A general energy modeling network for serial industrial robots integrating physical mechanism priors
Robotics and Computer-Integrated Manufacturing(2024)
摘要
Industrial robots (IRs), as the core equipment of intelligent manufacturing, play increasingly important roles in various industrial scenarios such as assembly, welding, handling, and spraying, significantly improving production efficiency and product quality. The massive popularization and application of IRs have brought about a sharp increase in energy consumption (EC), and the modeling and optimization of EC is becoming imperative. In this paper, a general energy modeling network DM-PLM for serial IRs based on Dynamic Model (DM) and Power Loss Model (PLM) is proposed by integrating the prior knowledge of IR power composition and dynamic mechanism, enabling efficient and accurate modeling of dynamics, power, and EC under multi-load conditions. Considering the force transmission characteristics of serial robots, this paper proposes an improved bidirectional recurrent neural network (BiRNN) to model the joint dynamics. Additionally, a power loss model based on the ResNet convolutional neural network is employed. Experiments are carried out with a KUKA KR210 heavy-duty robot and a UR5 collaborative robot. The results show that the DM-PLM model incorporating the physical mechanism priors achieves 97 %, 98 %, and 99 % modeling accuracy in joint torques, total power, and EC for both robots under multi-load conditions. In addition, the proposed DM-PLM model is applied to the EC optimization of KUKA KR210 through trajectory planning, which achieves over 30 % EC reduction with the genetic algorithm, providing an effective approach to improving the energy efficiency of serial IRs.
更多查看译文
关键词
Power modeling,Energy consumption modeling,Deep learning,Serial industrial robots,Physical mechanism priors
AI 理解论文
溯源树
样例
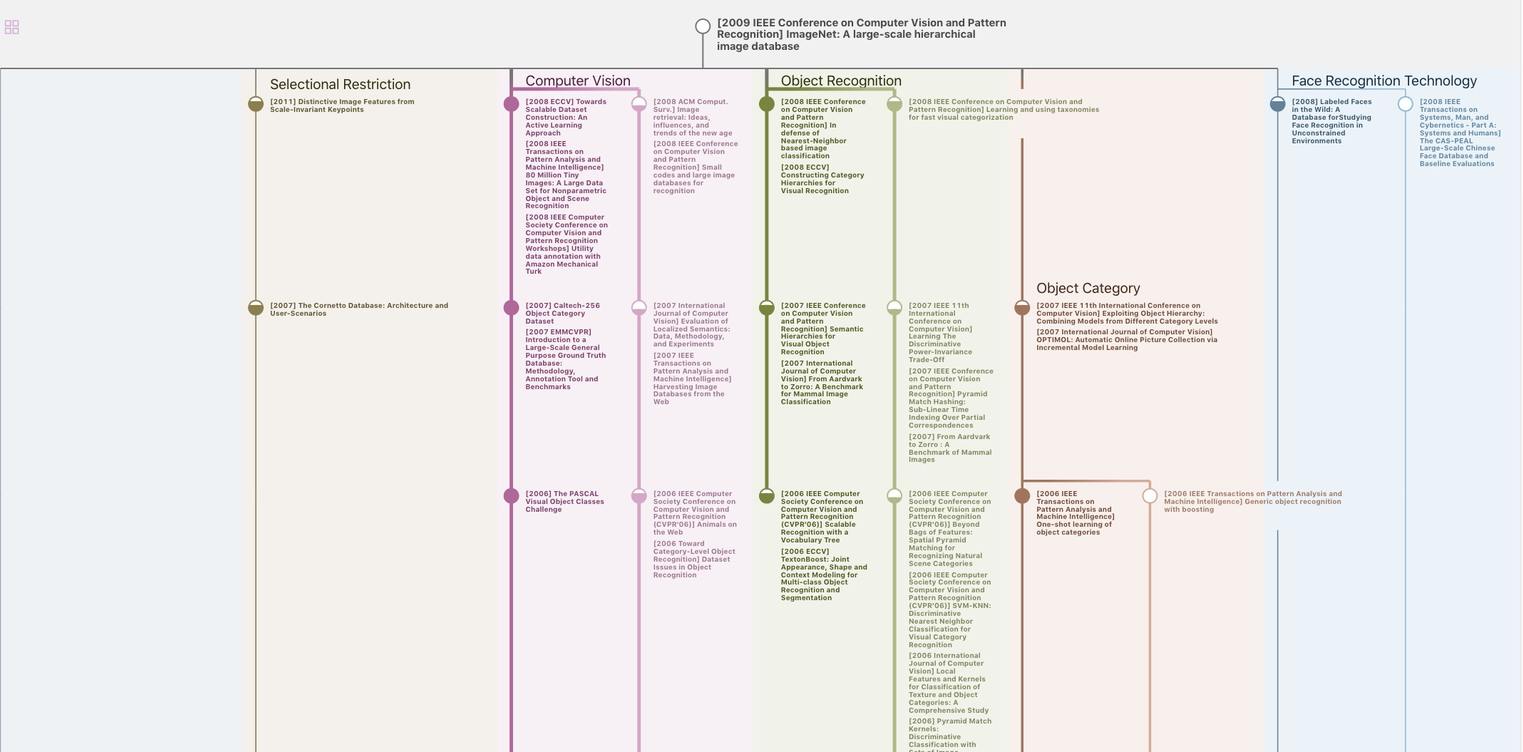
生成溯源树,研究论文发展脉络
Chat Paper
正在生成论文摘要