Short-Term Load Forecasting Using Multidimensional Long Time Series: A GMM- LSTNET Approach
2023 4th International Conference on Power Engineering (ICPE)(2023)
摘要
Short-term load forecasting is essential for optimizing energy generation and distribution, aiding in grid stability and reliability, and facilitating efficient energy resource allocation. It also enables timely decision-making in energy management and minimizes operational costs and environmental impacts. This study presents a novel approach for short-term load prediction by integrating the Gaussian mixture model (GMM) and Long and Short-term Time-series network (LSTNET). The proposed method utilizes multi-dimensional feature data from long time series to forecast short-term loads. LSTNET incorporates a traditional autoregressive linear model into a nonlinear neural network component, thereby enhancing the robustness of the nonlinear deep learning model for time series data with irregular scale changes. Additionally, the attention mechanism is employed to tackle the challenge of sequences with unclear or variable periodicity. Experimental results demonstrate the effectiveness of the proposed model in achieving accurate short-term load prediction.
更多查看译文
关键词
GMM,LSTNet,Short-term load forecasting,Time series data
AI 理解论文
溯源树
样例
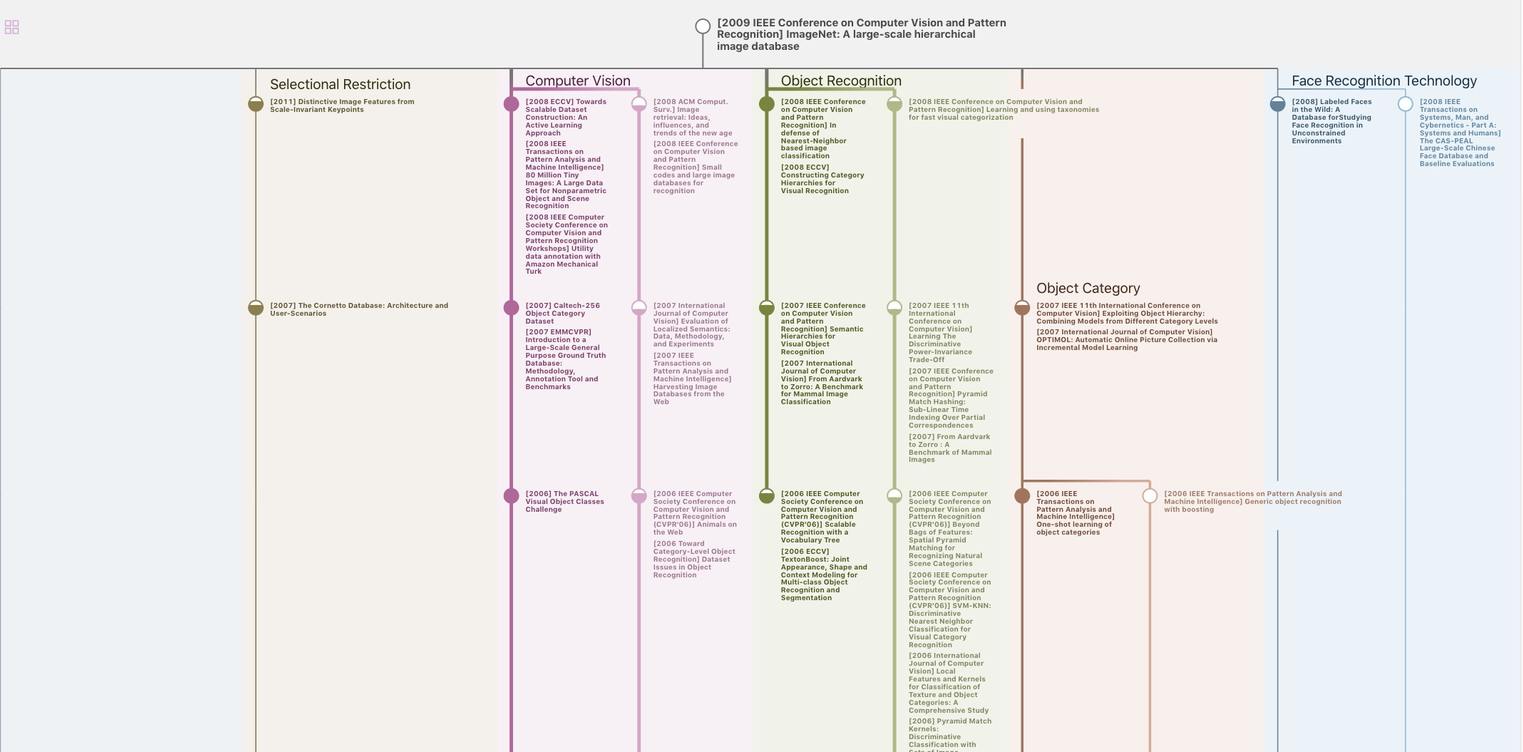
生成溯源树,研究论文发展脉络
Chat Paper
正在生成论文摘要