REGLO: Provable Neural Network Repair for Global Robustness Properties
AAAI 2024(2024)
摘要
We present REGLO, a novel methodology for repairing pretrained neural networks to satisfy global robustness and individual fairness properties. A neural network is said to be globally robust with respect to a given input region if and only if all the input points in the region are locally robust. This notion of global robustness also captures the notion of individual fairness as a special case. We prove that any counterexample to a global robustness property must exhibit a corresponding large gradient. For ReLU networks, this result allows us to efficiently identify the linear regions that violate a given global robustness property. By formulating and solving a suitable robust convex optimization problem, REGLO then computes a minimal weight change that will provably repair these violating linear regions.
更多查看译文
关键词
ML: Ethics, Bias, and Fairness,ML: Adversarial Learning & Robustness,ML: Privacy
AI 理解论文
溯源树
样例
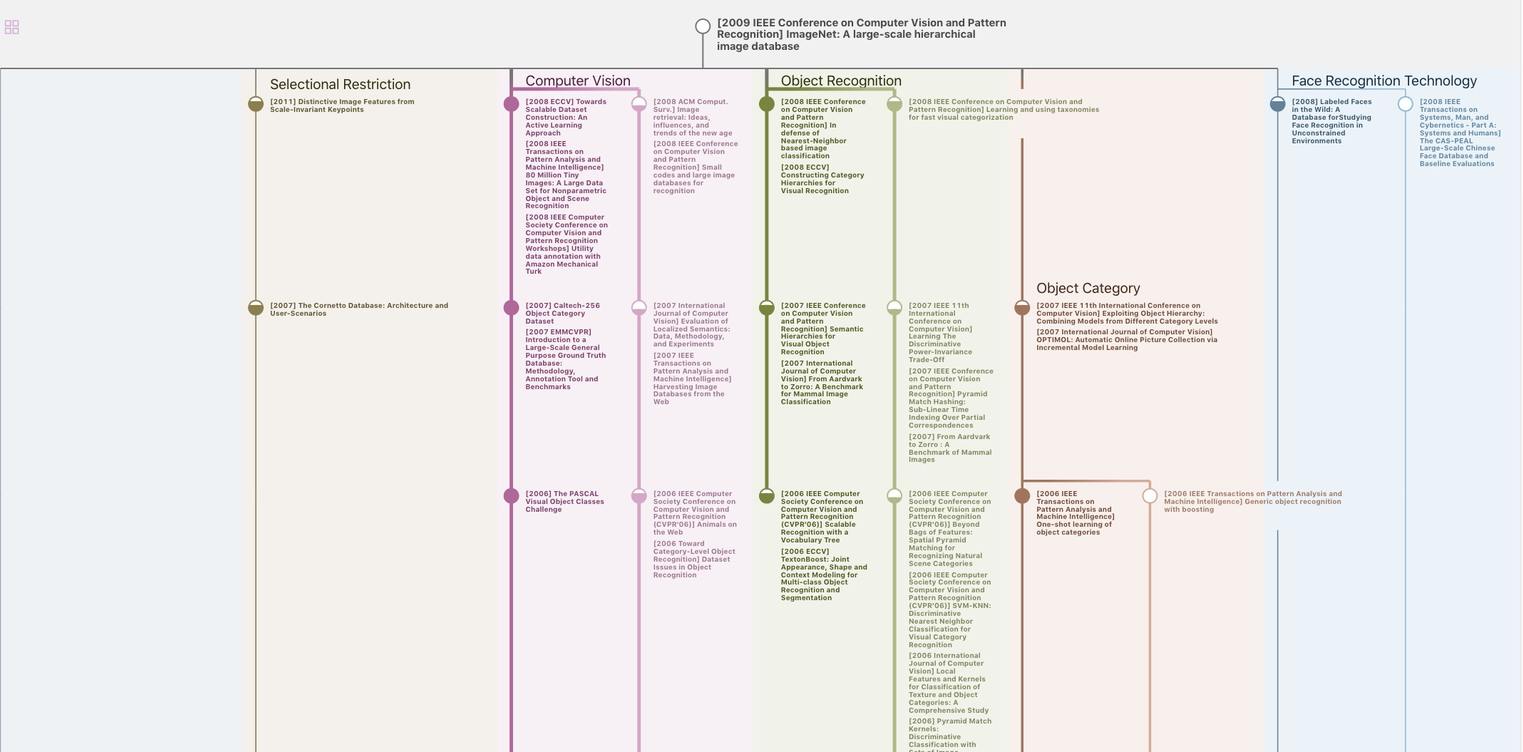
生成溯源树,研究论文发展脉络
Chat Paper
正在生成论文摘要