EigenGAN: An SVD subspace-based learning for image generation using Conditional GAN
Knowledge-Based Systems(2024)
摘要
Generative adversarial networks (GANs) represent a significant advance in the field of deep learning for image generation problems. With their ability to generate highly realistic and diverse images, GANs are quickly becoming the preferred technique for a wide range of applications. However, GANs come with several limitations and challenges, chief among which are their collapse mode and instability during training. In this paper, we propose a novel generator GAN model that incorporates the singular value decomposition (SVD) process into the decoder module. The SVD integration allows the generator to recognize the underlying structures in the feature space, resulting in more robust and effective image generation. This is achieved by adjusting the singular values during the training process, effectively optimizing the SVD-based generator to produce images that match the ground truth. Another advantage of SVD integration is its ability to perform discriminative spatial decomposition that clearly reflects the differences between the generated features and the target features. By including SVD in the generator model, the resulting loss values are higher and less prone to gradient vanishing than conventional GANs, such as Pix2Pix. Our proposed SVD-based generator model can be integrated into any auto-encoder architecture, making it as a generic and versatile solution for various image-generation tasks. The effectiveness of the proposed SVD-based GAN in image generation has been validated in three challenging benchmarks for image restoration and visible-to-infrared image translation. Extensive experiments demonstrate the significant quantitative and qualitative improvements achieved by our SVD-based GAN compared to baseline GAN architectures. The overall system outperforms the current state of the art in all benchmarks tested.
更多查看译文
关键词
GANs,Subspace learning,SVD decomposition,Image restoration,Singular values-aware decoding,Singular values equalization
AI 理解论文
溯源树
样例
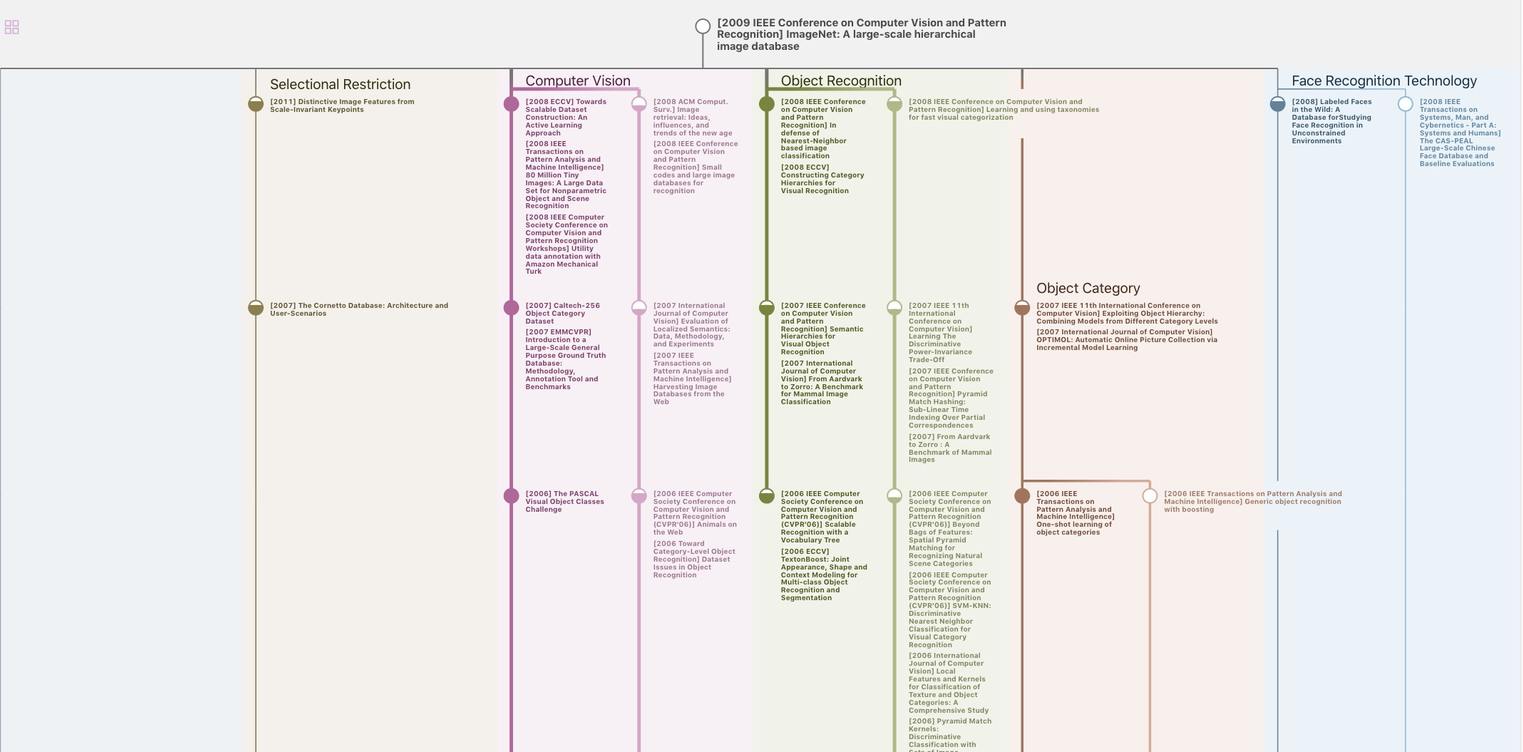
生成溯源树,研究论文发展脉络
Chat Paper
正在生成论文摘要