Improved sea surface salinity data for the Arctic Ocean derived from SMAP satellite data using machine learning approaches
FRONTIERS IN MARINE SCIENCE(2024)
摘要
Salinity is among the key climate characteristics of the World Ocean. During the last 15 years, sea surface salinity (SSS) is measured using satellite passive microwave sensors. Standard retrieving SSS algorithms from remote sensing data were developed and verified for the most typical temperature and salinity values of the World Ocean. However, they have far lower accuracy for the Arctic Ocean, especially its shelf areas, which are influenced by large river runoff and have low typical temperature and salinity values. In this study, an improved algorithm has been developed to retrieve SSS in the Arctic Ocean during ice-free season, based on Soil Moisture Active Passive (SMAP) mission data, and using machine learning approaches. Extensive database of in situ salinity measurements in the Russian Arctic seas collected during multiple field surveys is applied to train and validate the machine learning models. The error in SSS retrieval of the developed algorithm compared to the standard algorithm reduced from 3.15 to 2.15 psu, and the correlation with in situ data increased from 0.82 to 0.90. The obtained daily SSS fields are important to improve accurate assessment of spatial and temporal variability of large river plumes in the Arctic Ocean.
更多查看译文
关键词
sea surface salinity,machine learning,SMAP,river plume,Arctic Ocean
AI 理解论文
溯源树
样例
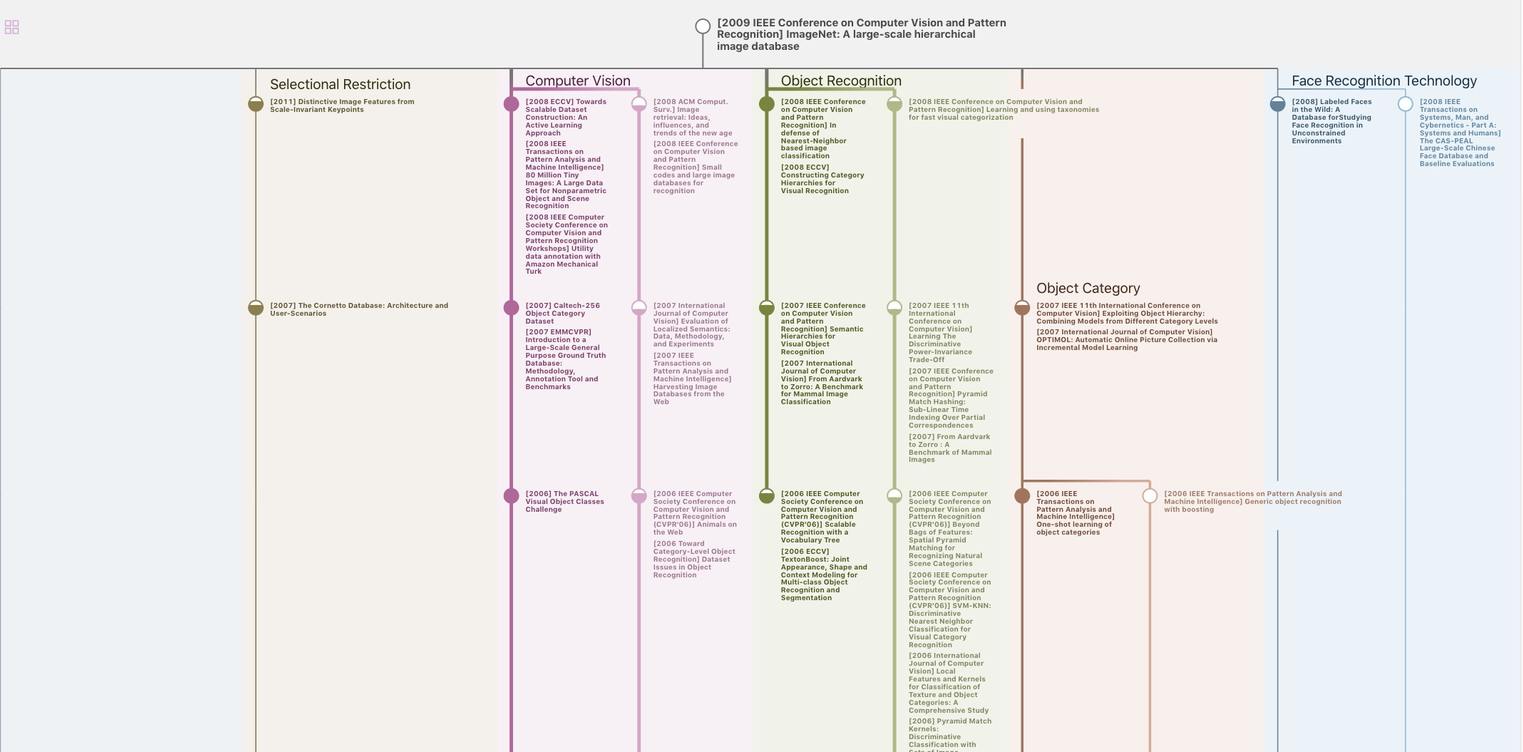
生成溯源树,研究论文发展脉络
Chat Paper
正在生成论文摘要