Benchmarks and Challenges in Pose Estimation for Egocentric Hand Interactions with Objects
arxiv(2024)
摘要
We interact with the world with our hands and see it through our own
(egocentric) perspective. A holistic 3D understanding of such interactions from
egocentric views is important for tasks in robotics, AR/VR, action recognition
and motion generation. Accurately reconstructing such interactions in 3D is
challenging due to heavy occlusion, viewpoint bias, camera distortion, and
motion blur from the head movement. To this end, we designed the HANDS23
challenge based on the AssemblyHands and ARCTIC datasets with carefully
designed training and testing splits. Based on the results of the top submitted
methods and more recent baselines on the leaderboards, we perform a thorough
analysis on 3D hand(-object) reconstruction tasks. Our analysis demonstrates
the effectiveness of addressing distortion specific to egocentric cameras,
adopting high-capacity transformers to learn complex hand-object interactions,
and fusing predictions from different views. Our study further reveals
challenging scenarios intractable with state-of-the-art methods, such as fast
hand motion, object reconstruction from narrow egocentric views, and close
contact between two hands and objects. Our efforts will enrich the community's
knowledge foundation and facilitate future hand studies on egocentric
hand-object interactions.
更多查看译文
AI 理解论文
溯源树
样例
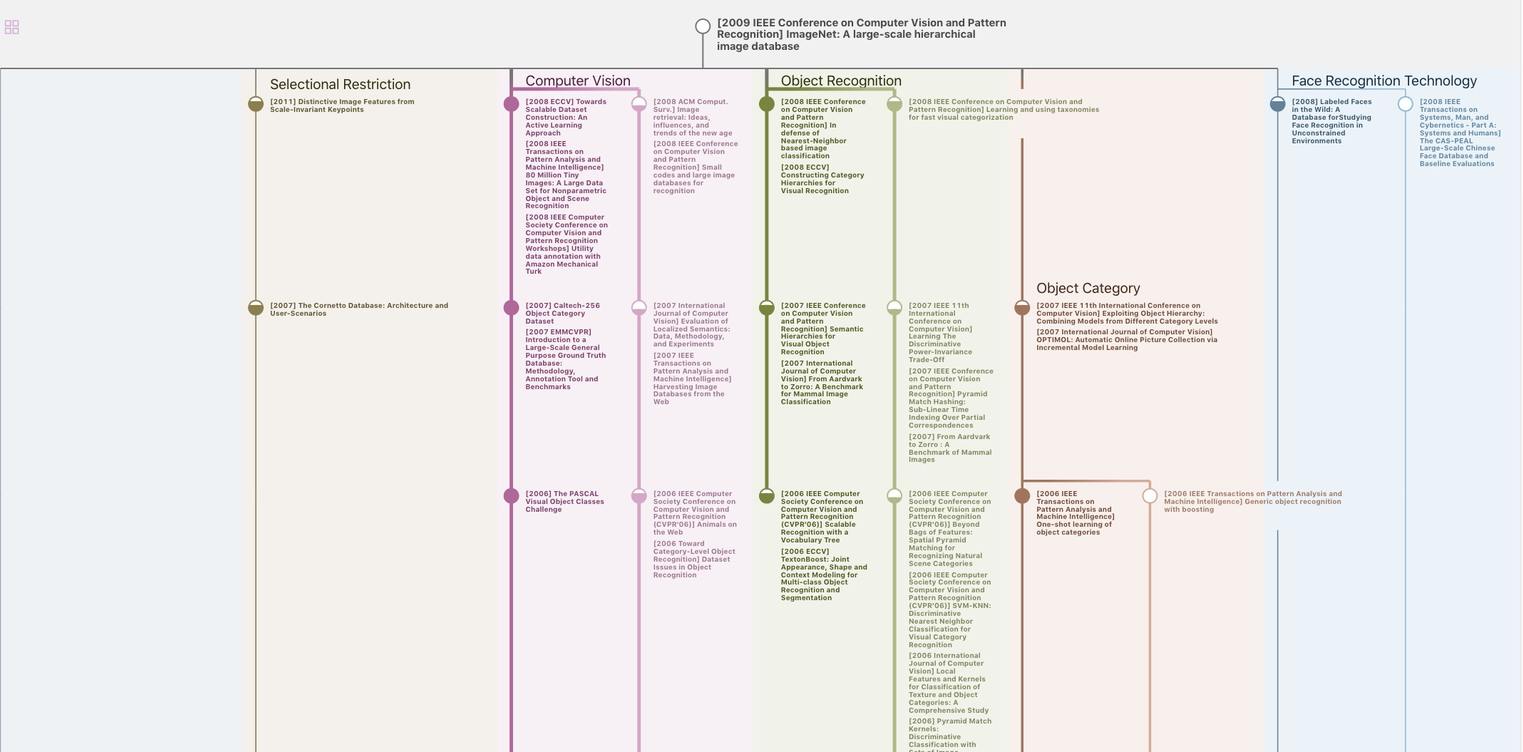
生成溯源树,研究论文发展脉络
Chat Paper
正在生成论文摘要