Rethinking 6-Dof Grasp Detection: A Flexible Framework for High-Quality Grasping
arxiv(2024)
摘要
Robotic grasping is a primitive skill for complex tasks and is fundamental to
intelligence. For general 6-Dof grasping, most previous methods directly
extract scene-level semantic or geometric information, while few of them
consider the suitability for various downstream applications, such as
target-oriented grasping. Addressing this issue, we rethink 6-Dof grasp
detection from a grasp-centric view and propose a versatile grasp framework
capable of handling both scene-level and target-oriented grasping. Our
framework, FlexLoG, is composed of a Flexible Guidance Module and a Local Grasp
Model. Specifically, the Flexible Guidance Module is compatible with both
global (e.g., grasp heatmap) and local (e.g., visual grounding) guidance,
enabling the generation of high-quality grasps across various tasks. The Local
Grasp Model focuses on object-agnostic regional points and predicts grasps
locally and intently. Experiment results reveal that our framework achieves
over 18
Furthermore, real-world robotic tests in three distinct settings yield a 95
success rate.
更多查看译文
AI 理解论文
溯源树
样例
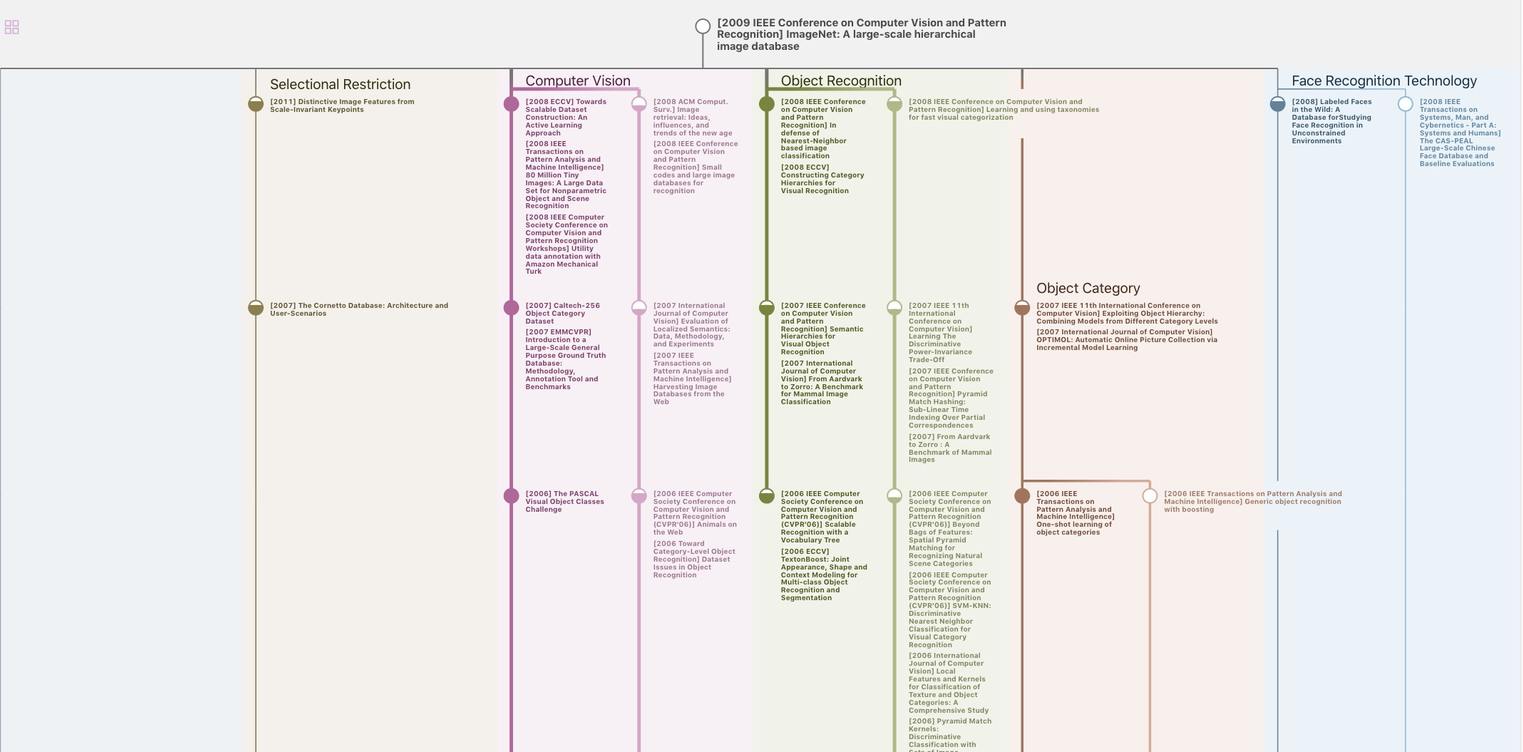
生成溯源树,研究论文发展脉络
Chat Paper
正在生成论文摘要