Intra- and Inter-group Optimal Transport for User-Oriented Fairness in Recommender Systems
AAAI 2024(2024)
摘要
Recommender systems are typically biased toward a small group of users, leading to severe unfairness in recommendation performance, i.e., User-Oriented Fairness (UOF) issue. Existing research on UOF exhibits notable limitations in two phases of recommendation models. In the training phase, current methods fail to tackle the root cause of the UOF issue, which lies in the unfair training process between advantaged and disadvantaged users. In the evaluation phase, the current UOF metric lacks the ability to comprehensively evaluate varying cases of unfairness. In this paper, we aim to address the aforementioned limitations and ensure recommendation models treat user groups of varying activity levels equally. In the training phase, we propose a novel Intra- and Inter-GrOup Optimal Transport framework (II-GOOT) to alleviate the data sparsity problem for disadvantaged users and narrow the training gap between advantaged and disadvantaged users. In the evaluation phase, we introduce a novel metric called ?-UOF, which enables the identification and assessment of various cases of UOF. This helps prevent recommendation models from leading to unfavorable fairness outcomes, where both advantaged and disadvantaged users experience subpar recommendation performance. We conduct extensive experiments on three real-world datasets based on four backbone recommendation models to prove the effectiveness of ?-UOF and the efficiency of our proposed II-GOOT.
更多查看译文
关键词
DMKM: Conversational Systems for Recommendation & Retrieval,PEAI: Bias, Fairness & Equity
AI 理解论文
溯源树
样例
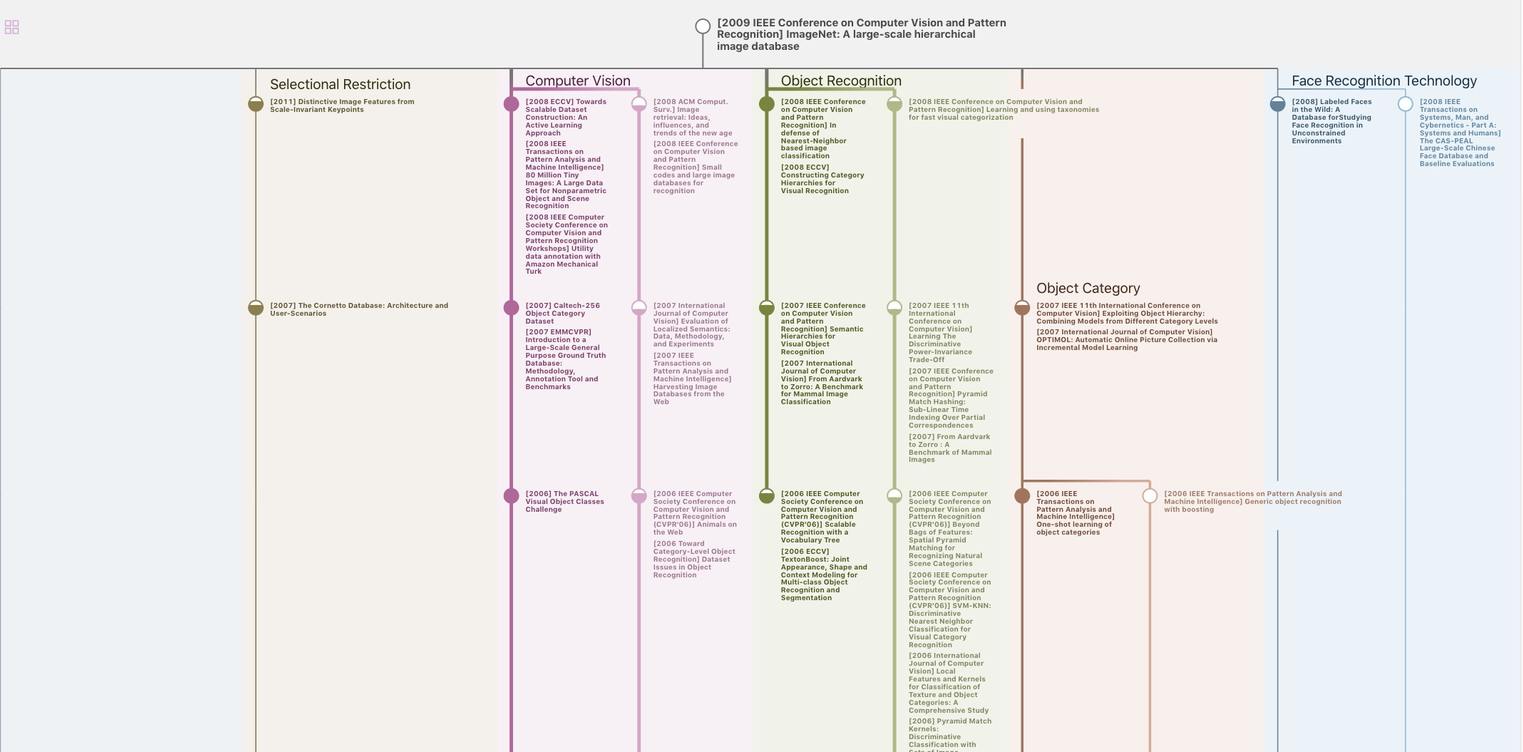
生成溯源树,研究论文发展脉络
Chat Paper
正在生成论文摘要