STFE-Net: A multi-stage approach to enhance statistical texture feature for defect detection on metal surfaces
Advanced Engineering Informatics(2024)
摘要
Statistical texture features are essential for metal surface defect detection. However, low contrast and cluttered backgrounds exacerbate the intrinsic blurriness and variability of defect textures, hampering accurate identification and localization. To tackle these challenges, we propose a Statistical Texture Feature Enhancement Network (STFE-Net) based on YOLO model, a multi-stage approach based on pooling attention mechanism and cross-scale shallow feature reinforcement. Firstly, we enhance the image contrast and propose a Stacked Pooling Attention Residual (SPA-Res) module to capture better texture features; then, we embed the Shallow Feature Weighted (SFW) branches to achieve cross-layer shallow feature reinforcement and add feature layer (AFL) to extract rich semantic features; and finally, we propose an Efficient Complete Intersection over Union (ECIoU) loss function and employ four detection heads to achieve precise localization of defects. On the NEU-DET dataset, our model achieves the state-of-the-art performance. Especially, the mean Average Precision (mAP@IoU = 0.5) of our model is 82.3 %, which is 6.2 % higher than baseline network. With the input resolution of 640 × 640, STFE-Net achieves 54 FPS inference speed with a GeForce GTX 1080. In addition, the experimental results on the DAGM dataset and GC10-DET dataset demonstrate that the proposed model has excellent generalization ability.
更多查看译文
关键词
Surface defect detection,Deep learning,Feature fusion,Attention mechanism,YOLO
AI 理解论文
溯源树
样例
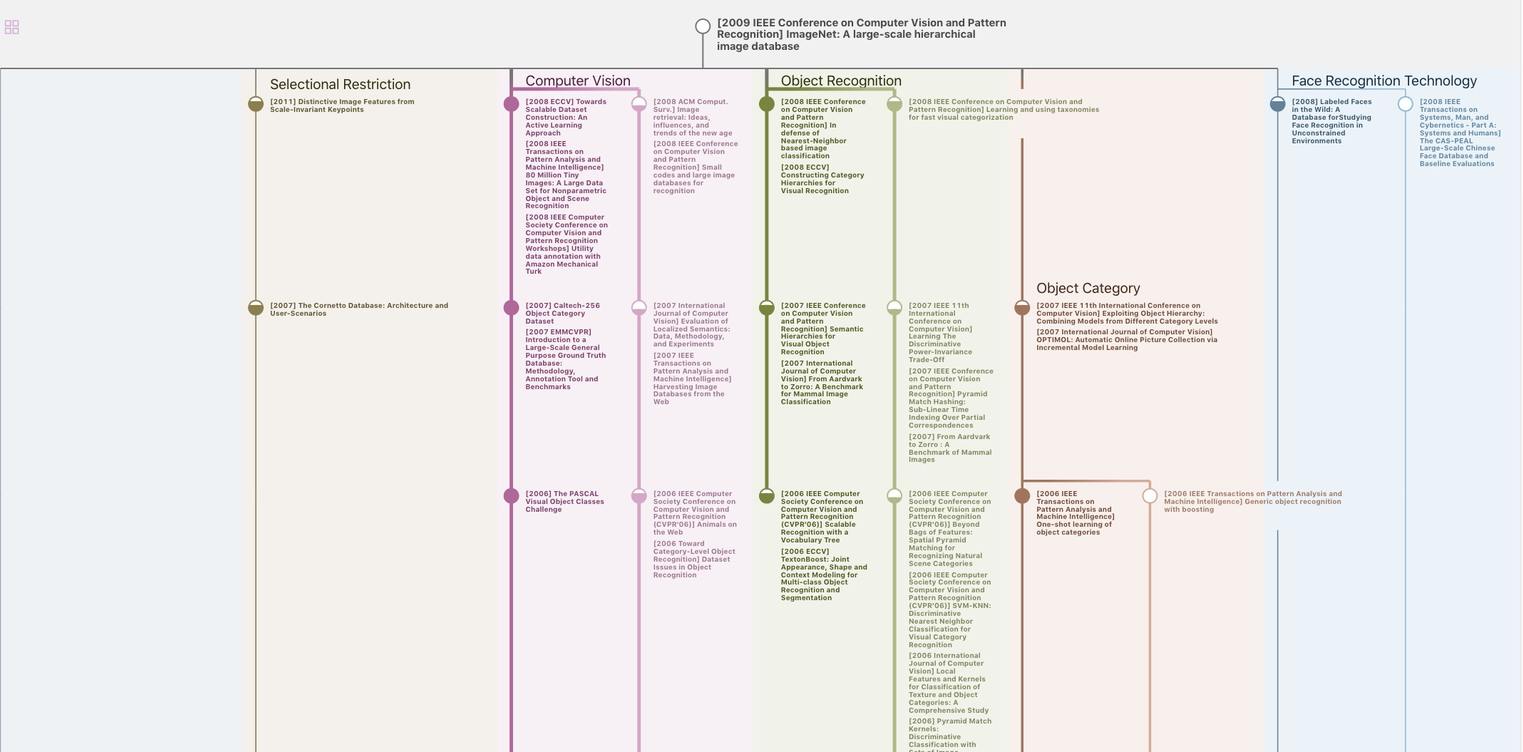
生成溯源树,研究论文发展脉络
Chat Paper
正在生成论文摘要