Investigation of Egg Quality Characteristics Affecting Egg Weight of Lohmann Brown Hen with Data Mining Methods
POULTRY SCIENCE JOURNAL(2024)
摘要
Hen egg weight is one of the most important traits in the egg production industry; however, the egg traits influencing it are poorly understood. Random forest (RF), multivariate adaptive regression spline (MARS), classification and regression trees (CART), bagging MARS, chi-square automatic interaction detector (CHAID), and exhaustive CHAID were used in egg weight (EW) prediction from selected egg quality characteristics in chicken. A total of 400 egg weight (EW), egg length (EL), egg width (EWD), shell weight (SW), yolk weight (YW), and albumen weight (AW) predictors were turned into account. The goodness-of-fit criteria were used to select the best model to estimate Lohman Brown hen egg weight. The data was separated into train and test datasets for validation using a 10 -fold cross-validation. The most significant EW predictors were albumen weight, egg width, and egg length. The correlation coefficient (r) value ranged from 0.957 (CHAID) to 0.99999 (MARS and Bagging MARS). The lowest RMSE (0.001) was found for MARS and bagging MARS algorithms and the highest (2.154) was obtained for CHAID. In general, the implemented algorithms excellently predicted the EW of hens. The ascertainment of the egg quality characteristics associated with EW using data mining algorithms can be considered an indirect selection criterion for further chicken breeding programs.
更多查看译文
关键词
Egg,Chicken,Random forest,MARS,Bagging MARS
AI 理解论文
溯源树
样例
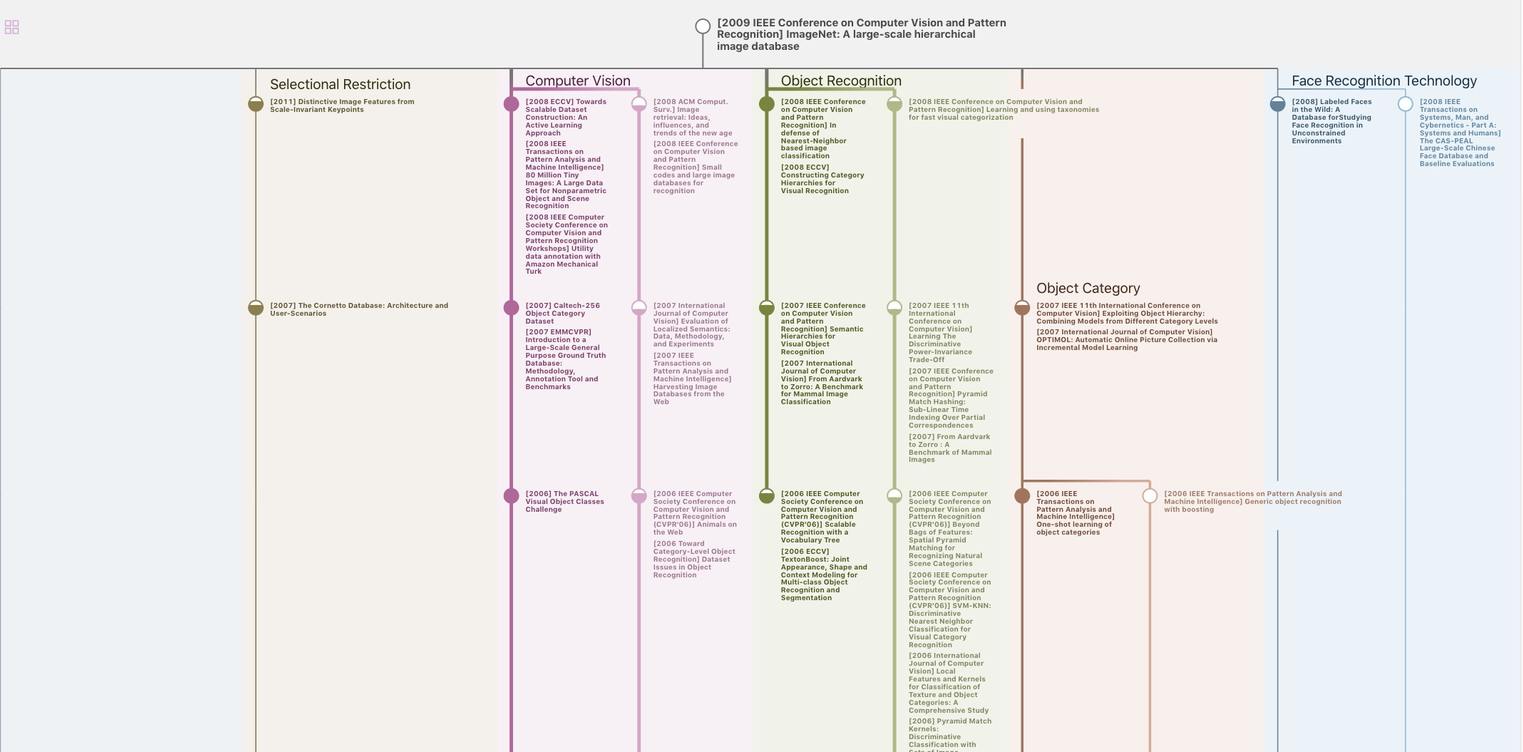
生成溯源树,研究论文发展脉络
Chat Paper
正在生成论文摘要