3D-TransUNet for Brain Metastases Segmentation in the BraTS2023 Challenge
arxiv(2024)
摘要
Segmenting brain tumors is complex due to their diverse appearances and
scales. Brain metastases, the most common type of brain tumor, are a frequent
complication of cancer. Therefore, an effective segmentation model for brain
metastases must adeptly capture local intricacies to delineate small tumor
regions while also integrating global context to understand broader scan
features. The TransUNet model, which combines Transformer self-attention with
U-Net's localized information, emerges as a promising solution for this task.
In this report, we address brain metastases segmentation by training the
3D-TransUNet model on the Brain Tumor Segmentation (BraTS-METS) 2023 challenge
dataset. Specifically, we explored two architectural configurations: the
Encoder-only 3D-TransUNet, employing Transformers solely in the encoder, and
the Decoder-only 3D-TransUNet, utilizing Transformers exclusively in the
decoder. For Encoder-only 3D-TransUNet, we note that Masked-Autoencoder
pre-training is required for a better initialization of the Transformer Encoder
and thus accelerates the training process. We identify that the Decoder-only
3D-TransUNet model should offer enhanced efficacy in the segmentation of brain
metastases, as indicated by our 5-fold cross-validation on the training set.
However, our use of the Encoder-only 3D-TransUNet model already yield notable
results, with an average lesion-wise Dice score of 59.8% on the test set,
securing second place in the BraTS-METS 2023 challenge.
更多查看译文
AI 理解论文
溯源树
样例
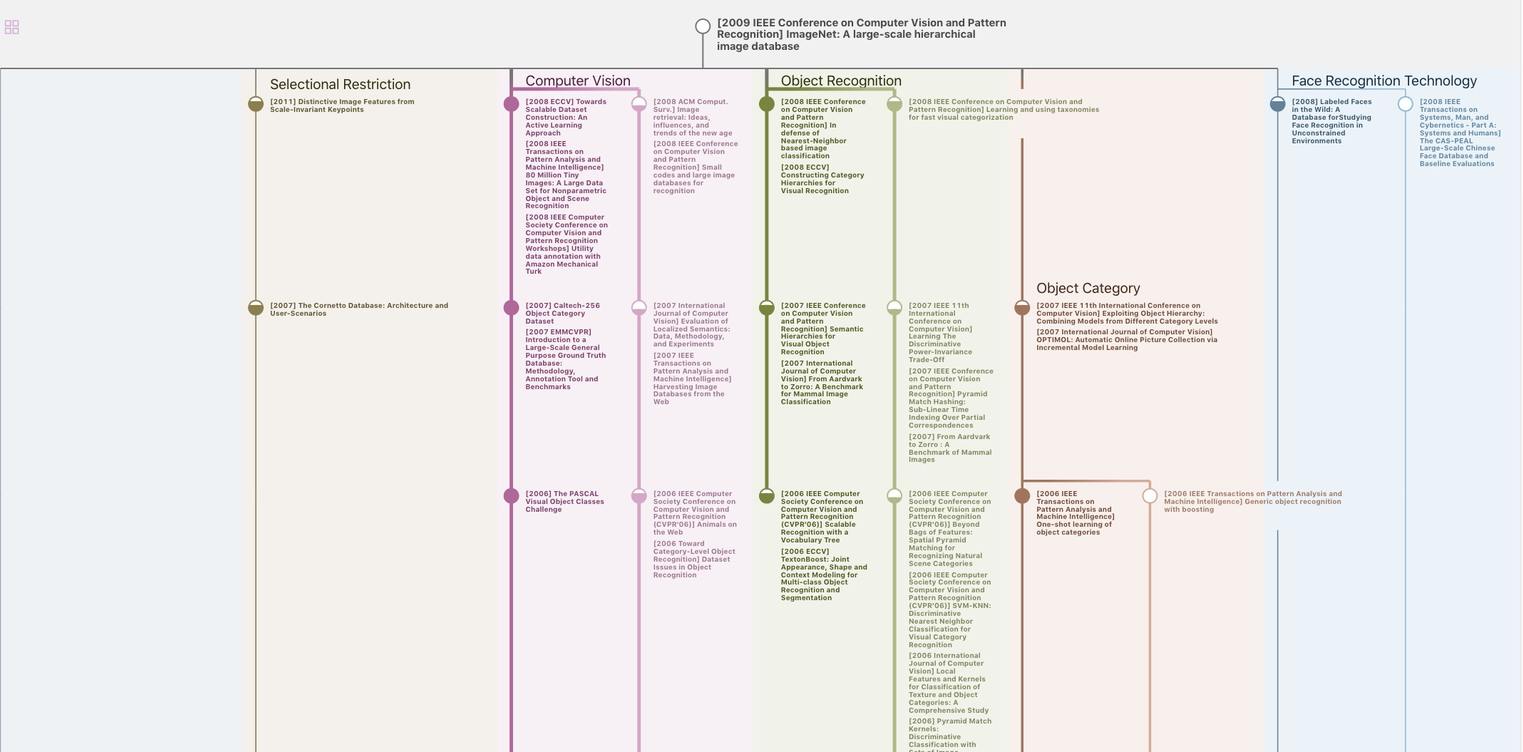
生成溯源树,研究论文发展脉络
Chat Paper
正在生成论文摘要