Quantitative modelling of type Ia supernovae spectral time series: Constraining the explosion physics
arxiv(2024)
摘要
Multiple explosion mechanisms have been proposed to explain type Ia
supernovae (SNe Ia). Through forward modelling, synthetic observables of
explosion models can be directly compared to observations of SNe Ia to
constrain the explosion physics. Due to the computational expense associated
with multi-dimensional explosion and radiative transfer simulations however,
empirical modelling tools have also been developed that allow for fast,
customised modelling of individual SNe. Such tools have provided useful
insights, but the subjective nature with which empirical modelling is performed
makes it difficult to obtain robust constraints on the explosion physics or
expand studies to large populations of objects. Machine learning accelerated
tools have therefore begun to gain traction. In this paper, we present riddler,
a framework for automated fitting of SNe Ia spectral sequences up to shortly
after maximum light. We train a series of neural networks on realistic ejecta
profiles predicted by the W7 and N100 explosion models to emulate full
radiative transfer simulations and apply nested sampling to determine the
best-fitting model parameters for multiple spectra of a given SN
simultaneously. We show that riddler is able to accurately recover the
parameters of input spectra and use it to fit observations of two well-studied
SNe Ia. We also investigate the impact of different weighting schemes when
performing quantitative spectral fitting. As spectroscopic samples of SNe Ia
continue to grow, automated spectral fitting tools such as riddler will become
increasingly important to maximise the physical constraints that can be gained
in a quantitative and consistent manner.
更多查看译文
AI 理解论文
溯源树
样例
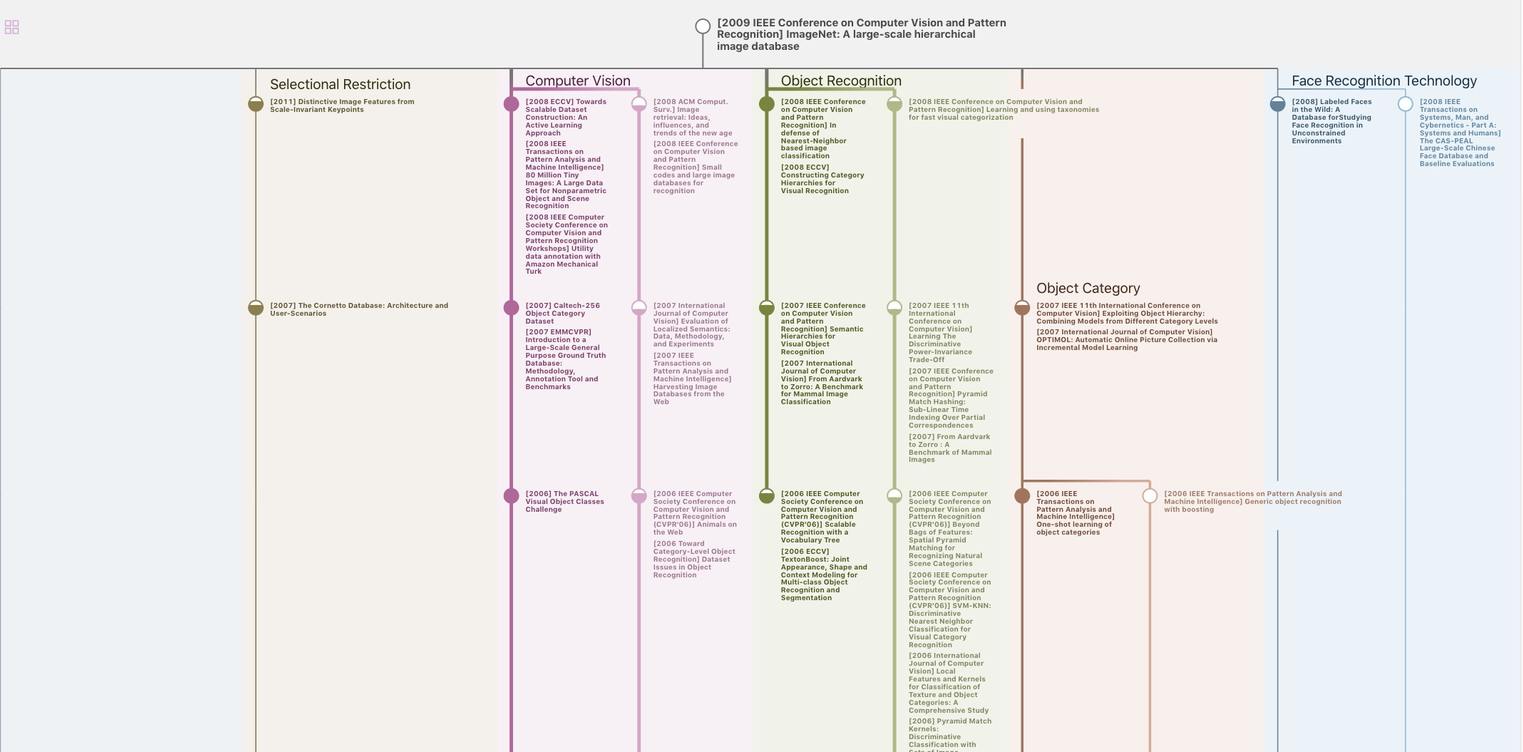
生成溯源树,研究论文发展脉络
Chat Paper
正在生成论文摘要