Q-adaptive: A Multi-Agent Reinforcement Learning Based Routing on Dragonfly Network
arxiv(2024)
摘要
on adaptive routing to balance network traffic for optimum performance.
Ideally, adaptive routing attempts to forward packets between minimal and
non-minimal paths with the least congestion. In practice, current adaptive
routing algorithms estimate routing path congestion based on local information
such as output queue occupancy. Using local information to estimate global path
congestion is inevitably inaccurate because a router has no precise knowledge
of link states a few hops away. This inaccuracy could lead to interconnect
congestion. In this study, we present Q-adaptive routing, a multi-agent
reinforcement learning routing scheme for Dragonfly systems. Q-adaptive routing
enables routers to learn to route autonomously by leveraging advanced
reinforcement learning technology. The proposed Q-adaptive routing is highly
scalable thanks to its fully distributed nature without using any shared
information between routers. Furthermore, a new two-level Q-table is designed
for Q-adaptive to make it computational lightly and saves 50
usage compared with the previous Q-routing. We implement the proposed
Q-adaptive routing in SST/Merlin simulator. Our evaluation results show that
Q-adaptive routing achieves up to 10.5
average packet latency reduction compared with adaptive routing algorithms.
Remarkably, Q-adaptive can even outperform the optimal VALn non-minimal routing
under the ADV+1 adversarial traffic pattern with up to 3
improvement and 75
更多查看译文
AI 理解论文
溯源树
样例
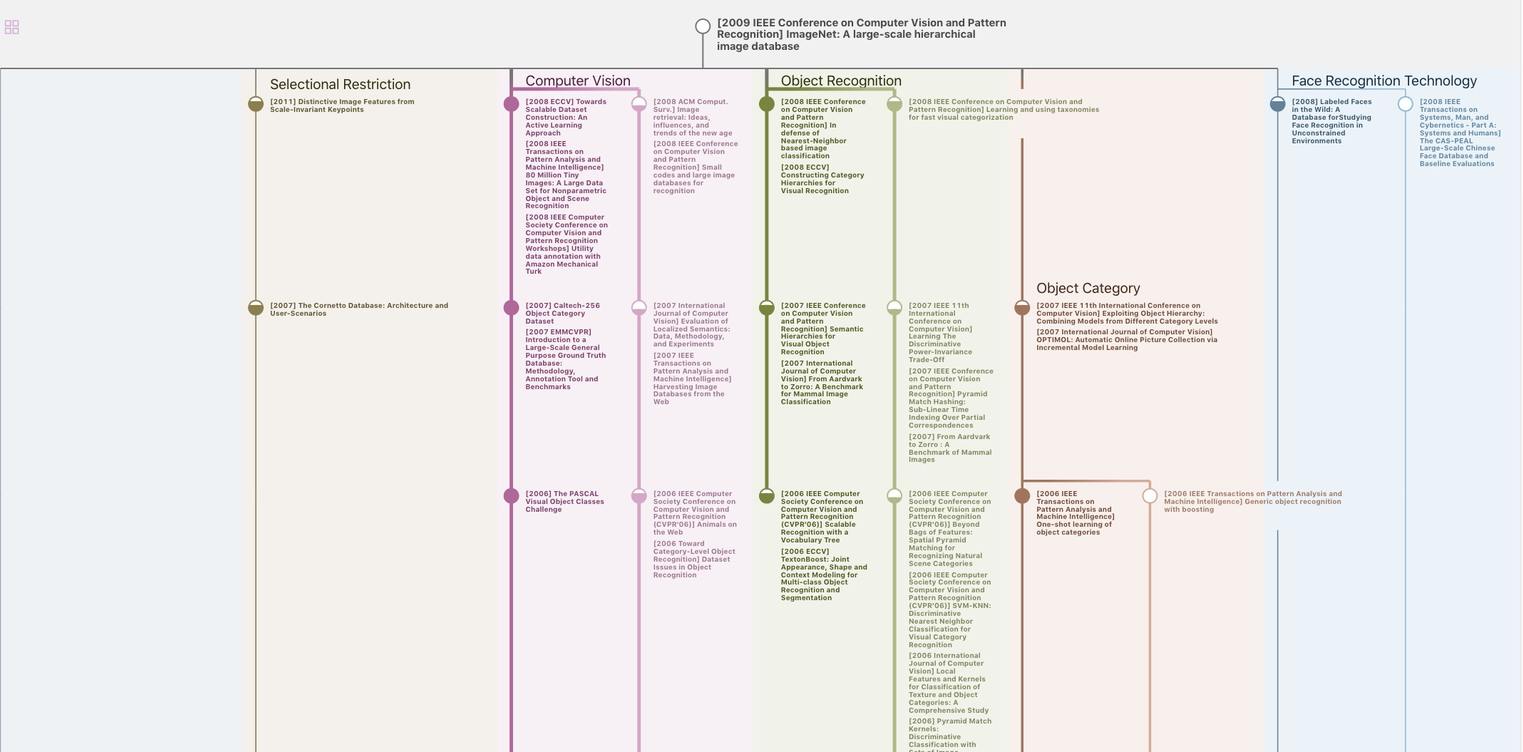
生成溯源树,研究论文发展脉络
Chat Paper
正在生成论文摘要