Unsupervised Object Interaction Learning with Counterfactual Dynamics Models
AAAI 2024(2024)
摘要
We present COIL (Counterfactual Object Interaction Learning), a novel way of learning skills of object interactions on entity-centric environments. The goal is to learn primitive behaviors that can induce interactions without external reward or any supervision. Existing skill discovery methods are limited to locomotion, simple navigation tasks, or single-object manipulation tasks, mostly not inducing interaction between objects. Unlike a monolithic representation usually used in prior skill learning methods, we propose to use a structured goal representation that can query and scope which objects to interact with, which can serve as a basis for solving more complex downstream tasks. We design a novel counterfactual intrinsic reward through the use of either a forward model or successor features that can learn an interaction skill between a pair of objects given as a goal. Through experiments on continuous control environments such as Magnetic Block and 2.5-D Stacking Box, we demonstrate that an agent can learn object interaction behaviors (e.g., attaching or stacking one block to another) without any external rewards or domain-specific knowledge.
更多查看译文
关键词
ML: Reinforcement Learning,ML: Unsupervised & Self-Supervised Learning
AI 理解论文
溯源树
样例
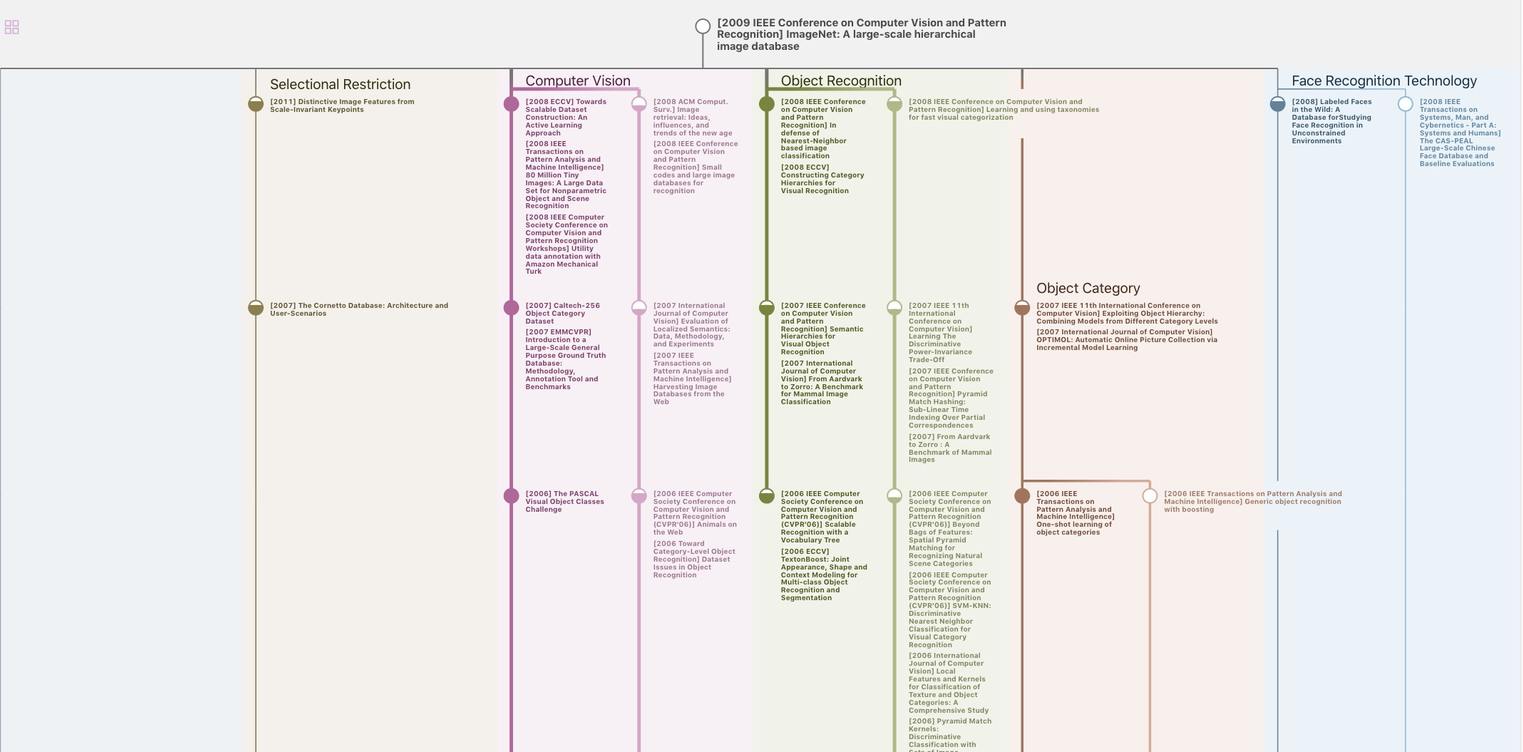
生成溯源树,研究论文发展脉络
Chat Paper
正在生成论文摘要