Enhanced Fine-Grained Motion Diffusion for Text-Driven Human Motion Synthesis
AAAI 2024(2024)
摘要
The emergence of text-driven motion synthesis technique provides animators with great potential to create efficiently. However, in most cases, textual expressions only contain general and qualitative motion descriptions, while lack fine depiction and sufficient intensity, leading to the synthesized motions that either (a) semantically compliant but uncontrollable over specific pose details, or (b) even deviates from the provided descriptions, bringing animators with undesired cases. In this paper, we propose DiffKFC, a conditional diffusion model for text-driven motion synthesis with KeyFrames Collaborated, enabling realistic generation with collaborative and efficient dual-level control: coarse guidance at semantic level, with only few keyframes for direct and fine-grained depiction down to body posture level. Unlike existing inference-editing diffusion models that incorporate conditions without training, our conditional diffusion model is explicitly trained and can fully exploit correlations among texts, keyframes and the diffused target frames. To preserve the control capability of discrete and sparse keyframes, we customize dilated mask attention modules where only partial valid tokens participate in local-to-global attention, indicated by the dilated keyframe mask. Additionally, we develop a simple yet effective smoothness prior, which steers the generated frames towards seamless keyframe transitions at inference. Extensive experiments show that our model not only achieves state-of-the-art performance in terms of semantic fidelity, but more importantly, is able to satisfy animator requirements through fine-grained guidance without tedious labor.
更多查看译文
关键词
CV: Biometrics, Face, Gesture & Pose,CV: Language and Vision,CV: Vision for Robotics & Autonomous Driving,HAI: Applications
AI 理解论文
溯源树
样例
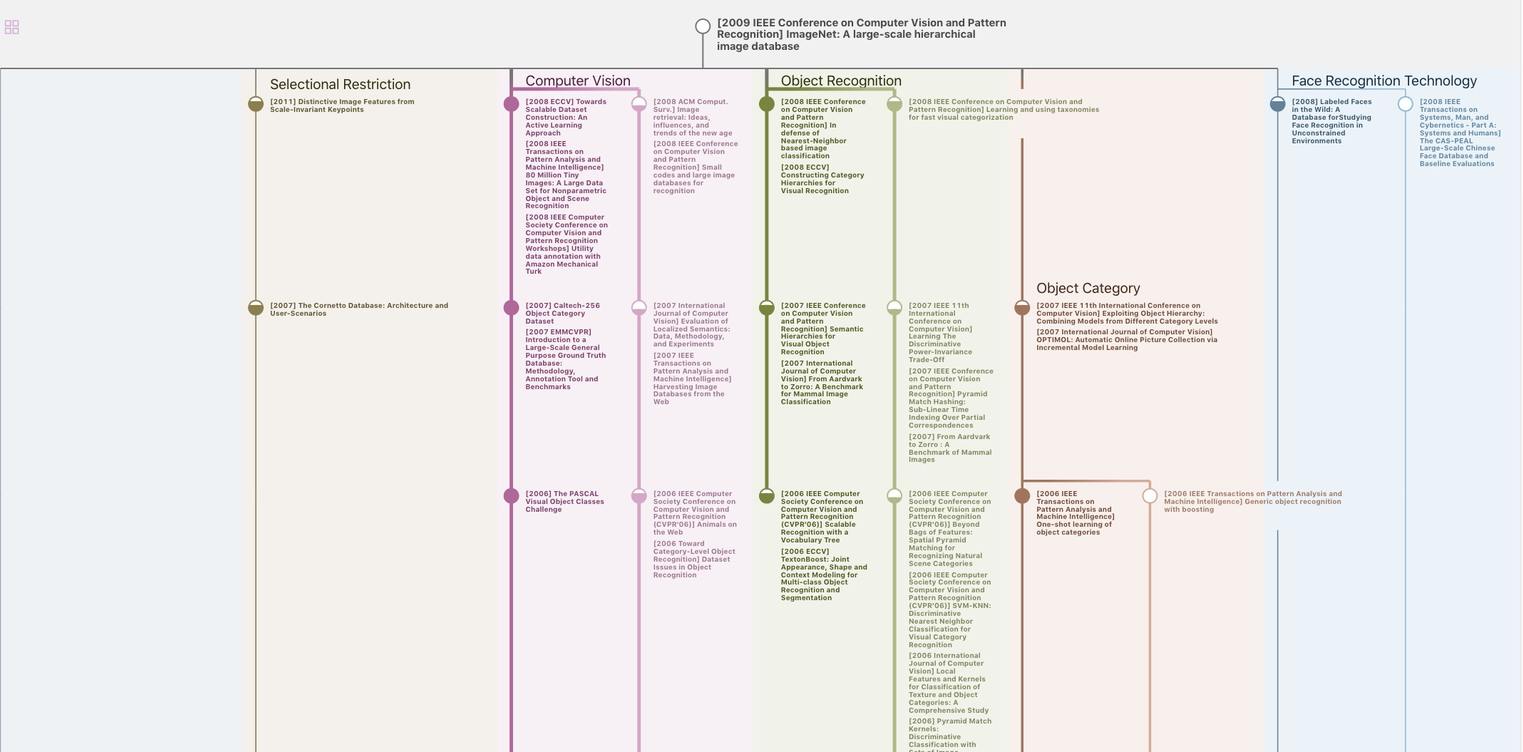
生成溯源树,研究论文发展脉络
Chat Paper
正在生成论文摘要