MGQFormer: Mask-Guided Query-Based Transformer for Image Manipulation Localization
AAAI 2024(2024)
摘要
Deep learning-based models have made great progress in image tampering localization, which aims to distinguish between manipulated and authentic regions. However, these models suffer from inefficient training. This is because they use ground-truth mask labels mainly through the cross-entropy loss, which prioritizes per-pixel precision but disregards the spatial location and shape details of manipulated regions. To address this problem, we propose a Mask-Guided Query-based Transformer Framework (MGQFormer), which uses ground-truth masks to guide the learnable query token (LQT) in identifying the forged regions. Specifically, we extract feature embeddings of ground-truth masks as the guiding query token (GQT) and feed GQT and LQT into MGQFormer to estimate fake regions, respectively. Then we make MGQFormer learn the position and shape information in ground-truth mask labels by proposing a mask-guided loss to reduce the feature distance between GQT and LQT. We also observe that such mask-guided training strategy has a significant impact on the convergence speed of MGQFormer training. Extensive experiments on multiple benchmarks show that our method significantly improves over state-of-the-art methods.
更多查看译文
关键词
CV: Segmentation,CV: Object Detection & Categorization
AI 理解论文
溯源树
样例
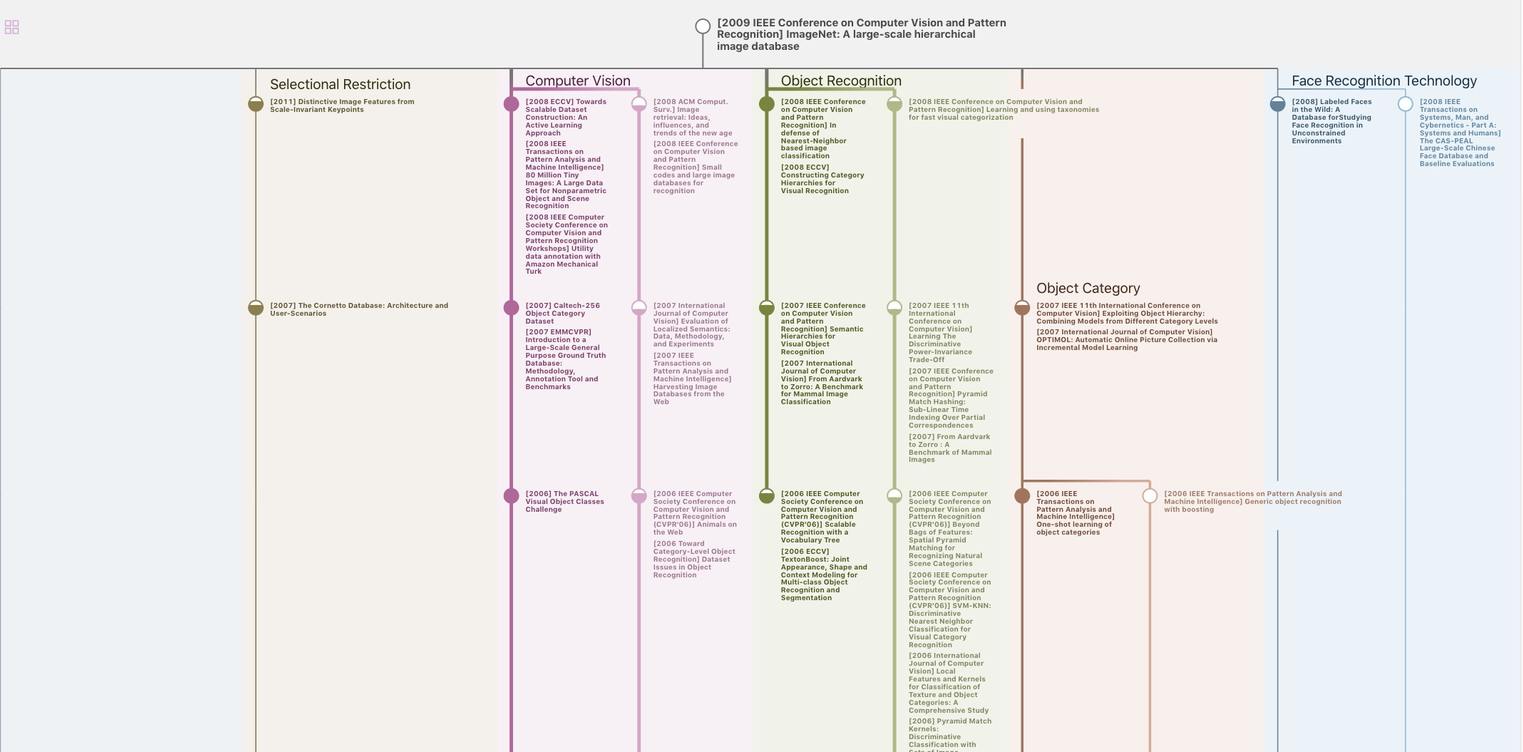
生成溯源树,研究论文发展脉络
Chat Paper
正在生成论文摘要