Assessing the Emergence and Evolution of Artificial Intelligence and Machine Learning Research in Neuroradiology.
AJNR. American journal of neuroradiology(2024)
摘要
BACKGROUND AND PURPOSE:Interest in artificial intelligence (AI) and machine learning (ML) has been growing in neuroradiology, but there is limited knowledge on how this interest has manifested into research and specifically, its qualities and characteristics. This study aims to characterize the emergence and evolution of AI/ML articles within neuroradiology and provide a comprehensive overview of the trends, challenges, and future directions of the field.
MATERIALS AND METHODS:We performed a bibliometric analysis of the American Journal of Neuroradiology (AJNR): the journal was queried for original research articles published since inception (Jan. 1, 1980) to Dec. 3, 2022 that contained any of the following key terms: "machine learning", "artificial intelligence", "radiomics", "deep learning", "neural network", "generative adversarial network", "object detection", or "natural language processing". Articles were screened by two independent reviewers, and categorized into Statistical Modelling (Type 1), AI/ML Development (Type 2), both representing developmental research work but without a direct clinical integration, or End-user Application (Type 3) which is the closest surrogate of potential AI/ML integration into day-to-day practice. To better understand the limiting factors to Type 3 articles being published, we analyzed Type 2 articles as they should represent the precursor work leading to Type 3.
RESULTS:A total of 182 articles were identified with 79% being non-integration focused (Type 1 n = 53, Type 2 n = 90) and 21% (n = 39) being Type 3. The total number of articles published grew roughly five-fold in the last five years, with the non-integration focused articles mainly driving this growth. Additionally, a minority of Type 2 articles addressed bias (22%) and explainability (16%). These articles were primarily led by radiologists (63%), with most of them (60%) having additional postgraduate degrees.
CONCLUSIONS:AI/ML publications have been rapidly increasing in neuroradiology with only a minority of this growth being attributable to end-user application. Areas identified for improvement include enhancing the quality of Type 2 articles, namely external validation, and addressing both bias and explainability. These results ultimately provide authors, editors, clinicians, and policymakers important insights to promote a shift towards integrating practical AI/ML solutions in neuroradiology.
ABBREVIATIONS:AI = artificial intelligence; ML = machine learning.
更多查看译文
AI 理解论文
溯源树
样例
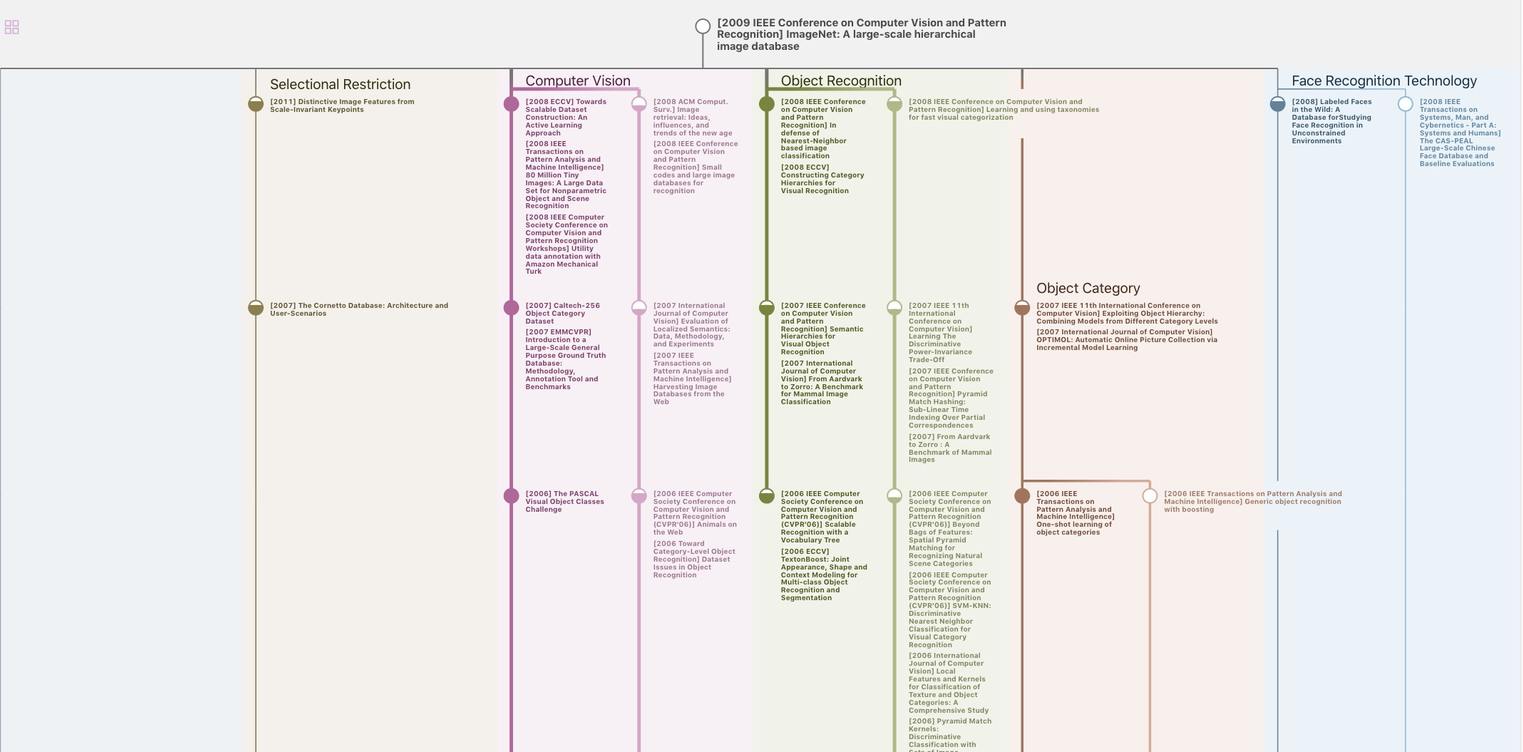
生成溯源树,研究论文发展脉络
Chat Paper
正在生成论文摘要