Automatic Scoring of Metaphor Creativity with Large Language Models
CREATIVITY RESEARCH JOURNAL(2024)
摘要
Metaphor is crucial in human cognition and creativity, facilitating abstract thinking, analogical reasoning, and idea generation. Typically, human raters manually score the originality of responses to creative thinking tasks - a laborious and error-prone process. Previous research sought to remedy these risks by scoring creativity tasks automatically using semantic distance and large language models (LLMs). Here, we extend research on automatic creativity scoring to metaphor generation - the ability to creatively describe episodes and concepts using nonliteral language. Metaphor is arguably more abstract and naturalistic than prior targets of automated creativity assessment. We collected 4,589 responses from 1,546 participants to various metaphor prompts and corresponding human creativity ratings. We fine-tuned two open-source LLMs (RoBERTa and GPT-2) - effectively "teaching" them to score metaphors like humans - before testing their ability to accurately assess the creativity of new metaphors. Results showed both models reliably predicted new human creativity ratings (RoBERTa r = .72, GPT-2 r = .70), significantly more strongly than semantic distance (r = .42). Importantly, the fine-tuned models generalized accurately to metaphor prompts they had not been trained on (RoBERTa r = .68, GPT-2 r = .63). We provide open access to the fine-tuned models, allowing researchers to assess metaphor creativity in a reproducible and timely manner.
更多查看译文
AI 理解论文
溯源树
样例
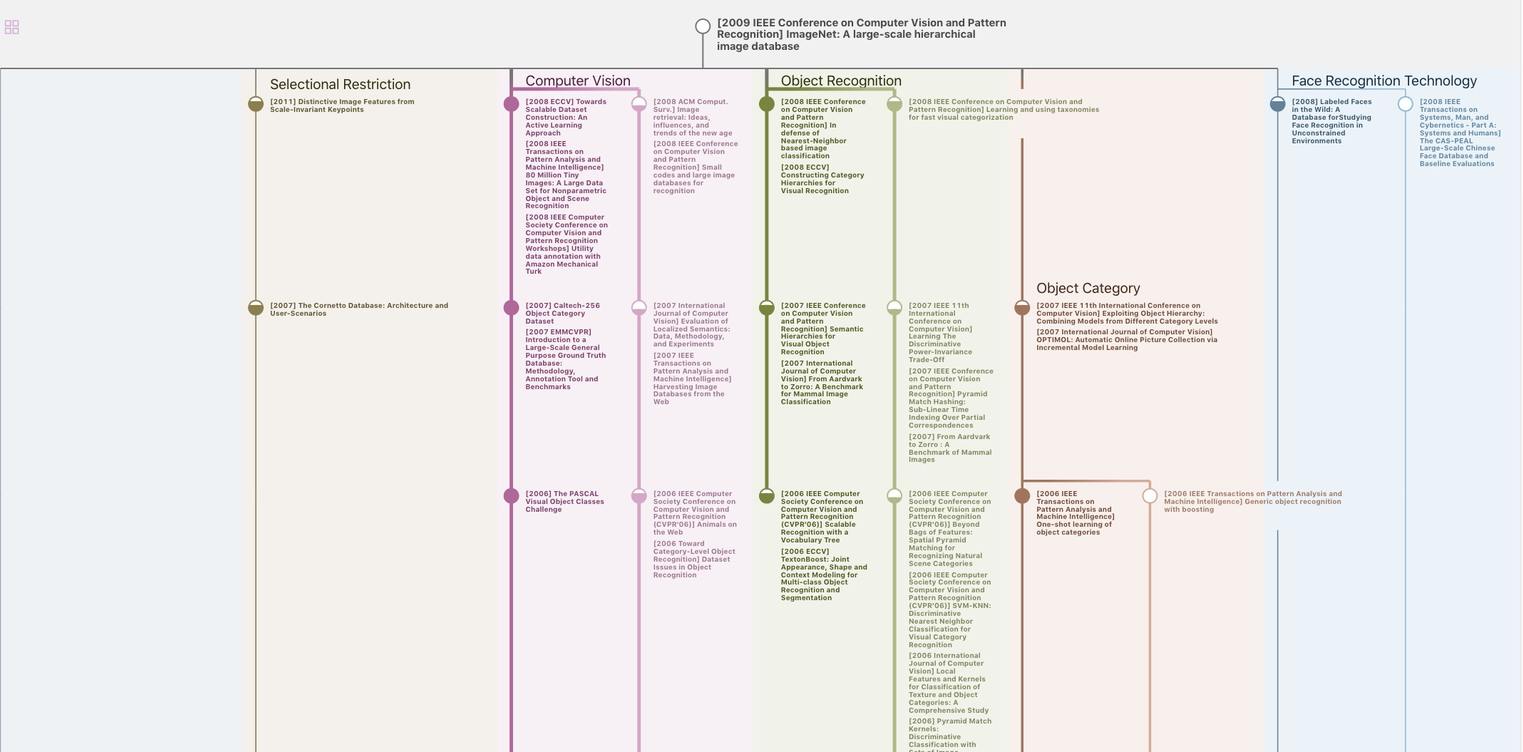
生成溯源树,研究论文发展脉络
Chat Paper
正在生成论文摘要