Trajectory Regularization Enhances Self-Supervised Geometric Representation
arxiv(2024)
摘要
Self-supervised learning (SSL) has proven effective in learning high-quality
representations for various downstream tasks, with a primary focus on semantic
tasks. However, its application in geometric tasks remains underexplored,
partially due to the absence of a standardized evaluation method for geometric
representations. To address this gap, we introduce a new pose-estimation
benchmark for assessing SSL geometric representations, which demands training
without semantic or pose labels and achieving proficiency in both semantic and
geometric downstream tasks. On this benchmark, we study enhancing SSL geometric
representations without sacrificing semantic classification accuracy. We find
that leveraging mid-layer representations improves pose-estimation performance
by 10-20
loss, which improves performance by an additional 4
generalization ability on out-of-distribution data. We hope the proposed
benchmark and methods offer new insights and improvements in self-supervised
geometric representation learning.
更多查看译文
AI 理解论文
溯源树
样例
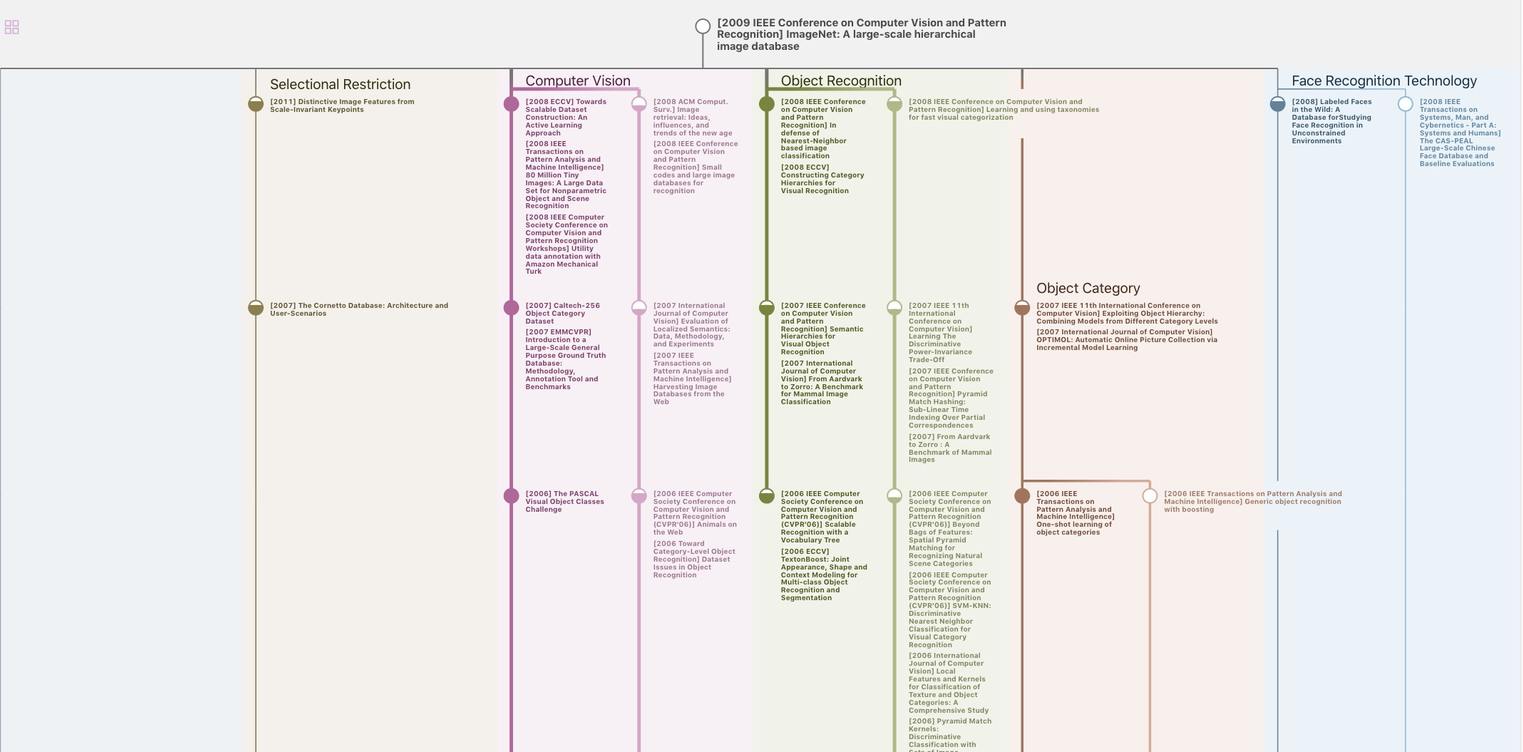
生成溯源树,研究论文发展脉络
Chat Paper
正在生成论文摘要