Correlation Fusion with Instance-level Auto-weighted for Multi-view Graph Clustering.
IEEE International Conference on Smart City(2023)
摘要
Multi-view graph clustering (MGC) has emerged as a hot research topic due to its effectiveness. Previous works mainly focus on the fusion of similarity graphs to exploit the complementary and consistent. However, these approaches suffer from three shortcomings: 1) The distance similarity estimate by the linear Euclidean metric may be incorrect in revealing the association relationship of heterogeneous multi-view samples. 2) Most of them often weight the whole view to balance the effect of different views, which ignores the importance of local samples. 3) They fail to preserve the inherent manifold structure between views via directly applying similarity matrix fusion to obtain a consistent graph. To fill these gaps, this paper proposed a novel framework called correlation fusion with instance-level auto-weighted for MGC (CFIMGC). In particular, the Spearman distance is leveraged to effectively characterize the versatile correlation matrix. The sample-level auto-weighted fusion mechanism is presented for flexible assigning weight values to different sample-wise relations in intra-view and inter-view, through which the complementary of numerous correlation matrices is appropri-ately explored to produce a consistent correlation matrix. What's more, a probability graph is adaptively learned based on the fusion correlation matrix for constructing a clustering-friendly similarity graph. Meanwhile, an alternating iteration algorithm is devised to address the non-convex joint optimization problem. Comprehensive experiment results on seven widely used but challenging multi-view datasets demonstrate that the proposed method yields superiority in clustering performance against six state-of-the-art counterparts.
更多查看译文
关键词
multi-view graph clustering,graph learning,correlation fusion,instance-level weighting
AI 理解论文
溯源树
样例
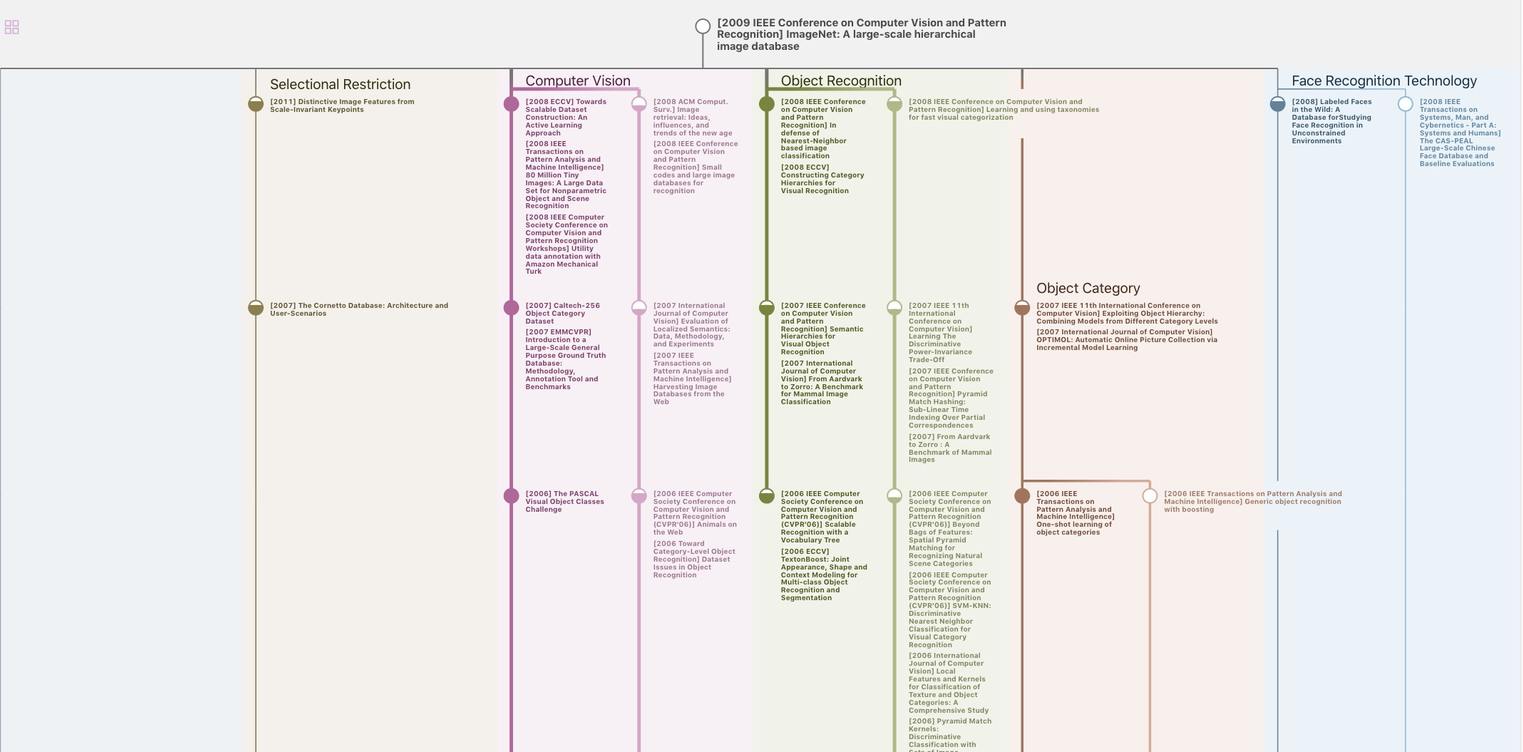
生成溯源树,研究论文发展脉络
Chat Paper
正在生成论文摘要