Image Captioning with Multi-Context Synthetic Data
AAAI 2024(2024)
摘要
Image captioning requires numerous annotated image-text pairs, resulting in substantial annotation costs. Recently, large models (e.g. diffusion models and large language models) have excelled in producing high-quality images and text. This potential can be harnessed to create synthetic image-text pairs for training captioning models. Synthetic data can improve cost and time efficiency in data collection, allow for customization to specific domains, bootstrap generalization capability for zero-shot performance, and circumvent privacy concerns associated with real-world data. However, existing methods struggle to attain satisfactory performance solely through synthetic data. We identify the issue as generated images from simple descriptions mostly capture a solitary perspective with limited context, failing to align with the intricate scenes prevalent in real-world imagery. To tackle this, we present an innovative pipeline that introduces multi-context data generation. Beginning with an initial text corpus, our approach employs a large language model to extract multiple sentences portraying the same scene from diverse viewpoints. These sentences are then condensed into a single sentence with multiple contexts. Subsequently, we generate intricate images using the condensed captions through diffusion models. Our model is exclusively trained on synthetic image-text pairs crafted through this process. The effectiveness of our pipeline is validated through experimental results in both the in-domain and cross-domain settings, where it achieves state-of-the-art performance on well-known datasets such as MSCOCO, Flickr30k, and NoCaps.
更多查看译文
关键词
CV: Language and Vision,CV: Multi-modal Vision
AI 理解论文
溯源树
样例
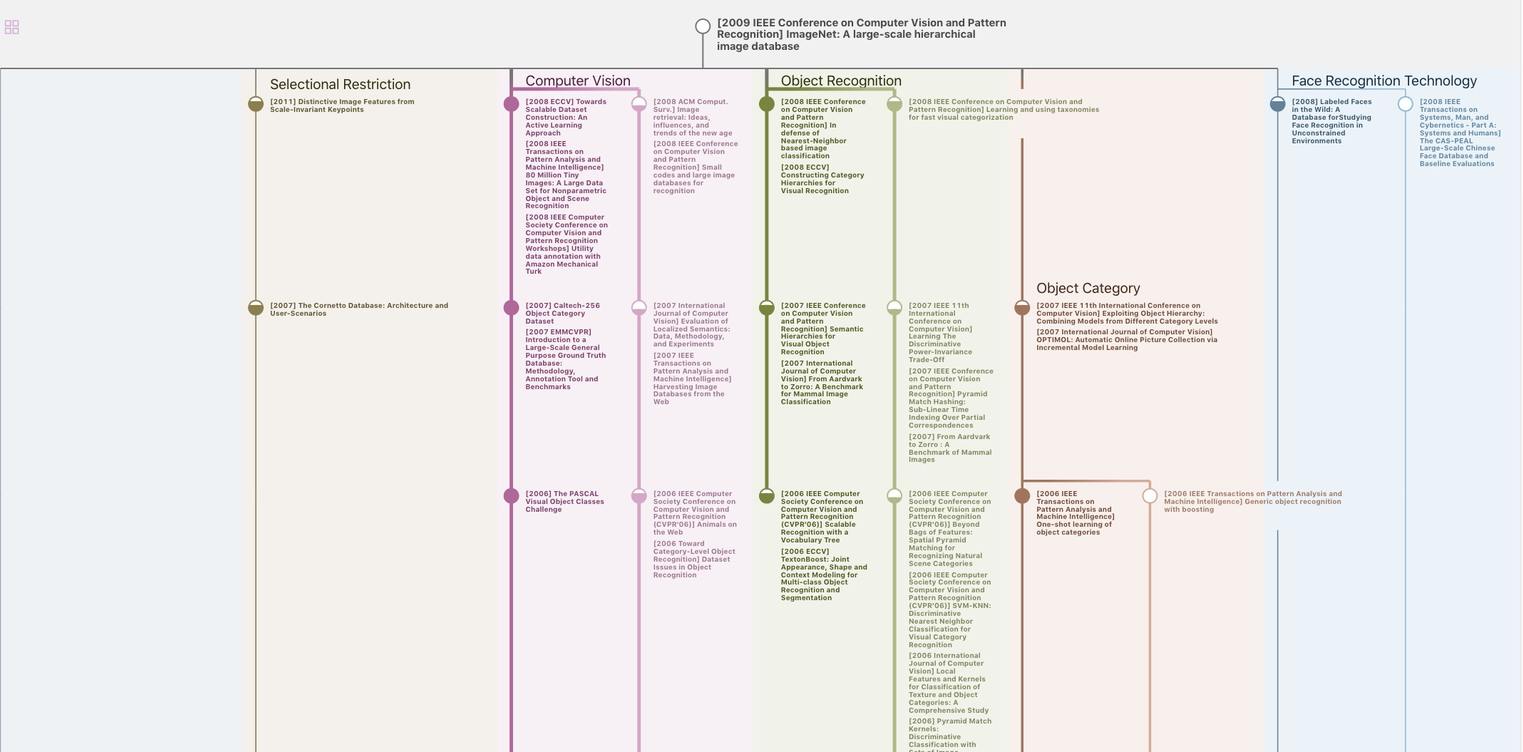
生成溯源树,研究论文发展脉络
Chat Paper
正在生成论文摘要