Abstract 2344: Federated image quality assessment of prostate MRI scans in a multi-institutional setting
Cancer Research(2024)
摘要
Abstract Introduction: Reliability of machine and deep learning models on medical images can be compromised by the presence of image artifacts of variability in image acquisition. This is further exacerbated when validating model performance across multiple institutions, where multiple acquisition protocols and scanner equipment may result in significant numbers of outlier scans. We developed a privacy-preserving outlier identification algorithm via federated MR imaging quality evaluation of a distributed multi-institutional cohort of prostate MRI scans. Methods: This retrospective study utilized T2-weighted prostate MRI scans from 1 public collection (used as a template) and 3 federated institutions. An open-source MR image quality evaluation tool (MRQy) was implemented via Docker within the RhinoHealth Federated Computing Platform (FCP). This enabled remote extraction of 9 specific metadata values and remote computation of 15 quality measurements from each scan, while ensuring confidentiality of patient data by avoiding direct access to patient images and information. Centralized analysis of extracted image quality attributes utilized a rule-based classifier to determine whether individual metadata or measurement values for a given MRI scan fell within or outside predefined bounds: (i) ±20% of template metadata values, (ii) 5th - 95th percentile range of template measurement values. Scans were considered outliers if more than 60% of their image quality attributes fell outside predefined bounds. Results: In total, 630 MRI scans from 3 institutions were analyzed in a federated manner. 344 MRI scans from 1 public collection formed a benchmark cohort to calibrate the rule-based classifier and determine predefined bounds for outlier detection. Centralized analysis of federated image quality attributes showed marked differences in the number of outliers per institution (3%, 19%, 79% for Institutions 1, 2, 3, respectively). Further analysis of Institution 3 determined significant intensity and resolution differences compared to other institutions, requiring extensive post-processing prior to downstream analysis. Conclusion: Federated image quality assessment can allow for distributed, privacy preserving analysis of outliers and thus curate multi-institutional cohorts of consistent quality for downstream machine learning tasks. Further analysis of the impact of quality-based curation on federated machine learning applications is currently underway. Citation Format: Mohsen Hariri, Prathyush Chirra, Malhar Patel, Tal Tiano Einat, Ittai Dayan, Alex Tonetti, Yuval Baror, Tristan Barrett, Nikita Sushentsev, Joshua D. Kaggie, Shuaiyu Yuan, Dufan Wu, Baihui Yu, Zhiliang Lyu, Cheyu Hsu, Weichung Wang, Smitha Krishnamurthi, Satish E. Viswanath. Federated image quality assessment of prostate MRI scans in a multi-institutional setting [abstract]. In: Proceedings of the American Association for Cancer Research Annual Meeting 2024; Part 1 (Regular Abstracts); 2024 Apr 5-10; San Diego, CA. Philadelphia (PA): AACR; Cancer Res 2024;84(6_Suppl):Abstract nr 2344.
更多查看译文
AI 理解论文
溯源树
样例
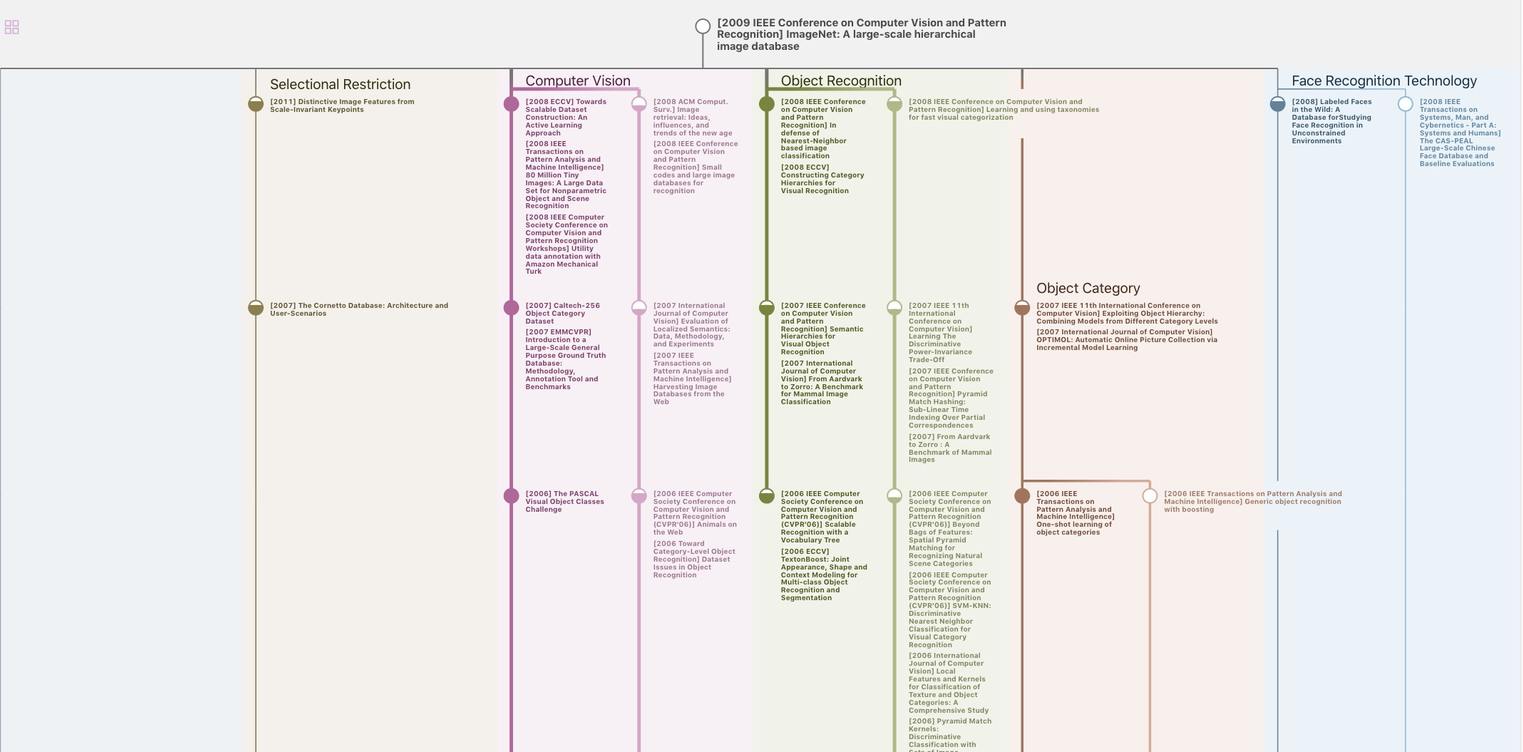
生成溯源树,研究论文发展脉络
Chat Paper
正在生成论文摘要