APPLE: An Explainer of ML Predictions on Circuit Layout at the Circuit-Element Level
2024 29th Asia and South Pacific Design Automation Conference (ASP-DAC)(2024)
摘要
In recent years, we have witnessed many excellent machine learning (ML) solutions targeting circuit layouts. These ML models provide fast predictions on various design objectives. However, almost all existing ML solutions have neglected the basic interpretability requirement from potential users. As a result, it is very difficult for users to figure out any potential accuracy degradation or abnormal behaviors of given ML models. In this work, we propose a new technique named APPLE to explain each ML prediction at the resolution level of circuit elements. To the best of our knowledge, this is the first effort to explain ML predictions on circuit layouts. It provides a significantly more reasonable, useful, and efficient explanation for lithography hotspot prediction, compared with the highest-cited prior solution for natural images.
更多查看译文
关键词
Machine Learning Prediction,Circuit Elements,Circuit Layout,Potential Utility,Machine Learning Models,Reasonable Explanation,Machine Learning Solutions,Accuracy Of Model,Convolutional Neural Network,Machine Learning Techniques,Test Accuracy,Positive Predictor,Input Samples,Negative Predictor,Decision Boundary,Feature Engineering,Malicious Attacks,Adversarial Attacks,Discrete Cosine Transform,Correct Pattern,Wrong Predictions,Neighboring Elements,Accuracy Of Machine Learning Models,Accurate Machine Learning,Design Manufacturing,Higher Test Accuracy,Test Circuit,Test Samples,Complex Samples,Positive Samples
AI 理解论文
溯源树
样例
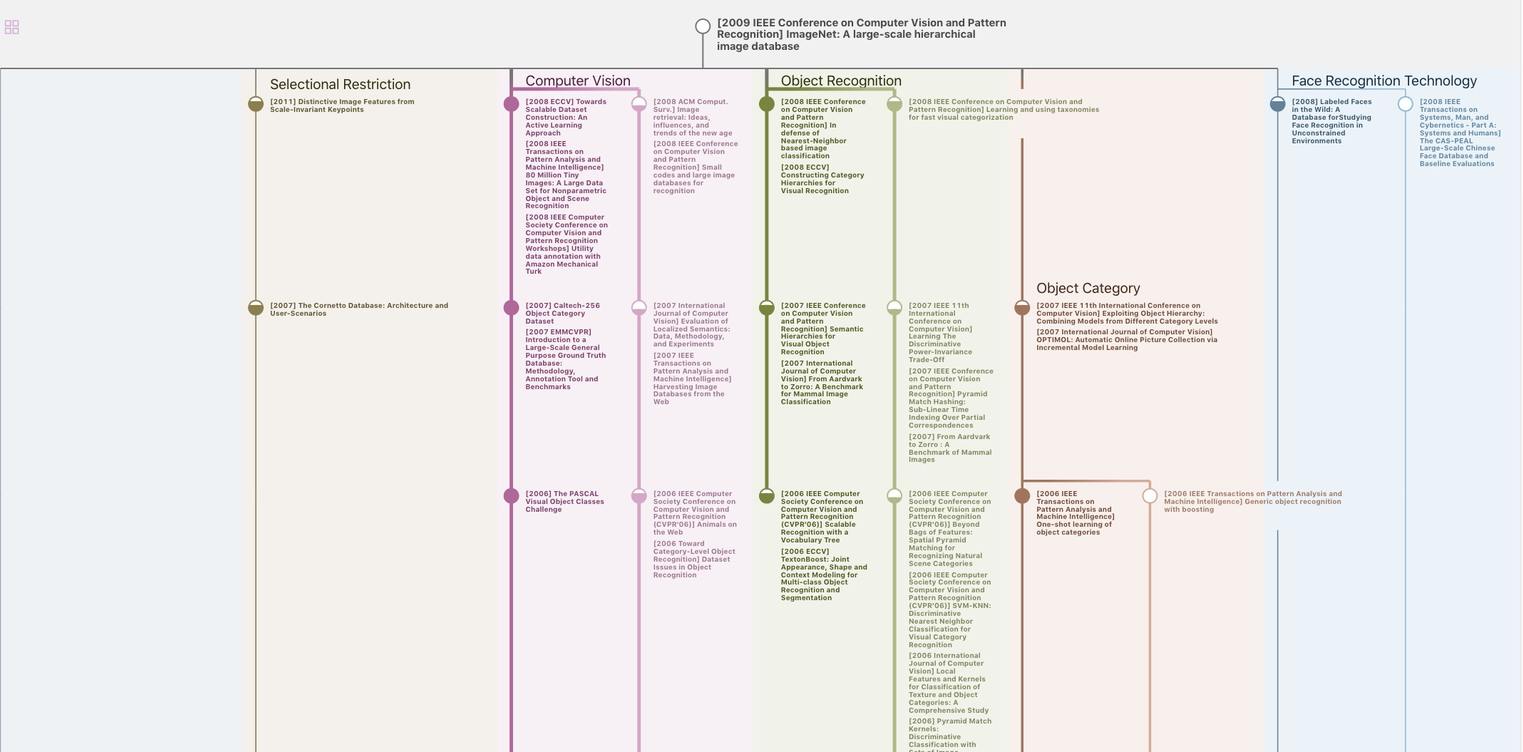
生成溯源树,研究论文发展脉络
Chat Paper
正在生成论文摘要