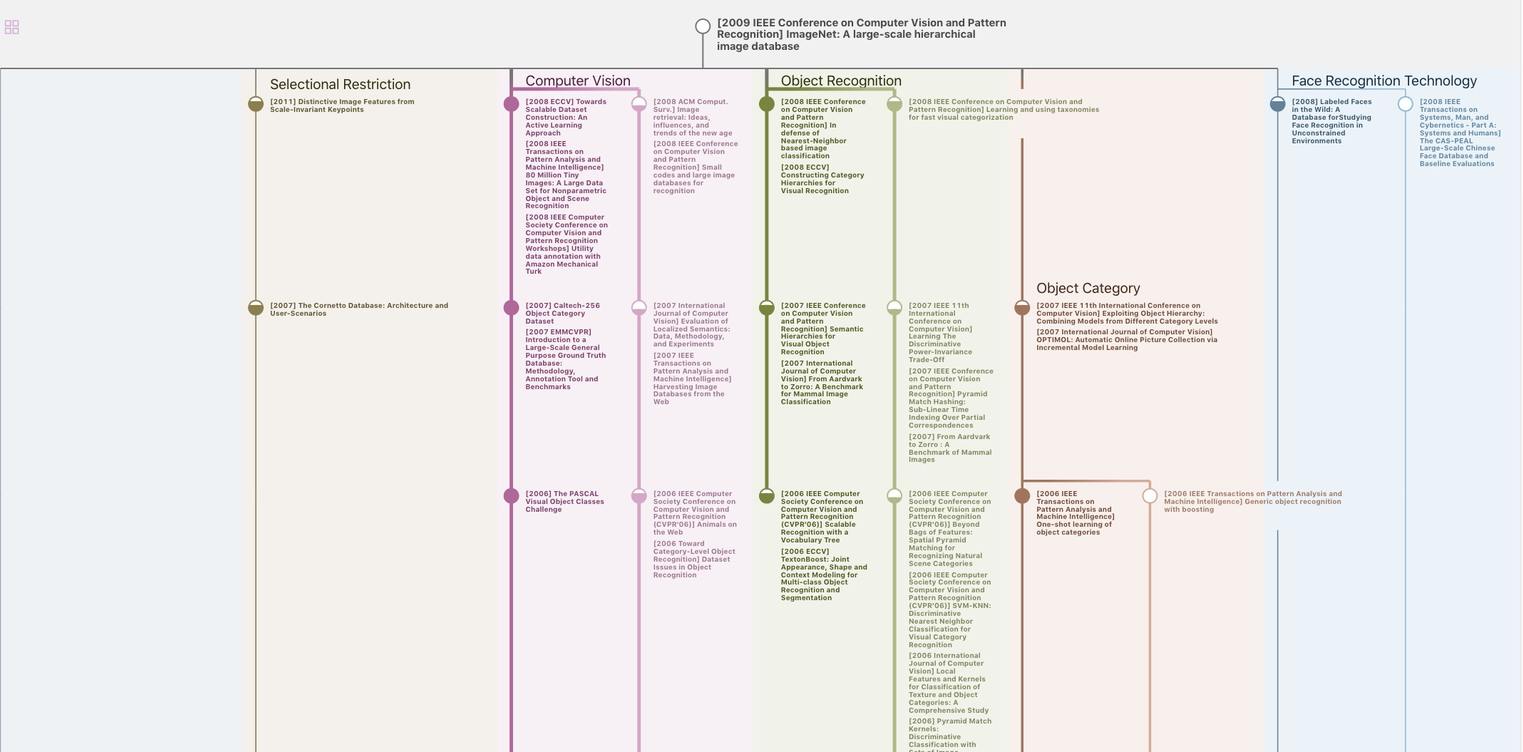
生成溯源树,研究论文发展脉络
Chat Paper
正在生成论文摘要