Learning Representations for Robust Human-Robot Interaction
AAAI 2024(2024)
摘要
For robots to robustly and flexibly interact with humans, they need to acquire skills to use across scenarios. One way to enable the generalization of skills is to learn representations that are useful for downstream tasks. Learning a representation for interactions requires an understanding of what (e.g., objects) as well as how (e.g., actions, controls, and manners) to interact with. However, most existing language or visual representations mainly focus on objects. To enable robust human-robot interactions, we need a representation that is not just grounded at the object level but to reason at the action level. The ability to reason about an agent’s own actions and other’s actions will be crucial for long-tail interactions. My research focuses on leveraging the compositional nature of language and reward functions to learn representations that generalize to novel scenarios. Together with the information from multiple modalities, the learned representation can reason about task progress, future behaviors, and the goals/beliefs of an agent. The above ideas have been demonstrated in my research on building robots to understand language and engage in social interactions.
更多查看译文
关键词
Human-robot Interaction,Language Representations,Theory Of Mind,Grounding
AI 理解论文
溯源树
样例
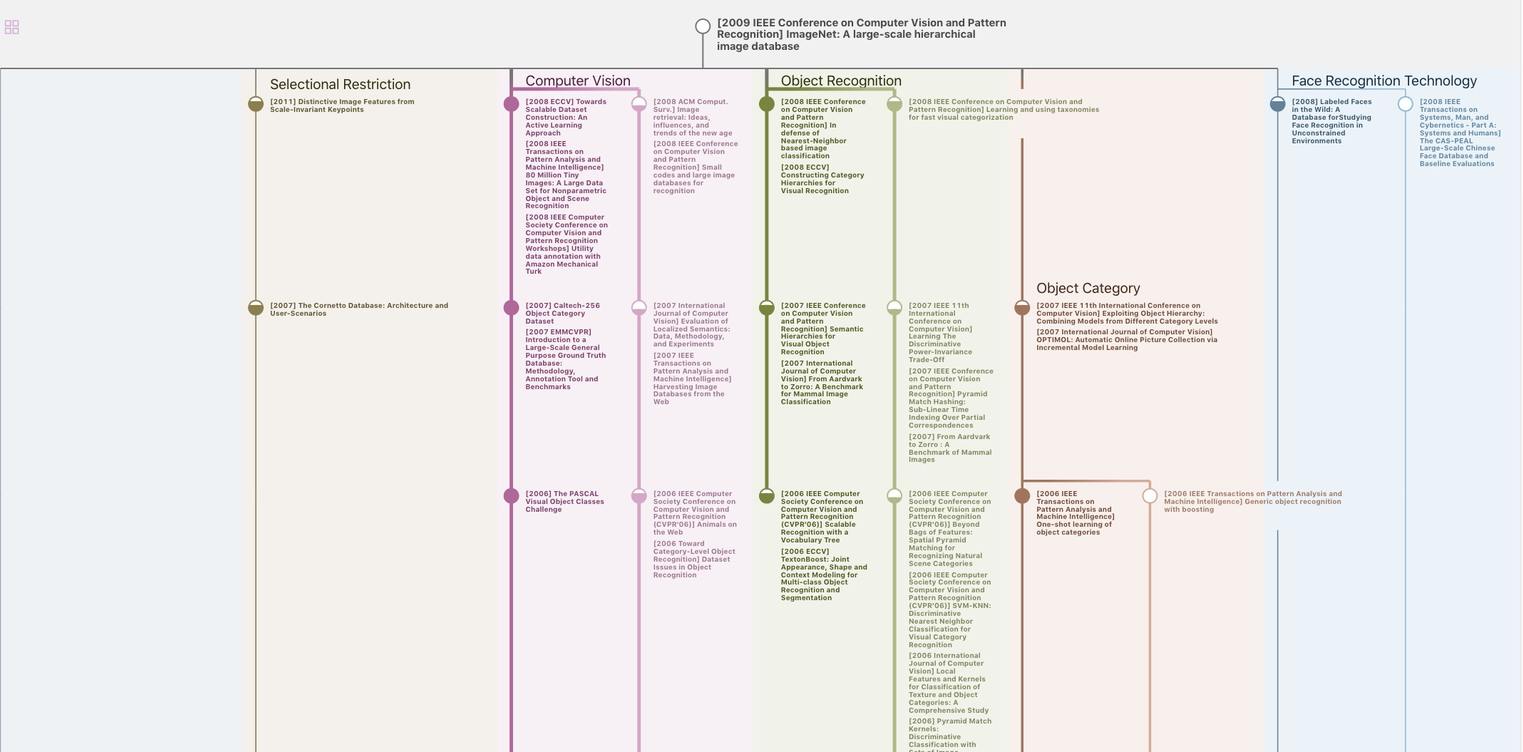
生成溯源树,研究论文发展脉络
Chat Paper
正在生成论文摘要