Abstract 3521: Robust inference of PTEN deletion in prostate cancer from routine histopathology whole slide images using attention-based deep learning
Cancer Research(2024)
摘要
Abstract The diagnostic workup of prostate cancer (PCa) requires a thorough manual histopathological inspection of prostate tissue specimens stained with hematoxylin and eosin (H&E). While immunohistochemistry and advanced molecular tests like FISH could uncover key genetic alterations, they are not cost-effective and often require special training, necessitating the development of more effective tools that can accurately infer the status of such alterations directly from the tissue morphology depicted in routine H&E-stained glass slides. PTEN deletion, which is present in 15-20% of PCa, is significantly associated with tumor aggressiveness and negative prognostic impact. Deep learning (DL) models can be trained to predict the presence of PTEN deletion on routine whole slide images (WSIs) and select patients that could benefit from further treatment. In this study, we trained and tested the performance of a weakly supervised, multiple-instance learning network on a cohort of 449 WSIs radical prostatectomy specimens stained with hematoxylin & eosin. Our model demonstrated robust performance in predicting PTEN loss based on tumor morphology alone. Through the model, we also generated attention heatmaps of the most informative tiles of each slide, allowing us to quantify the different cellular components, thus inferring the morphological features associated with PTEN loss. This study shows that DL is a potential tool that can assist the pathologist in accurately inferring the molecular status of key genetic alterations using the tissue morphology depicted in histopathology slides. Moreover, the morphological information that can be obtained by attention-based models can be leveraged to close the gap between the pathologist’s morphological analysis and the interpretability of artificial intelligence models. Citation Format: Federico Scarfò, Mohamed Omar, Luigi Marchionni. Robust inference of PTEN deletion in prostate cancer from routine histopathology whole slide images using attention-based deep learning [abstract]. In: Proceedings of the American Association for Cancer Research Annual Meeting 2024; Part 1 (Regular Abstracts); 2024 Apr 5-10; San Diego, CA. Philadelphia (PA): AACR; Cancer Res 2024;84(6_Suppl):Abstract nr 3521.
更多查看译文
AI 理解论文
溯源树
样例
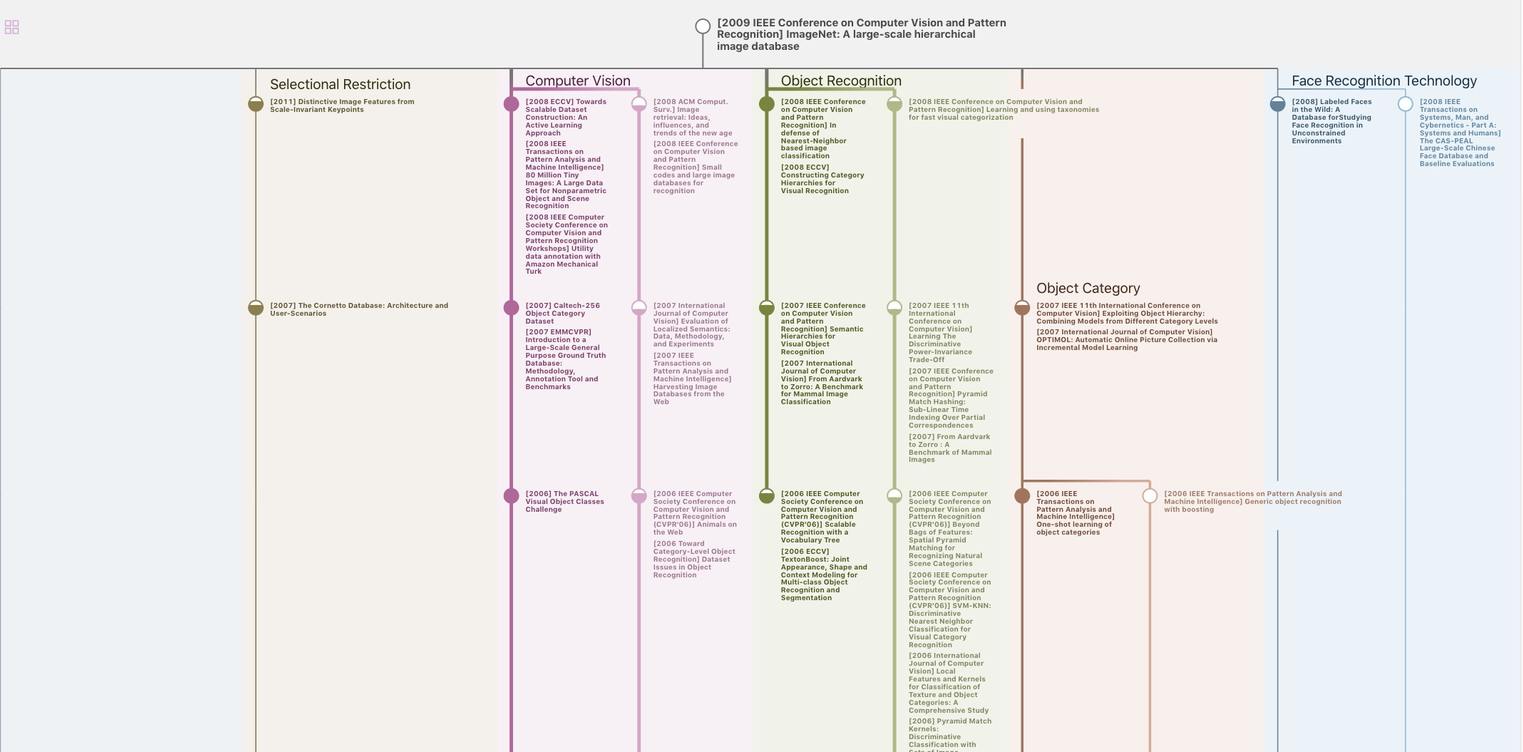
生成溯源树,研究论文发展脉络
Chat Paper
正在生成论文摘要