An Optimal Transport View for Subspace Clustering and Spectral Clustering
AAAI 2024(2024)
摘要
Clustering is one of the most fundamental problems in machine learning and data mining, and many algorithms have been proposed in the past decades. Among them, subspace clustering and spectral clustering are the most famous approaches. In this paper, we provide an explanation for subspace clustering and spectral clustering from the perspective of optimal transport. Optimal transport studies how to move samples from one distribution to another distribution with minimal transport cost, and has shown a powerful ability to extract geometric information. By considering a self optimal transport model with only one group of samples, we observe that both subspace clustering and spectral clustering can be explained in the framework of optimal transport, and the optimal transport matrix bridges the spaces of features and spectral embeddings. Inspired by this connection, we propose a spectral optimal transport barycenter model, which learns spectral embeddings by solving a barycenter problem equipped with an optimal transport discrepancy and guidance of data. Based on our proposed model, we take advantage of optimal transport to exploit both feature and metric information involved in data for learning coupled spectral embeddings and affinity matrix in a unified model. We develop an alternating optimization algorithm to solve the resultant problems, and conduct experiments in different settings to evaluate the performance of our proposed methods.
更多查看译文
关键词
ML: Clustering
AI 理解论文
溯源树
样例
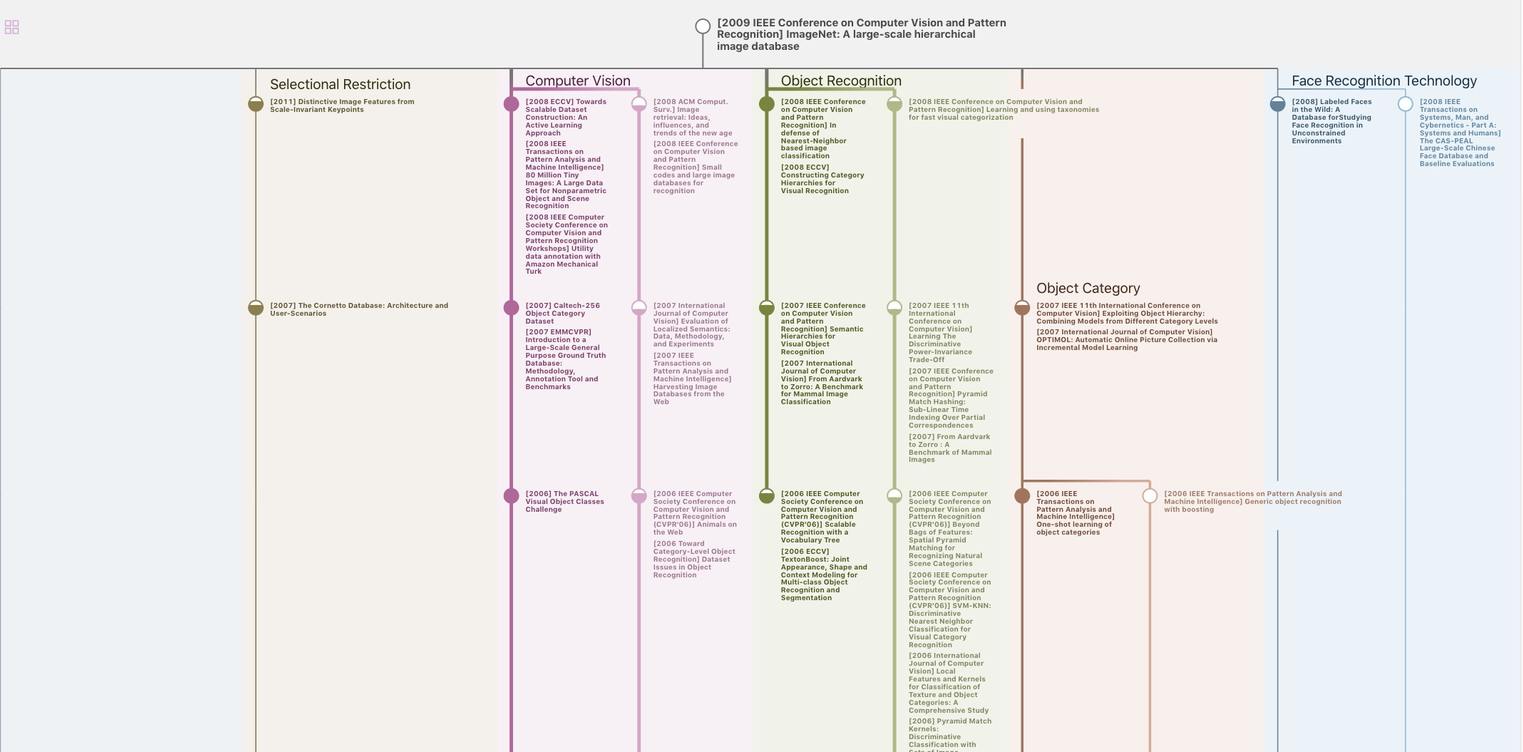
生成溯源树,研究论文发展脉络
Chat Paper
正在生成论文摘要