Anderson Acceleration Without Restart: A Novel Method with n-Step Super Quadratic Convergence Rate
arxiv(2024)
摘要
In this paper, we propose a novel Anderson's acceleration method to solve
nonlinear equations, which does not require a restart strategy to
achieve numerical stability. We propose the greedy and random versions of our
algorithm. Specifically, the greedy version selects the direction to maximize a
certain measure of progress for approximating the current Jacobian matrix. In
contrast, the random version chooses the random Gaussian vector as the
direction to update the approximate Jacobian. Furthermore, our algorithm,
including both greedy and random versions, has an n-step super quadratic
convergence rate, where n is the dimension of the objective problem. For
example, the explicit convergence rate of the random version can be presented
as _k+n+1 - _* / _k- _*^2 =
((1-1/n)^kn) for any k≥ 0 where
_* is the optimum of the objective problem. This kind of convergence rate
is new to Anderson's acceleration and quasi-Newton methods. The experiments
also validate the fast convergence rate of our algorithm.
更多查看译文
AI 理解论文
溯源树
样例
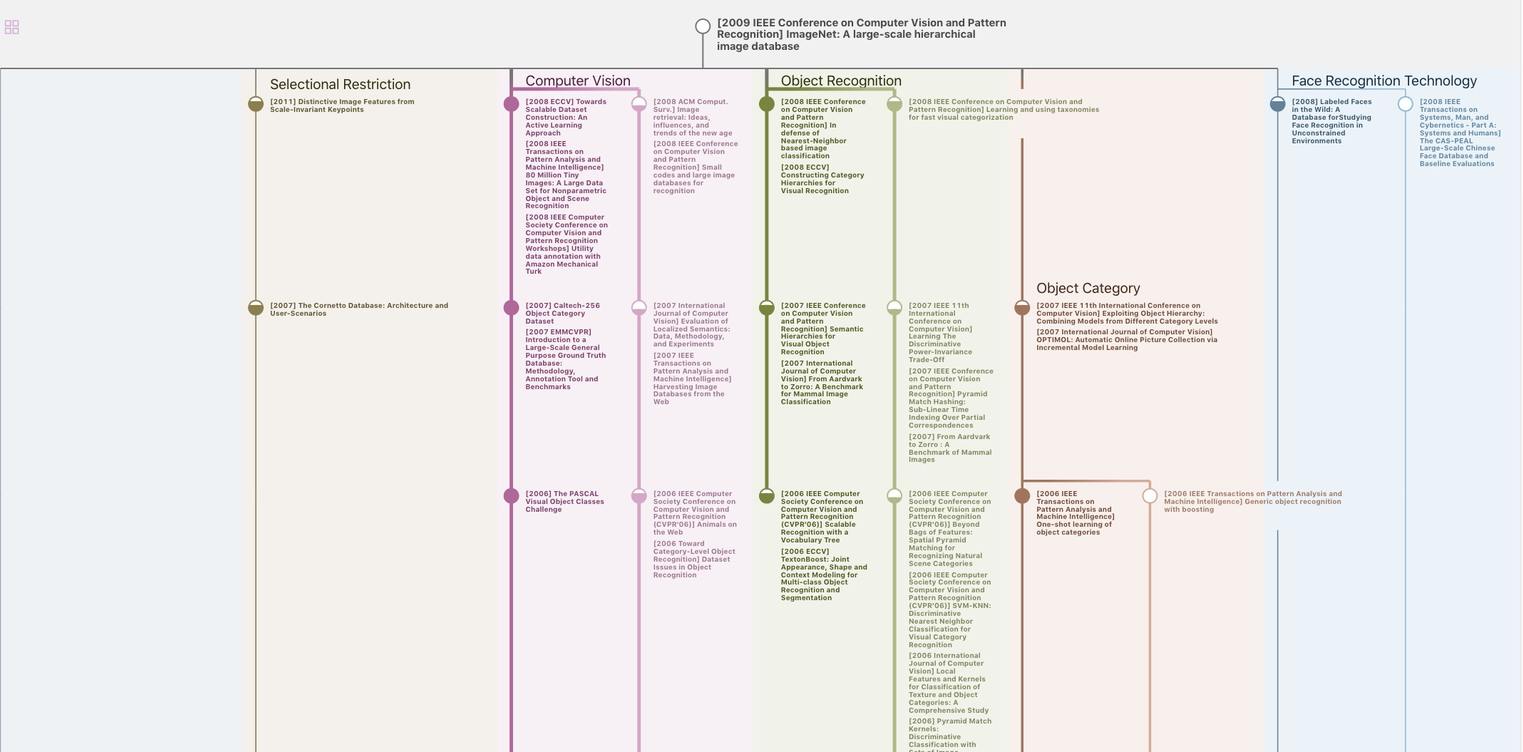
生成溯源树,研究论文发展脉络
Chat Paper
正在生成论文摘要