ULTRA: Machine Learning Optimized TRA For Enhanced Resource Allocation in MCF-based SDM-EONs.
International Conference on Advanced Networks and Telecommuncations Systems(2023)
摘要
The Tridental Resource Assignment algorithm (TRA) has been shown to effectively assign resources by balancing the tradeoff between spectrum utilization and intercore crosstalk in multicore fiber networks. It calculates the Tridental Coefficient (TC) to capture the tradeoff. The TC is calculated for all the resource choices to establish a lightpath, which is computationally expensive. In this paper, we show that by using machine learning enabled tuning of weights in TC, we can improve the performance of TRA. We also show that we can achieve an acceptable performance of TRA while decreasing the computational overhead with the optimized weights. We observe that bandwidth blocking of already better-performing TRA reduces by up to ≈3 orders of magnitude with the help of machine learning tuned weights. We also observe that acceptable performance of TRA can be achieved with as low as 45% of the total computations with optimized weights.
更多查看译文
关键词
Machine Learning,Optimal Weight,Unlicensed Spectrum,Data Rate,Machine Learning Models,Ergogenic,Exponential Distribution,Loss Of Capacity,Convex Optimization,Terahertz,Resource Selection,Average Execution Time,Modulation Formats,Percentage Of Choices
AI 理解论文
溯源树
样例
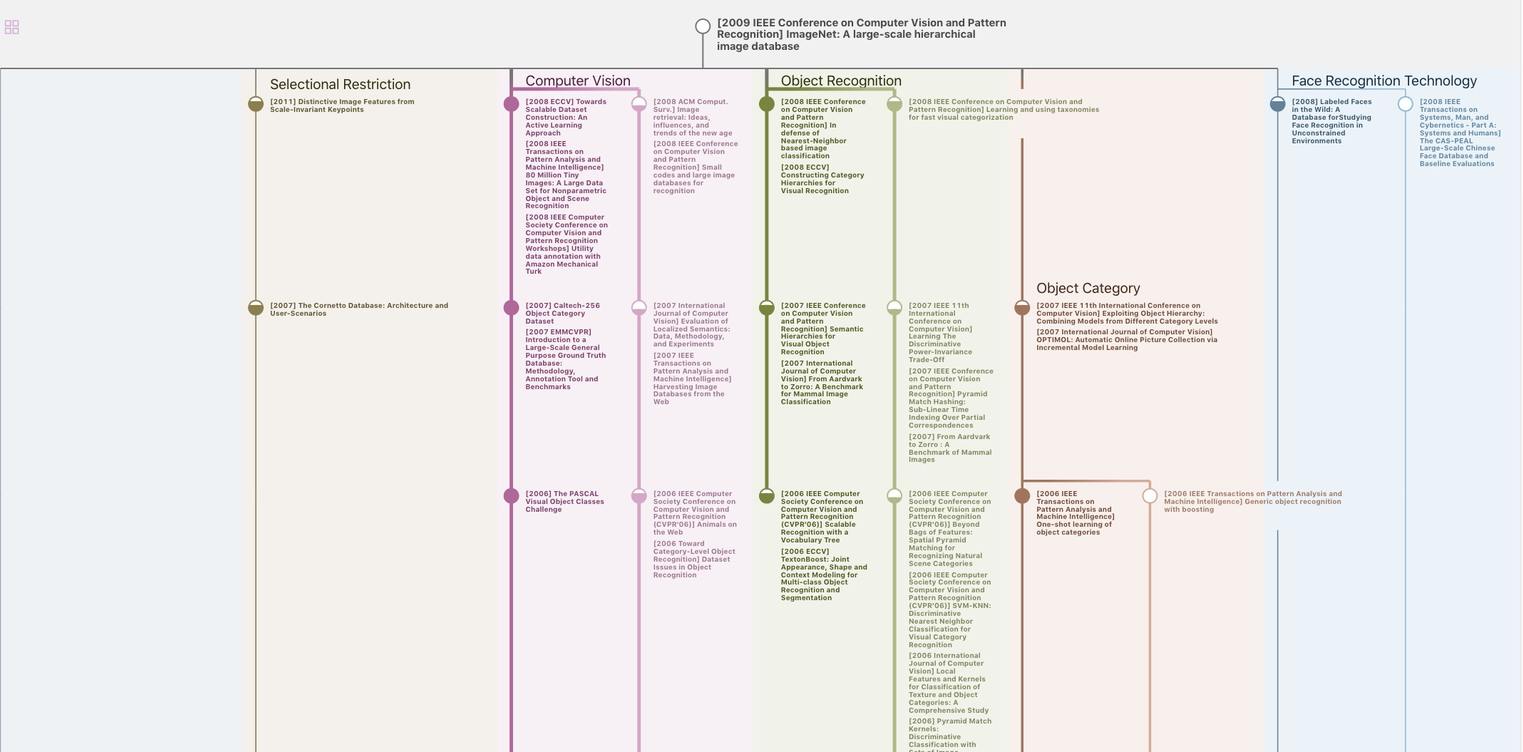
生成溯源树,研究论文发展脉络
Chat Paper
正在生成论文摘要