Data-Driven Extrusion Force Control Tuning for 3D Printing
arxiv(2024)
摘要
The quality of 3D prints often varies due to different conditions inherent to
each print, such as filament type, print speed, and nozzle size. Closed-loop
process control methods improve the accuracy and repeatability of 3D prints.
However, optimal tuning of controllers for given process parameters and design
geometry is often a challenge with manually tuned controllers resulting in
inconsistent and suboptimal results. This work employs Bayesian optimization to
identify the optimal controller parameters. Additionally, we explore transfer
learning in the context of 3D printing by leveraging prior information from
past trials. By integrating optimized extrusion force control and transfer
learning, we provide a novel framework for closed-loop 3D printing and propose
an automated calibration routine that produces high-quality prints for a
desired combination of print settings, material, and shape.
更多查看译文
AI 理解论文
溯源树
样例
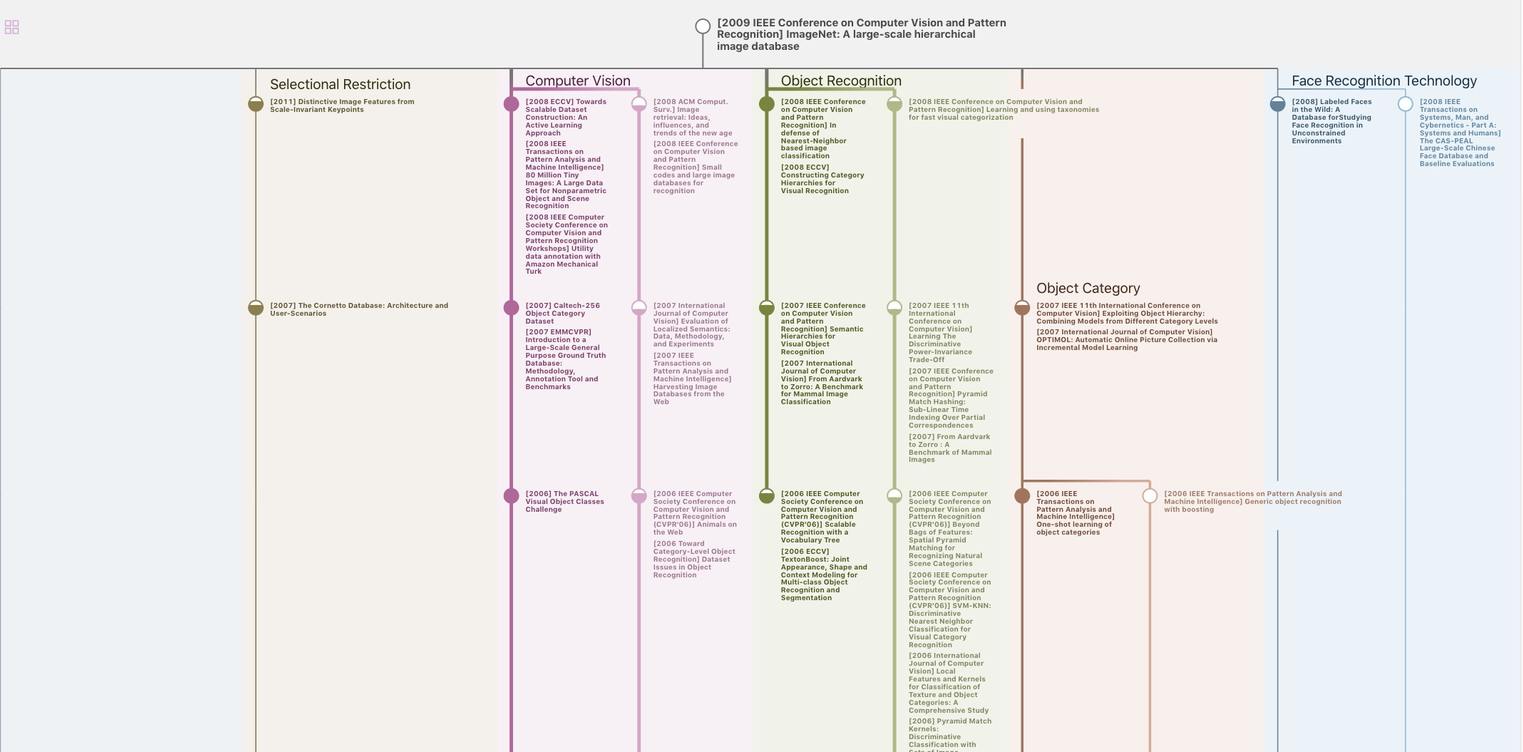
生成溯源树,研究论文发展脉络
Chat Paper
正在生成论文摘要